Learning high-order structural and attribute information by knowledge graph attention networks for enhancing knowledge graph embedding
Knowledge-Based Systems(2022)
摘要
The goal of representation learning of knowledge graph is to encode both entities and relations into a low-dimensional embedding space. Many recent works have demonstrated the benefits of knowledge graph embedding on knowledge graph completion task, such as relation extraction. However, we observe that: (1) existing methods simply take direct relations between entities into consideration and fails to express high-order structural relationships between entities; (2) these methods simply leverage relation triples of Knowledge Graphs while ignoring a large number of attribute triples that encode rich semantic information. To overcome these limitations, this paper proposes a novel knowledge graph embedding method, named (KANE), which is inspired by the recent developments in graph convolutional networks (GCN). KANE can capture both high-order structural and attribute information of Knowledge Graphs in an efficient, explicit and unified manner under the graph convolutional networks framework. Empirical results on three datasets show that KANE significantly outperforms seven state-of-the-art methods. Further analysis verify the efficiency of our method and the benefits brought by the attention mechanism.
更多查看译文
关键词
Knowledge graph,Representation learning,Knowledge graph embedding
AI 理解论文
溯源树
样例
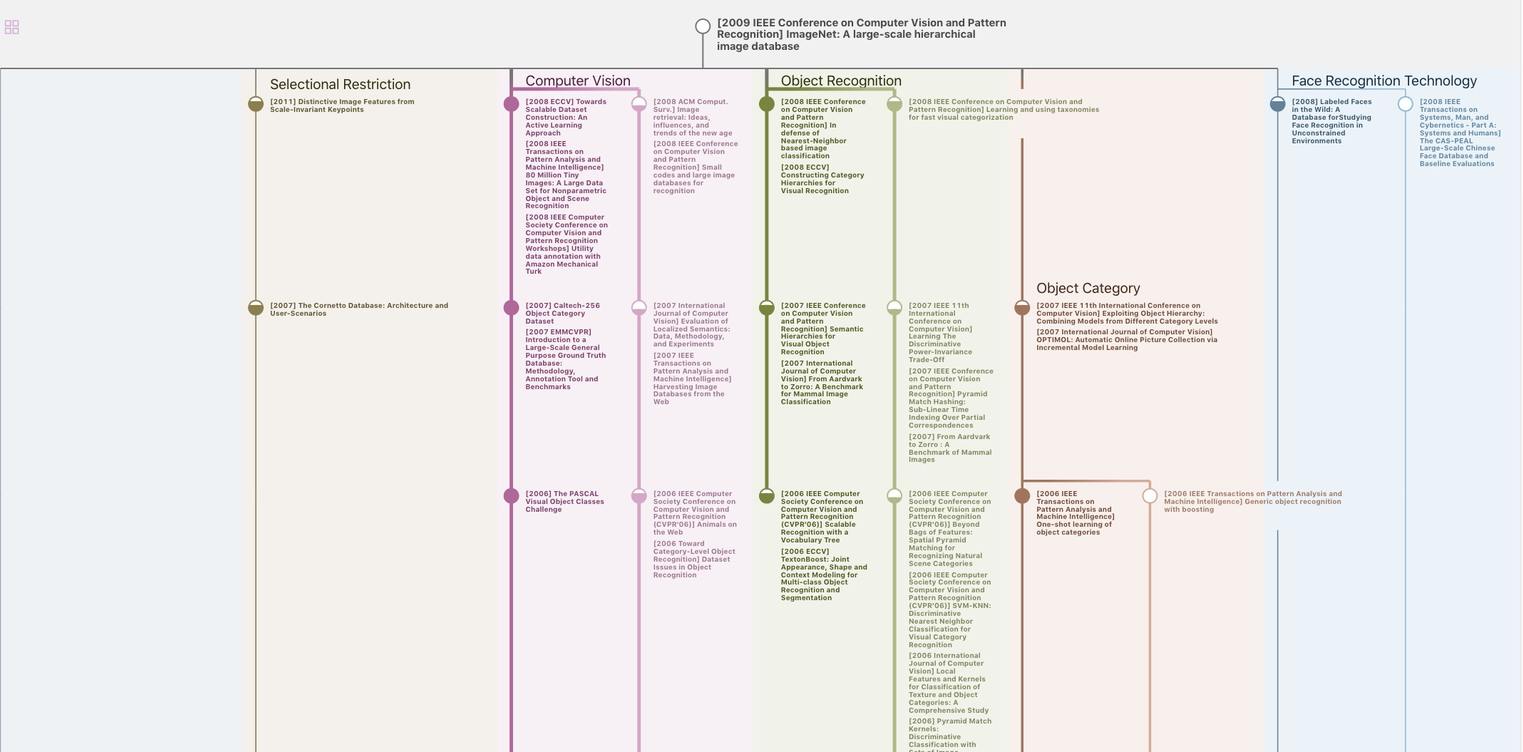
生成溯源树,研究论文发展脉络
Chat Paper
正在生成论文摘要