Big Data For The Stratification Of Readmission Risk After Hospital Discharge Of Older Adults With Complex Conditions
INTERNATIONAL JOURNAL OF INTEGRATED CARE(2019)
摘要
Introduction and objective: The increase in life expectancy along with scientific advances have led to an increasing number of elderly patients with several chronic conditions, requiring frequent hospital admissions due to exacerbation of their chronic diseases. Older patients that need to be readmitted within 30 days have a higher risk of negative health outcomes, thus it is important to identify those people at risk of readmission to be able to apply specific procedures. Our objective is to develop a method to stratify the risk of readmission in an older, frailty and high comorbid population. Methods: We collected demographic, clinical, functional and social data from consecutive patients admitted into the Subacute Care Unit (SCU) of an intermediate care hospital from January 2015 to April 2016. The 30 days readmission for each case was assessed according to shared electronical medical records. This dataset was used to train different Machine Learning models, including Logistic regression, Support Vector Machines, Decision Trees, and Random Forests, to predict whether a patient will require readmission from the information available at discharge time. A second dataset contained information of acute hospitalization episodes for these patients. Variables derived from this dataset were added to the previous one, and models retrained, to investigate whether this additional information allowed for better prediction. More precisely, three variables were added: number of hospitalizations in the past, total number of days in acute hospitals, and number of distinct ICD9 diagnostics recorded in those hospitalizations. Predictor error in all experiments was assessed using cross-validation. A toy economic model was built on top of the predictor to assess its usefulness. It can be tuned by parameters such as cost of a readmission, cost of a preventive action on a high-risk patient, risk threshold, and success probability of the action. Many others could be added in the future. Results: The Random Forest classifier gave best results among those tested. The C-statistic (or Area-under-the-curve measure) was 0.63. This is consistent with results in the literature for readmission prediction problems with data of similar detail. When the additional information was added, the measure raised up to around 0.8. In both cases, with realistic figures for the parameters of the economic model, the predictors appeared to be accurate enough to support cost-effective action plans. Conclusions: The data available at the SCU contains enough information to predict non-trivially 30-day readmission, well enough to support focused preventive measures on high-risk patients. Given that we work with highly vulnerable patients, which in most programs would be at risk of readmission, being able to increase the accuracy in assessing this risk is a major breakthrough. Since predictive power is largely increased by adding information on past acute hospitalizations of the patient, we encourage to move forward in the integration of different sources of information.
更多查看译文
关键词
readmission, older adults, predictive models, big data, machine learning, fragility
AI 理解论文
溯源树
样例
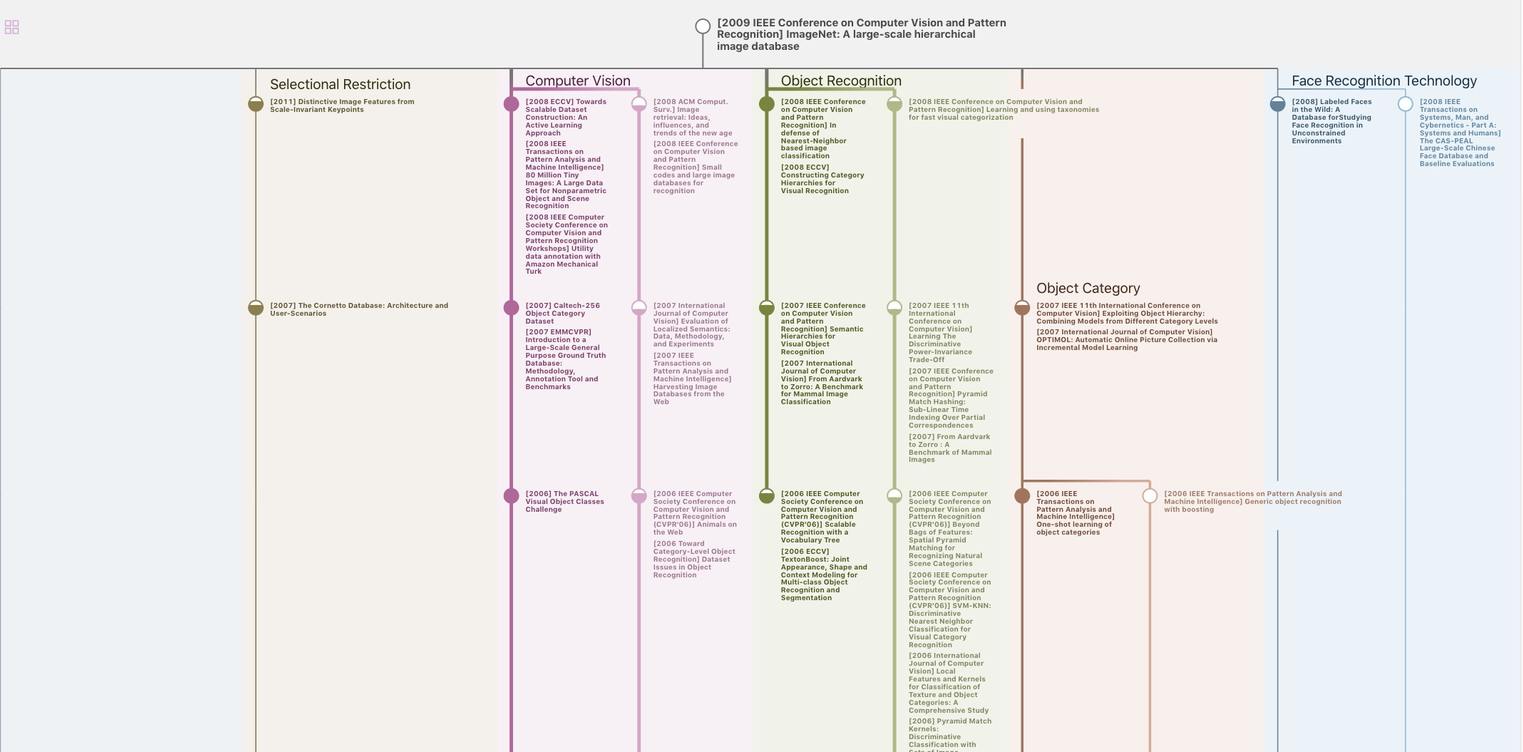
生成溯源树,研究论文发展脉络
Chat Paper
正在生成论文摘要