Absinthe: Learning an Analytical Performance Model to Fuse and Tile Stencil Codes in One Shot
2019 28th International Conference on Parallel Architectures and Compilation Techniques (PACT)(2019)
摘要
Expensive data movement makes the optimal target-specific selection of data-locality transformations essential. Loop fusion and tiling are the most important data-locality transformations. Their optimal selection is hard since good tile size choices inherently depend on the fusion choices and vice versa. Existing approaches avoid this difficulty by optimizing independent analytical models or by reverting to heuristics. Absinthe formulates the first unified linear optimization problem to derive single shot fusion and tile size decisions for stencil codes. At the core of our optimization problem, we place a learned analytic performance model that captures the characteristics of the target system. The tuned application kernels demonstrate excellent performance within 10% of exhaustively auto-tuned versions and up to 74% faster than the results of independent optimization with max fusion heuristic and Absinthe tile size selection. While the full search space is non-linear, bounding it to relevant solutions enables the efficient exploration of the exponential search space using linear solvers. As a result, the tuning of our application kernels takes less than one minute. Our approach thus establishes the foundations for next-generation compilers, which exploit empirical information to guide target-specific code transformations.
更多查看译文
关键词
stencils, performance model, data-locality
AI 理解论文
溯源树
样例
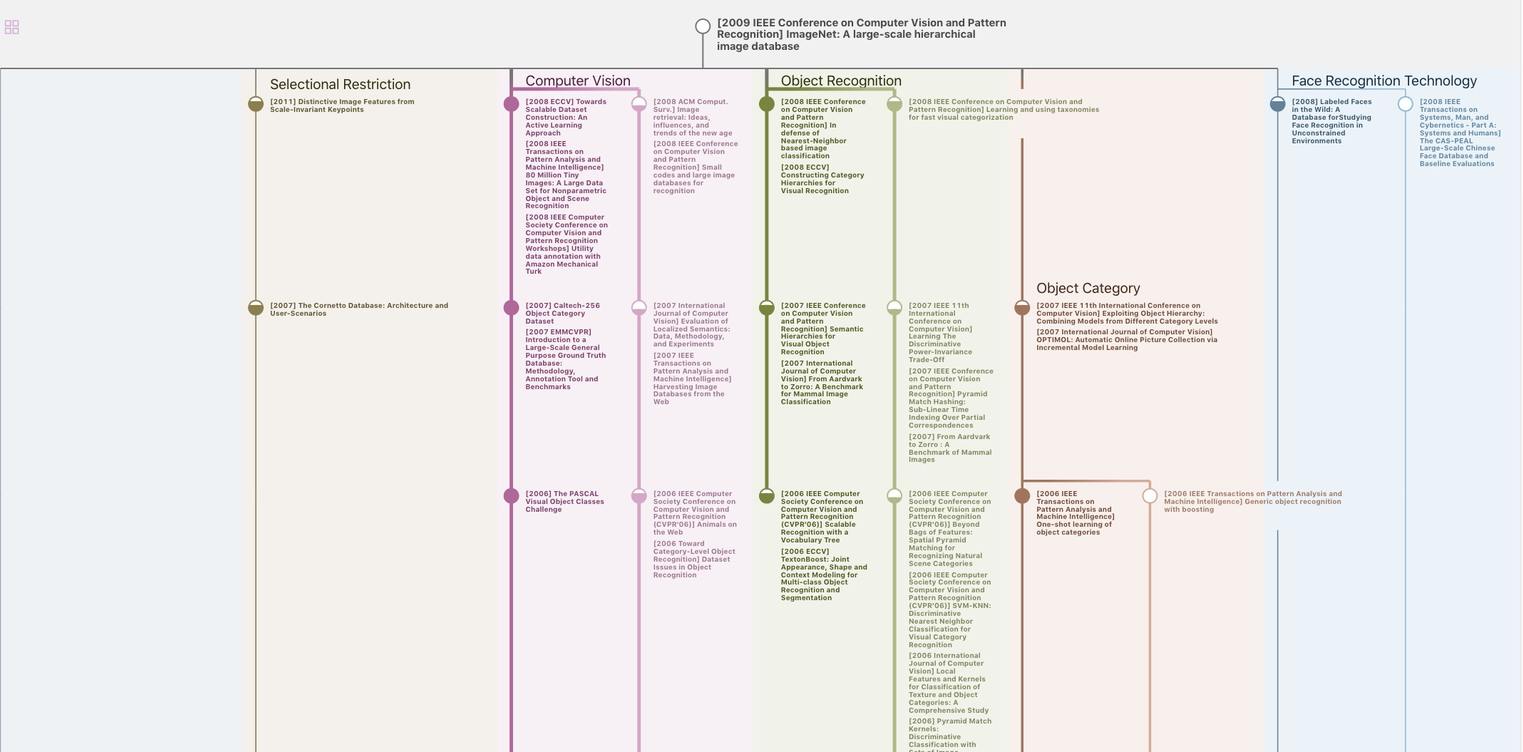
生成溯源树,研究论文发展脉络
Chat Paper
正在生成论文摘要