Fuzzy Deep Stack of Autoencoders for Dealing with Data Uncertainty
2019 IEEE International Conference on Fuzzy Systems (FUZZ-IEEE)(2019)
摘要
This paper addresses the problem of dealing with uncertainty on neural networks for the specific case of Autoencoders. The recently introduced concepts of `Autoencoders' and `Deep Stacks of Autoencoders' (DSAE) and their use for dimensionality reduction and data compression problems have gained considerable attention and reached very promising results. However, similarly to the traditional neural networks, Autoencoders are deterministic structures that are not very suitable for dealing with data uncertainty, a very important aspect of the real-world applications. In this paper, we propose a fuzzy approach to reduce uncertainty on stacks of Autoencoders by automatically adding qualitative fuzzy information about the data to the input layer. This can be accomplished by adding a fuzzy layer-0 to the stack of Autoencoders that extracts fuzzy knowledge from the crisp data set and includes that knowledge as extra information to the network input. The approach is completely transparent to the network and to the user and, theoretically, can be generalized to any architecture of neural network, including convolutional neural networks. The results presented here are very encouraging and present substantial improvement, especially when dealing with noisy data.
更多查看译文
关键词
data uncertainty,neural network,traditional neural networks,qualitative fuzzy information,fuzzy layer-0,extracts fuzzy knowledge,convolutional neural networks,fuzzy deep stack,deep stacks of autoencoders,DSAE
AI 理解论文
溯源树
样例
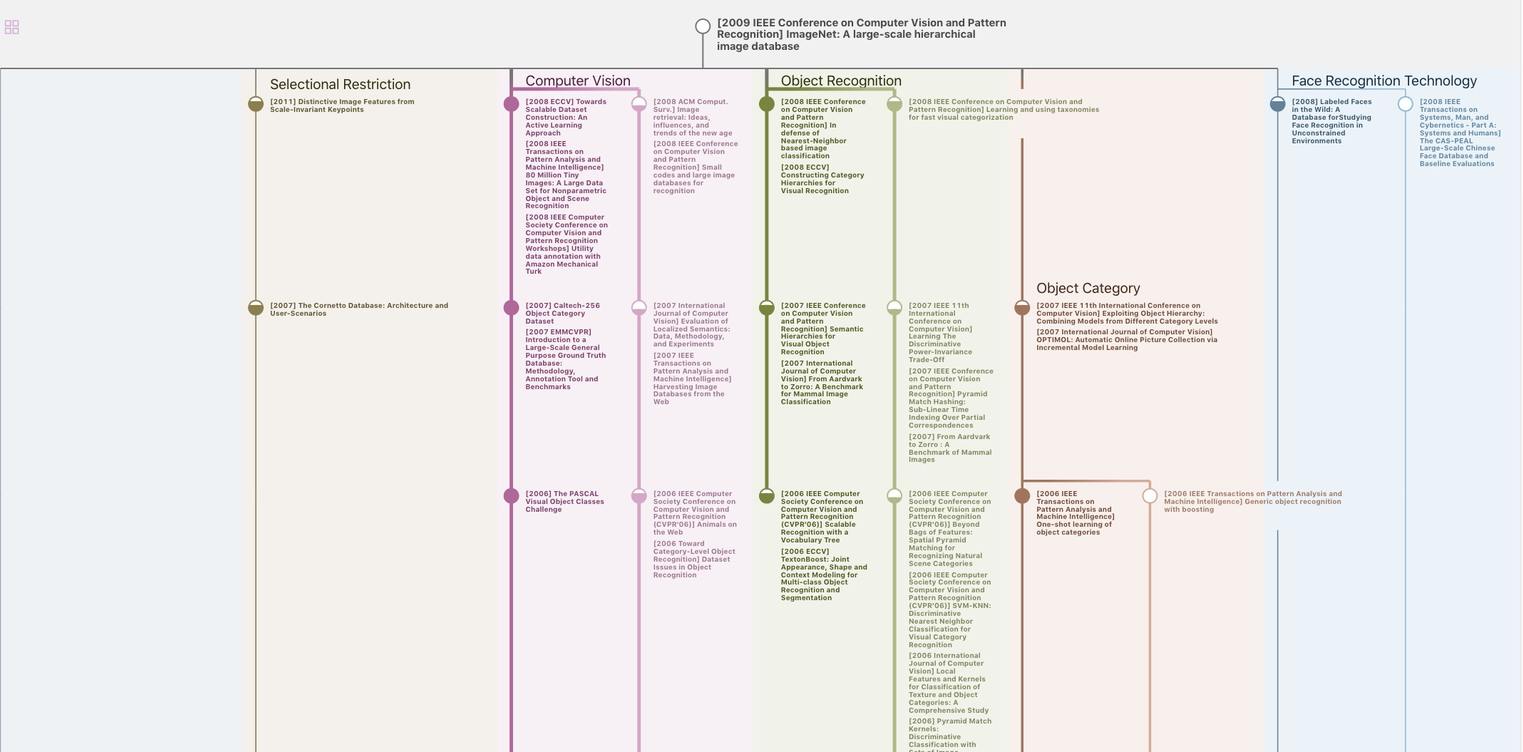
生成溯源树,研究论文发展脉络
Chat Paper
正在生成论文摘要