Self-Supervised Object-Level Deep Reinforcement Learning
arxiv(2020)
摘要
Current deep reinforcement learning approaches incorporate minimal prior knowledge about the environment, limiting computational and sample efficiency. We incorporate a few object-based priors that humans are known to use: "Infants divide perceptual arrays into units that move as connected wholes, that move separately from one another, that tend to maintain their size and shape over motion, and that tend to act upon each other only on contact" [Spelke]. We propose a probabilistic object-based model of environments and use human object priors to develop an efficient self-supervised algorithm for maximum likelihood estimation of the model parameters from observations and for inferring objects directly from the perceptual stream. We then use object features and incorporate object-contact priors to improve the sample efficiency our object-based RL agent.We evaluate our approach on a subset of the Atari benchmarks, and learn up to four orders of magnitude faster than the standard deep Q-learning network, rendering rapid desktop experiments in this domain feasible. To our knowledge, our system is the first to learn any Atari task in fewer environment interactions than humans.
更多查看译文
关键词
reinforcement learning,self-supervised,object-level
AI 理解论文
溯源树
样例
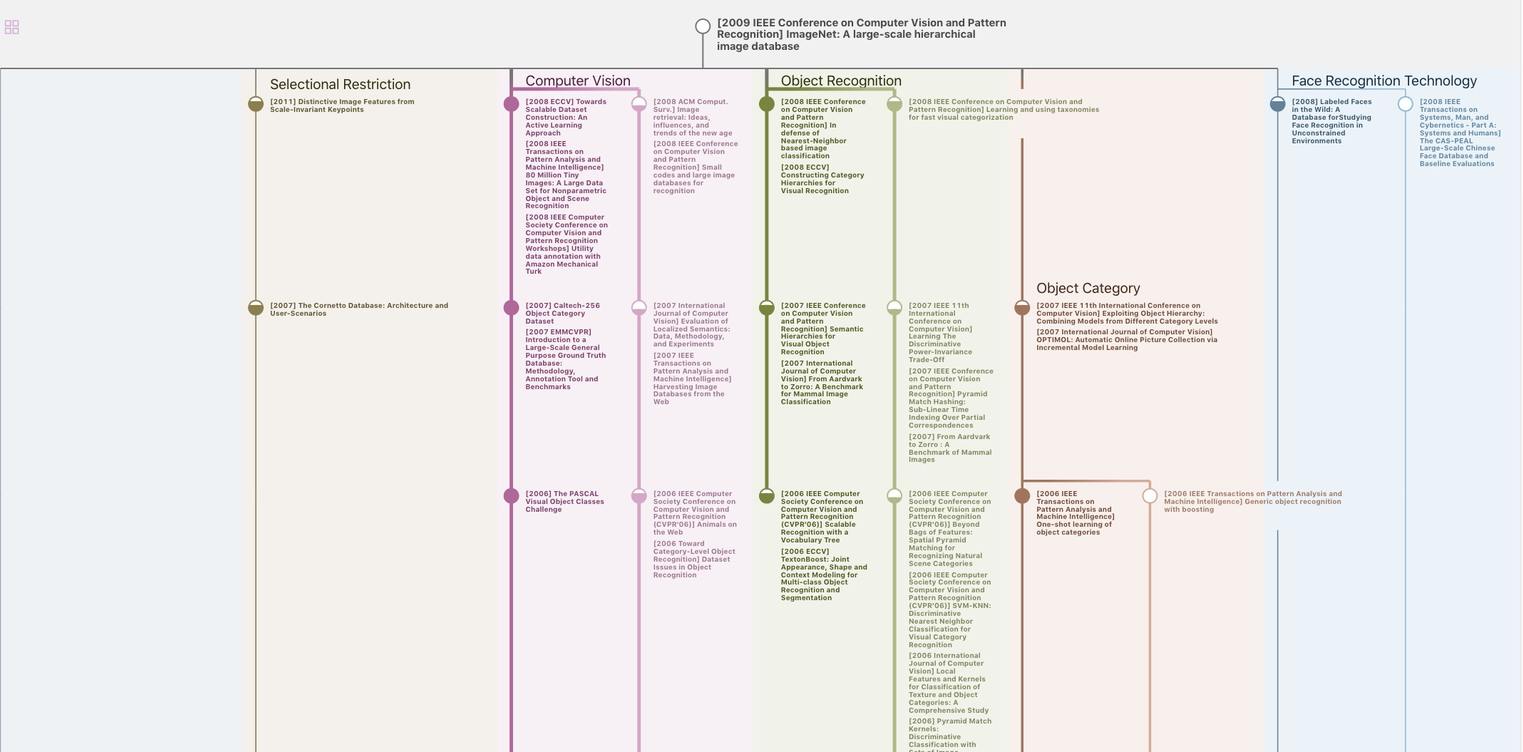
生成溯源树,研究论文发展脉络
Chat Paper
正在生成论文摘要