Adversarial Examples in RF Deep Learning: Detection and Physical Robustness
IEEE Global Conference on Signal and Information Processing(2019)
摘要
While research on adversarial examples (AdExs) in machine learning for images has been prolific, similar attacks on deep learning (DL) for radio frequency (RF) signals and corresponding mitigation strategies are scarcely addressed in the published work, with only a handful of recent publications in the RF domain. With minimal waveform perturbation, RF adversarial examples (AdExs) can cause a substantial increase in misclassifications for spectrum sensing/survey applications (e.g. ZigBee mistaken for Bluetooth). In this work, two statistical tests for AdEx detection are proposed. One statistical test leverages the peak-to-average-power ratio (PAPR) of the RF samples. The second test uses the softmax outputs of the machine learning model, which is proportional to the likelihoods the classifier assigns to each of the trained classes. The first test leverages the RF nature of the data while the latter is universally applicable to AdExs regardless of the domain. Both solutions are shown as viable mitigation methods to subvert adversarial attacks against RF waveforms, and their effectiveness is analyzed as function of the propagation channel and type of waveform.
更多查看译文
关键词
RF deep learning,physical robustness,radio frequency signals,RF adversarial examples,statistical tests,AdEx detection,peak-to-average-power ratio,RF samples,machine learning model,viable mitigation methods,adversarial attacks,RF waveforms,waveform perturbation,spectrum sensing
AI 理解论文
溯源树
样例
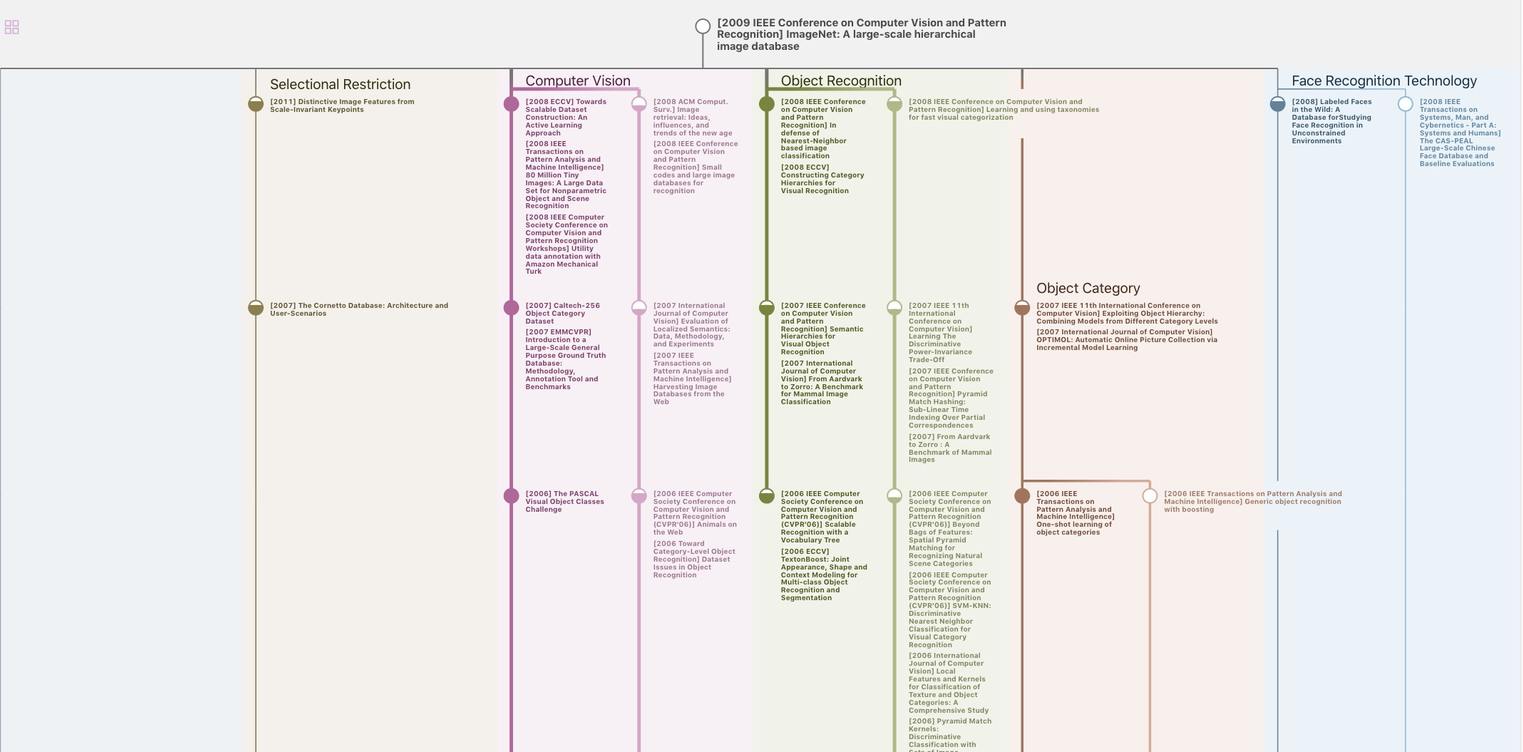
生成溯源树,研究论文发展脉络
Chat Paper
正在生成论文摘要