Neighborhood Structure Assisted Non-Negative Matrix Factorization And Its Application In Unsupervised Point-Wise Anomaly Detection
JOURNAL OF MACHINE LEARNING RESEARCH(2021)
摘要
Dimensionality reduction is considered as an important step for ensuring competitive performance in unsupervised learning such as anomaly detection. Non-negative matrix factorization (NMF) is a widely used method to accomplish this goal. But NMF do not have the provision to include the neighborhood structure information and, as a result, may fail to provide satisfactory performance in presence of nonlinear manifold structure. To address this shortcoming, we propose to consider the neighborhood structural similarity information within the NMF framework and do so by modeling the data through a minimum spanning tree. We label the resulting method as the neighborhood structure-assisted NMF. We further develop both offline and online algorithms for implementing the proposed method. Empirical comparisons using twenty benchmark data sets as well as an industrial data set extracted from a hydropower plant demonstrate the superiority of the neighborhood structure-assisted NMF. Looking closer into the formulation and properties of the proposed NMF method and comparing it with several NMF variants reveal that inclusion of the MST-based neighborhood structure plays a key role in attaining the enhanced performance in anomaly detection.
更多查看译文
关键词
minimum spanning tree, non-negative matrix factorization, unsupervised anomaly detection
AI 理解论文
溯源树
样例
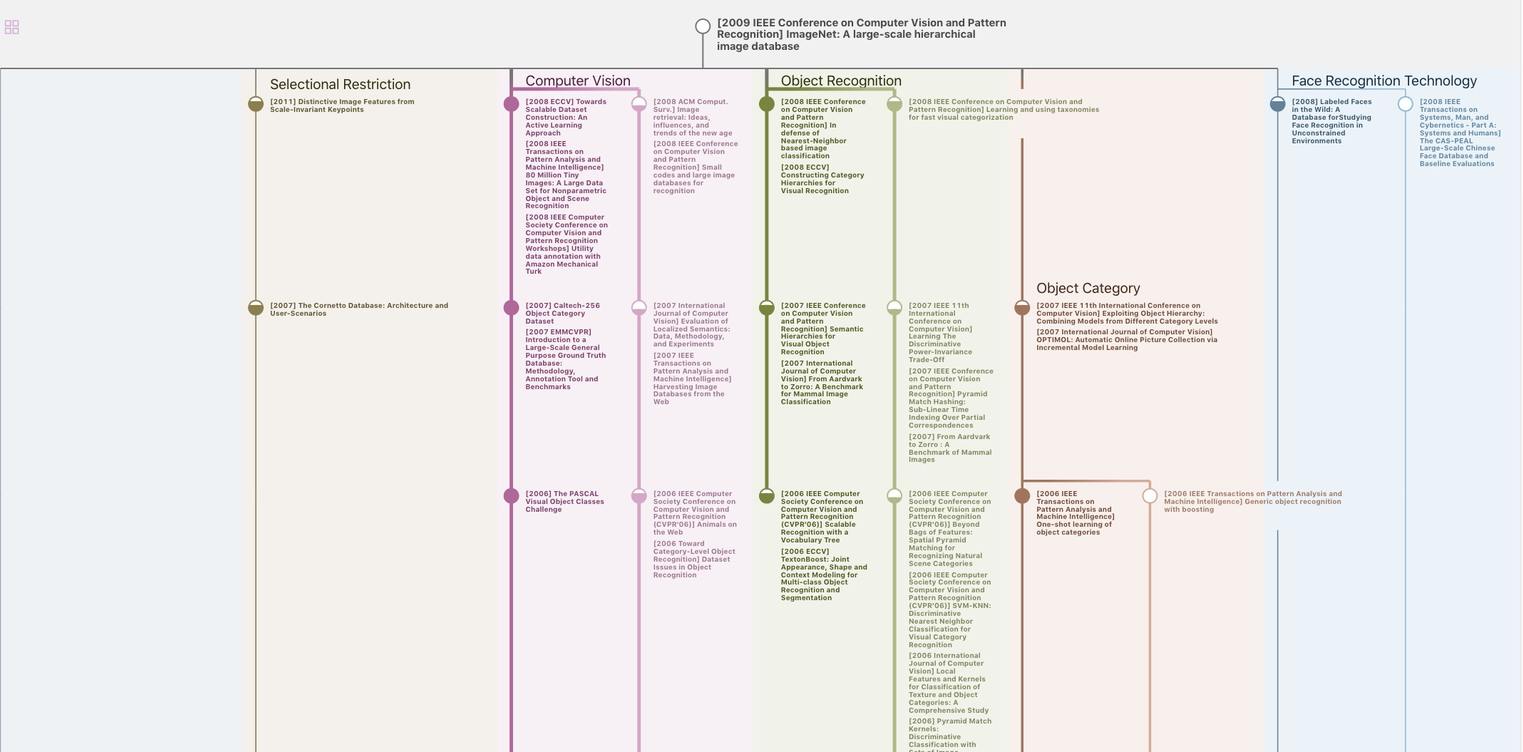
生成溯源树,研究论文发展脉络
Chat Paper
正在生成论文摘要