Panopticfusion: Online Volumetric Semantic Mapping At The Level Of Stuff And Things
2019 IEEE/RSJ INTERNATIONAL CONFERENCE ON INTELLIGENT ROBOTS AND SYSTEMS (IROS)(2019)
摘要
We propose PanopticFusion, a novel online volumetric semantic mapping system at the level of stuff and things. In contrast to previous semantic mapping systems, PanopticFusion is able to densely predict class labels of a background region (stuff) and individually segment arbitrary foreground objects (things). In addition, our system has the capability to reconstruct a large-scale scene and extract a labeled mesh thanks to its use of a spatially hashed volumetric map representation. Our system first predicts pixel-wise panoptic labels (class labels for stuff regions and instance IDs for thing regions) for incoming RGB frames by fusing 2D semantic and instance segmentation outputs. The predicted panoptic labels are integrated into the volumetric map together with depth measurements while keeping the consistency of the instance IDs, which could vary frame to frame, by referring to the 3D map at that moment. In addition, we construct a fully connected conditional random field (CRF) model with respect to panoptic labels for map regularization. For online CRF inference, we propose a novel unary potential approximation and a map division strategy.We evaluated the performance of our system on the ScanNet (v2) dataset. PanopticFusion outperformed or compared with state-of-the-art offline 3D DNN methods in both semantic and instance segmentation benchmarks. Also, we demonstrate a promising augmented reality application using a 3D panoptic map generated by the proposed system.
更多查看译文
关键词
spatially hashed volumetric map representation,pixel-wise panoptic labels,class labels,instance IDs,incoming RGB frames,fully connected conditional random field model,map regularization,online CRF inference,map division strategy,PanopticFusion,semantic instance segmentation benchmarks,3D panoptic map,online volumetric semantic mapping,semantic mapping system,background region,large-scale scene,labeled mesh,arbitrary foreground object segmentation,ScanNet dataset
AI 理解论文
溯源树
样例
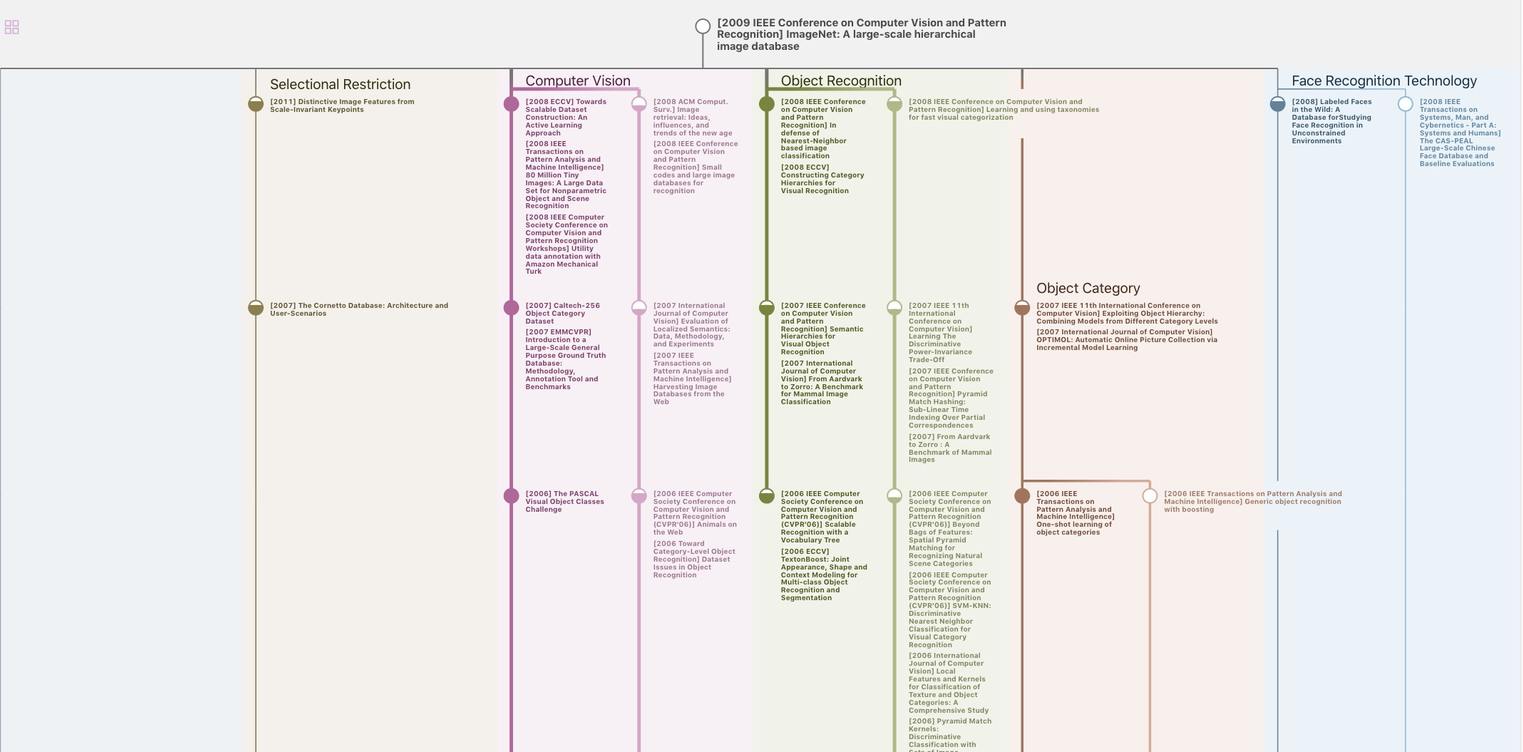
生成溯源树,研究论文发展脉络
Chat Paper
正在生成论文摘要