Understanding and Improving Knowledge Distillation
arxiv(2020)
摘要
Knowledge distillation is a model-agnostic technique to improve model quality while having a fixed capacity budget. It is a commonly used technique for model compression, where a higher capacity teacher model with better quality is used to train a more compact student model with better inference efficiency. Through distillation, one hopes to benefit from student's compactness, without sacrificing too much on model quality. Despite the large success of knowledge distillation, better understanding of how it benefits student model's training dynamics remains under-explored. In this paper, we dissect the effects of knowledge distillation into three main factors: (1) benefits inherited from label smoothing, (2) example re-weighting based on teacher's confidence on ground-truth, and (3) prior knowledge of optimal output (logit) layer geometry. Using extensive systematic analyses and empirical studies on synthetic and real-world datasets, we confirm that the aforementioned three factors play a major role in knowledge distillation. Furthermore, based on our findings, we propose a simple, yet effective technique to improve knowledge distillation empirically.
更多查看译文
关键词
knowledge,understanding
AI 理解论文
溯源树
样例
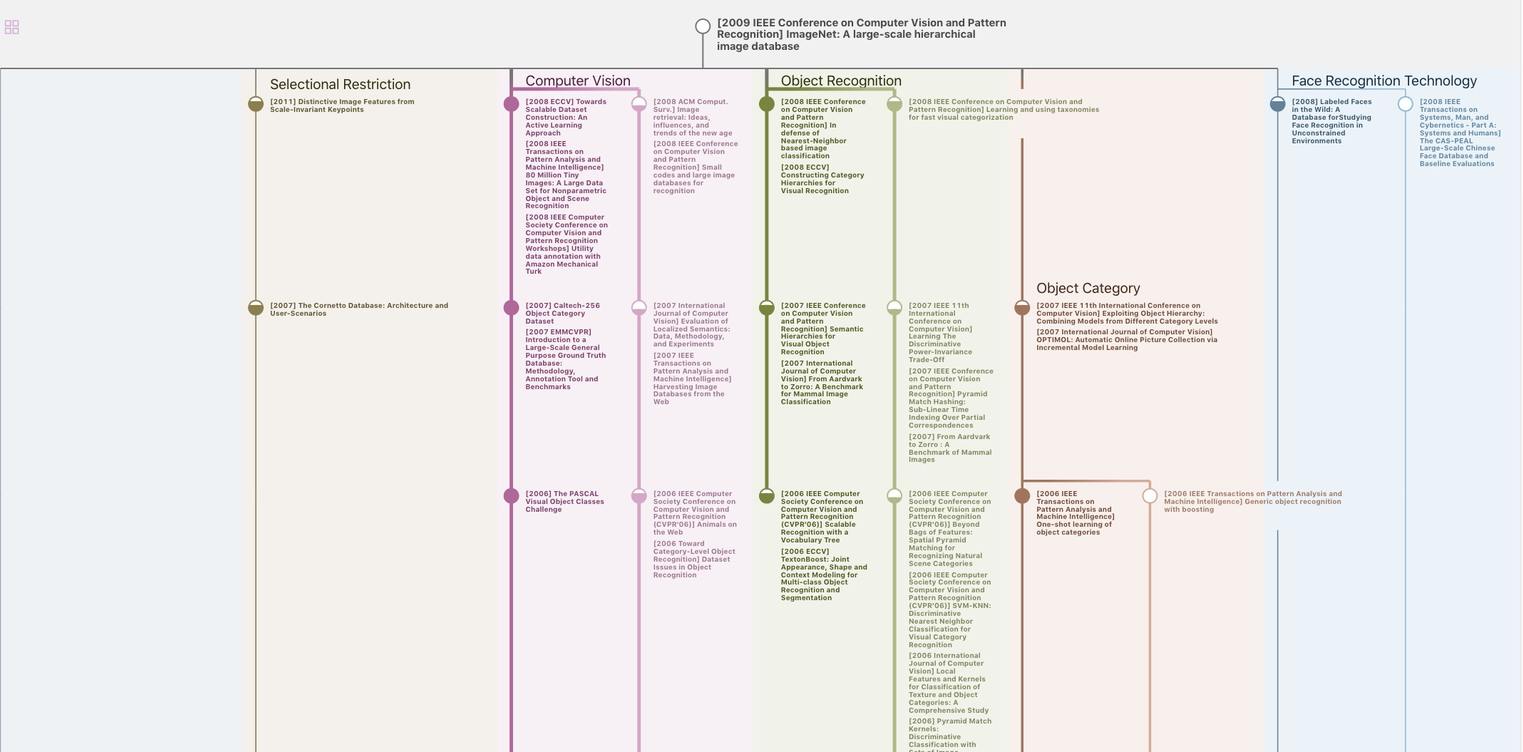
生成溯源树,研究论文发展脉络
Chat Paper
正在生成论文摘要