Domain-Independent Dominance of Adaptive Methods
2021 IEEE/CVF CONFERENCE ON COMPUTER VISION AND PATTERN RECOGNITION, CVPR 2021(2021)
摘要
From a simplified analysis of adaptive methods, we derive AvaGrad, a new optimizer which outperforms SGD on vision tasks when its adaptability is properly tuned. We observe that the power of our method is partially explained by a decoupling of learning rate and adaptability, greatly simplifying hyperparameter search. In light of this observation, we demonstrate that, against conventional wisdom, Adam can also outperform SGD on vision tasks, as long as the coupling between its learning rate and adaptability is taken into account. In practice, AvaGrad matches the best results, as measured by generalization accuracy, delivered by any existing optimizer (SGD or adaptive) across image classification (CIFAR, ImageNet) and character-level language modelling (Penn Treebank) tasks. When training GANs, AvaGrad improves upon existing optimizers.
更多查看译文
关键词
domain-independent dominance,adaptive methods,simplified analysis,AvaGrad,SGD,vision tasks,adaptability,learning rate,greatly simplifying hyperparameter search,existing optimizer,image classification,CIFAR,ImageNet,character-level language modelling tasks,Penn Treebank
AI 理解论文
溯源树
样例
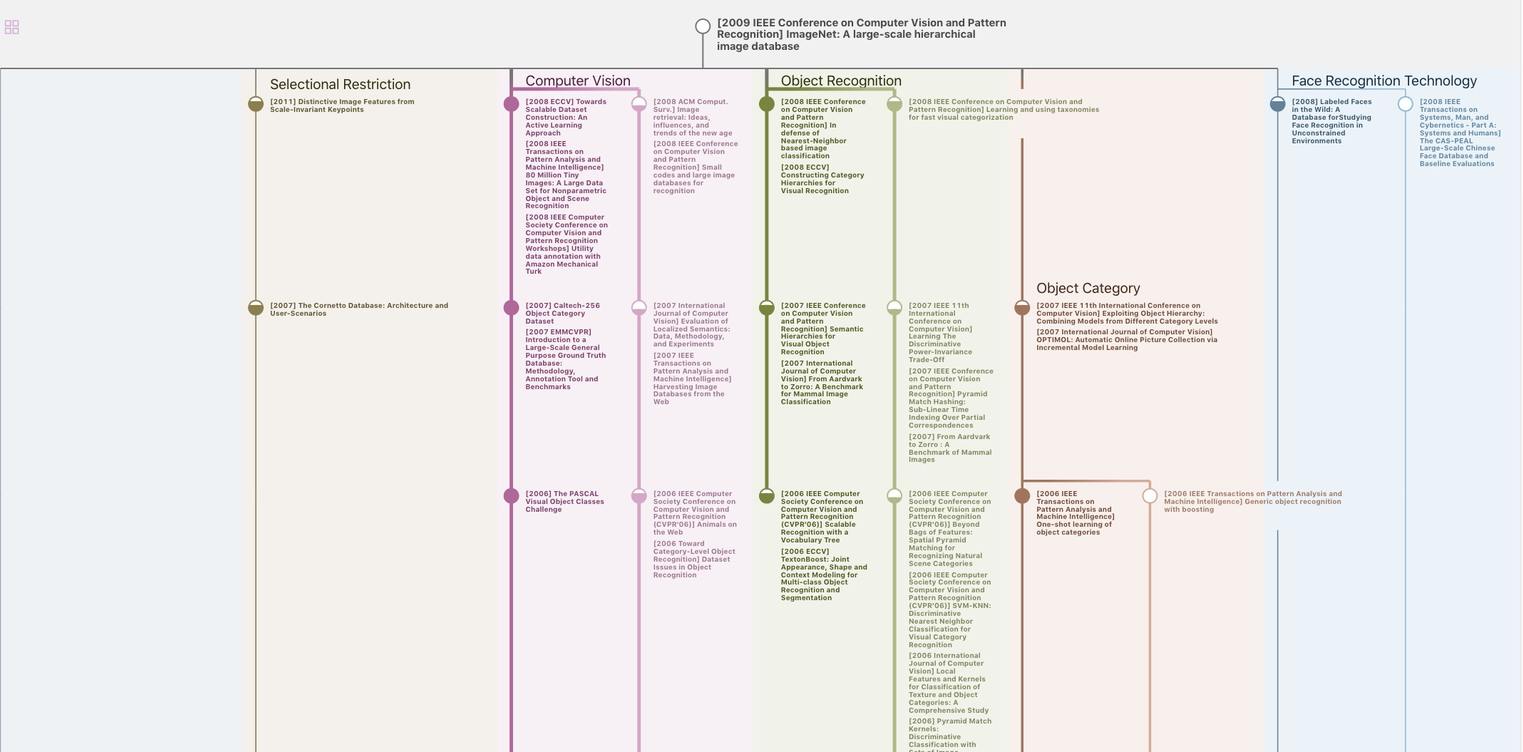
生成溯源树,研究论文发展脉络
Chat Paper
正在生成论文摘要