Infinite-Horizon Differentiable Model Predictive Control
arXiv (Cornell University)(2020)
摘要
This paper proposes a differentiable linear quadratic Model Predictive Control (MPC) framework for safe imitation learning. The infinite-horizon cost is enforced using a terminal cost function obtained from the discrete-time algebraic Riccati equation (DARE), so that the learned controller can be proven to be stabilizing in closed-loop. A central contribution is the derivation of the analytical derivative of the solution of the DARE, thereby allowing the use of differentiation-based learning methods. A further contribution is the structure of the MPC optimization problem: an augmented Lagrangian method ensures that the MPC optimization is feasible throughout training whilst enforcing hard constraints on state and input, and a pre-stabilizing controller ensures that the MPC solution and derivatives are accurate at each iteration. The learning capabilities of the framework are demonstrated in a set of numerical studies.
更多查看译文
关键词
Model Predictive Control, Riccati Equation, Imitation Learning, Safe Learning
AI 理解论文
溯源树
样例
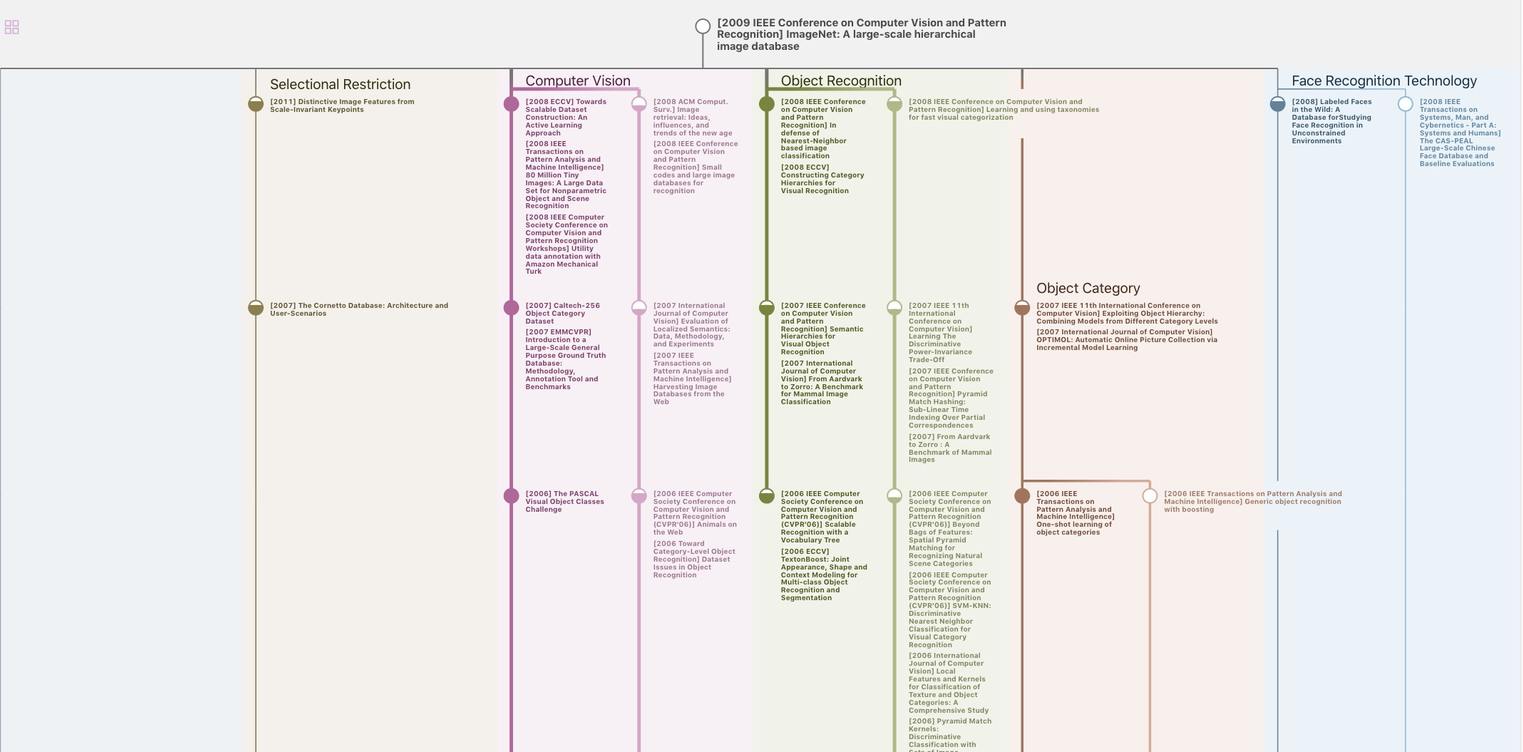
生成溯源树,研究论文发展脉络
Chat Paper
正在生成论文摘要