Enhancing deep discriminative feature maps via perturbation for face presentation attack detection
Image and Vision Computing(2020)
摘要
Face presentation attack detection (PAD) in unconstrained conditions is one of the key issues in face biometric-based authentication and security applications. In this paper, we propose a perturbation layer — a learnable pre-processing layer for low-level deep features — to enhance the discriminative ability of deep features in face PAD. The perturbation layer takes the deep features of a candidate layer in Convolutional Neural Network (CNN), the corresponding hand-crafted features of an input image, and produces adaptive convolutional weights for the deep features of the candidate layer. These adaptive convolutional weights determine the importance of the pixels in the deep features of the candidate layer for face PAD. The proposed perturbation layer adds very little overhead to the total trainable parameters in the model. We evaluated the proposed perturbation layer with Local Binary Patterns (LBP), with and without color information, on three publicly available face PAD databases, i.e., CASIA, Idiap Replay-Attack, and OULU-NPU databases. Our experimental results show that the introduction of the proposed perturbation layer in the CNN improved the face PAD performance, in both intra-database and cross-database scenarios. Our results also highlight the attention created by the proposed perturbation layer in the deep features and its effectiveness for face PAD in general.
更多查看译文
关键词
Spoofing,Presentation attack detection,CNN,Attention,Face-biometrics
AI 理解论文
溯源树
样例
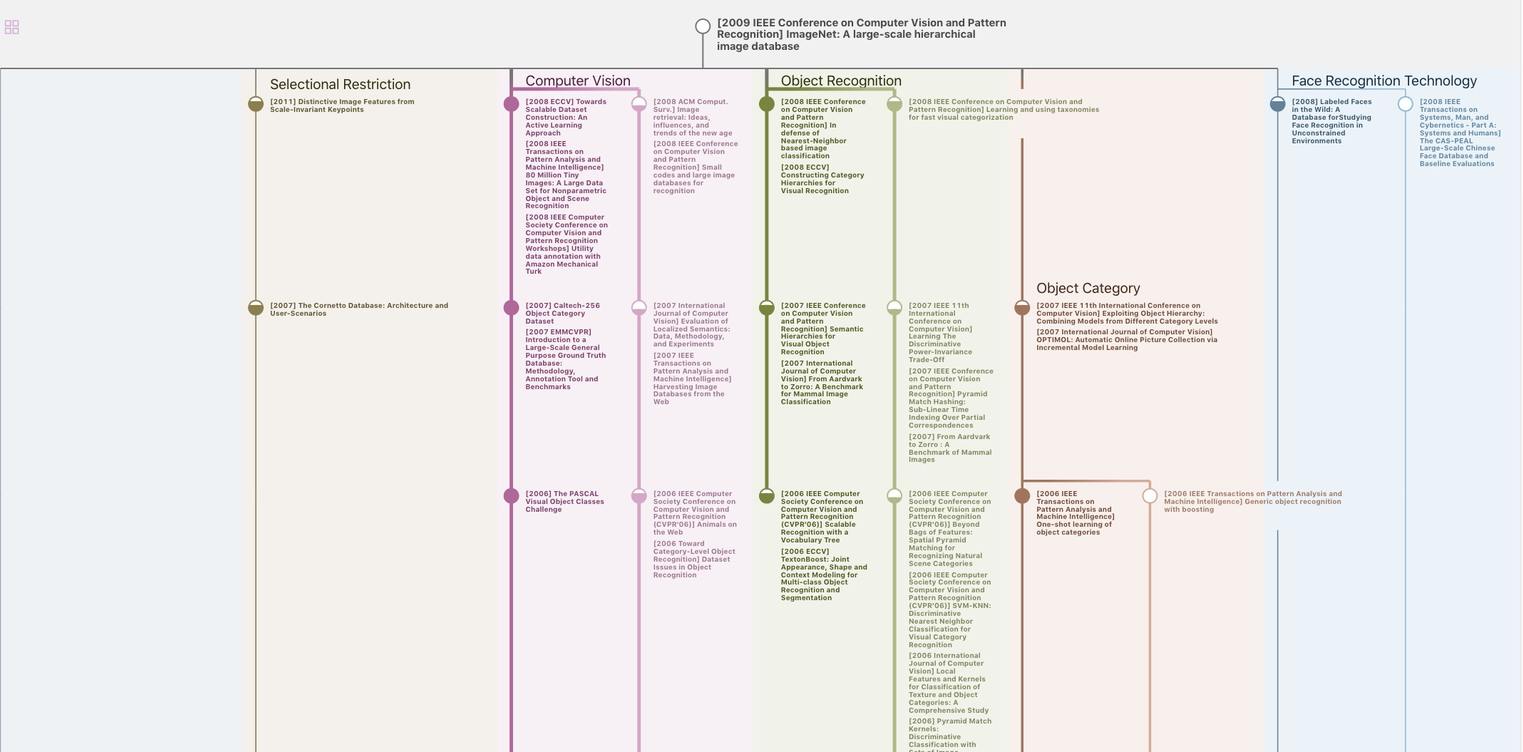
生成溯源树,研究论文发展脉络
Chat Paper
正在生成论文摘要