Unsupervised Deep Learning for Depth Estimation with Offset Pixels
Optics Express(2020)
摘要
Offset Pixel Aperture (OPA) camera has been recently proposed to estimate disparity of a scene with a single shot. Disparity is obtained in the image by offsetting the pixels by a fixed distance. Previously, correspondence matching schemes have been used for disparity estimation with OPA. To improve disparity estimation we use a data-oriented approach. Specifically, we use unsupervised deep learning to estimate the disparity in OPA images. We propose a simple modification to the training strategy which solves the vanishing gradients problem with the very small baseline of the OPA camera. Training degenerates to poor disparity maps if the OPA images are used directly for left-right consistency check. By using images obtained from displaced cameras at training, accurate disparity maps are obtained. The performance of the OPA camera is significantly improved compared to previously proposed single-shot cameras and unsupervised disparity estimation methods. The approach provides 8 frames per second on a single Nvidia 1080 GPU with 1024x512 OPA images. Unlike conventional approaches, which are evaluated in controlled environments, our paper shows the utility of deep learning for disparity estimation with real life sensors and low quality images. By combining OPA with deep learning, we obtain a small depth sensor capable of providing accurate disparity at usable frame rates. Also the ideas in this work can be used in small-baseline stereo systems for short-range depth estimation and multi-baseline stereo to increase the depth range. (C) 2020 Optical Society of America under the terms of the OSA Open Access Publishing Agreement
更多查看译文
AI 理解论文
溯源树
样例
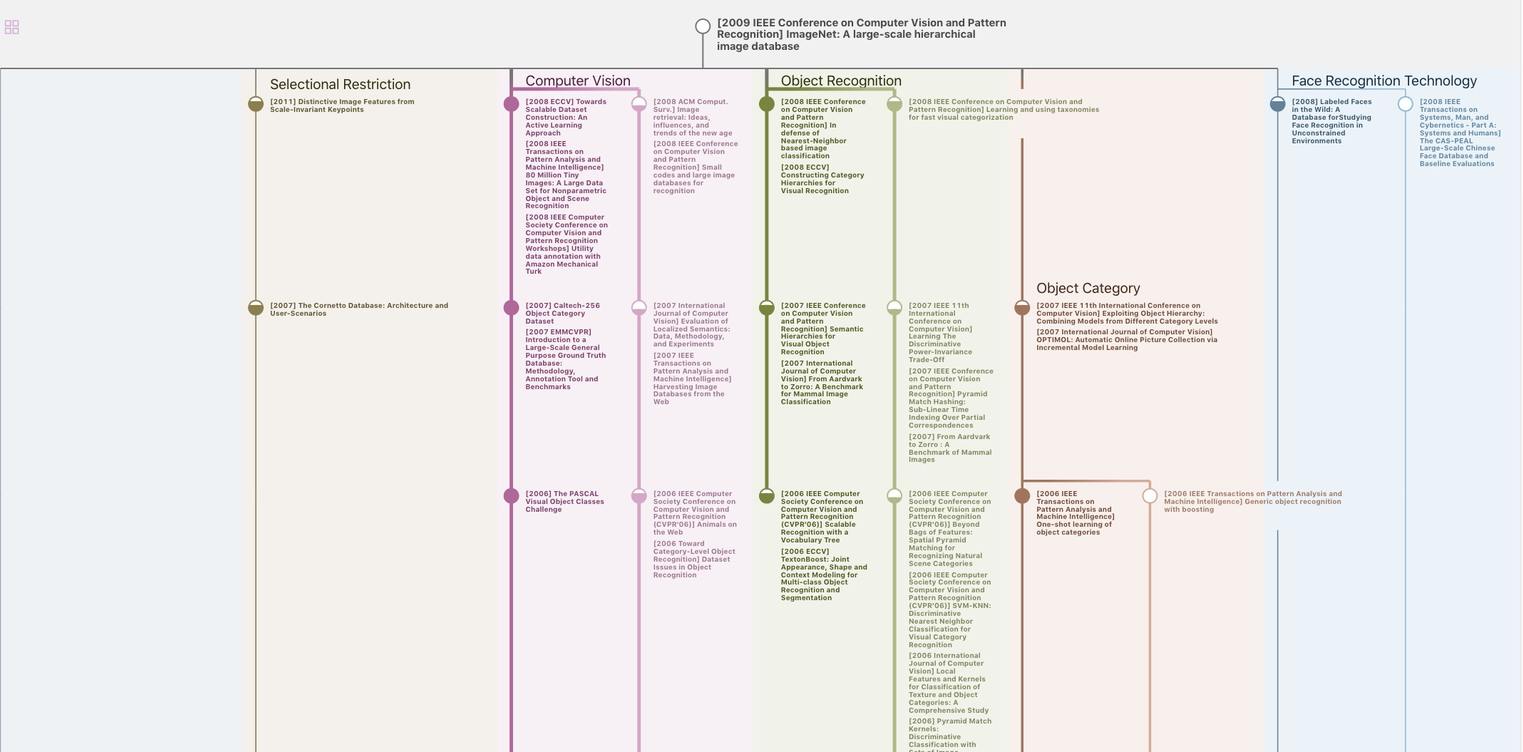
生成溯源树,研究论文发展脉络
Chat Paper
正在生成论文摘要