Conditional Learning of Fair Representations
CoRR(2019)
摘要
We propose a novel algorithm for learning fair representations that can simultaneously mitigate two notions of disparity among different demographic subgroups. Two key components underpinning the design of our algorithm are balanced error rate and conditional alignment of representations. We show how these two components contribute to ensuring accuracy parity and equalized false-positive and false-negative rates across groups without impacting demographic parity. Furthermore, we also demonstrate both in theory and on two real-world experiments that the proposed algorithm leads to a better utility-fairness trade-off on balanced datasets compared with existing algorithms on learning fair representations.
更多查看译文
关键词
algorithmic fairness, representation learning
AI 理解论文
溯源树
样例
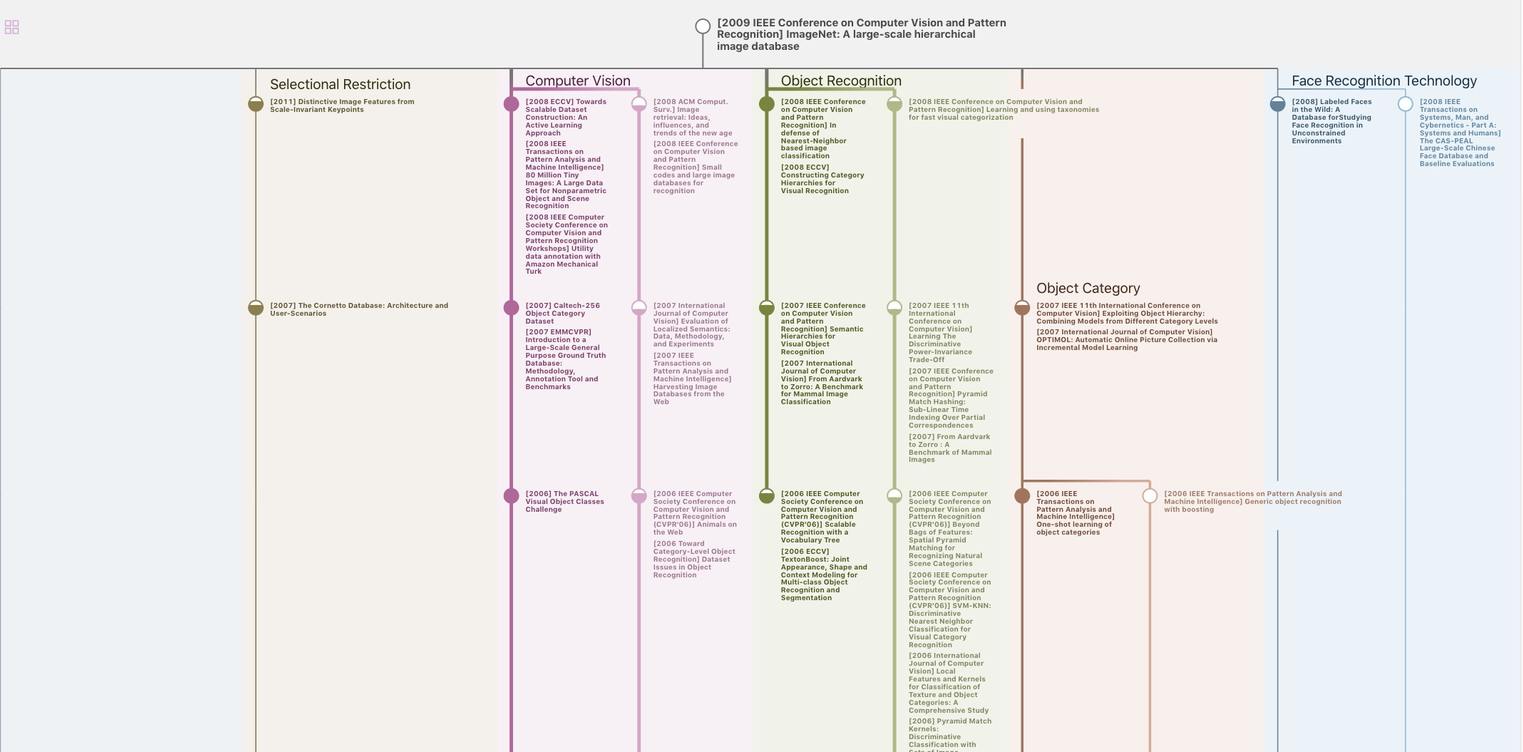
生成溯源树,研究论文发展脉络
Chat Paper
正在生成论文摘要