An Attention-Based Model for Learning Dynamic Interaction Networks
2019 International Joint Conference on Neural Networks (IJCNN)(2019)
摘要
In the physical world, complex systems are generally created as the composition of multiple primitive components that interact with each other rather than a single monolithic structure. Recently, spatio-temporal graphs received a reasonable amount of attention from the research community since they emerged as a natural representational tool able to capture the interactive and interrelated structure of a complex problem. To better understand the nature of complex systems, there is the need to define models that can easily explain the learned causal relationship. To this end, we propose an attentive model able to learn and project the relational structure into a fixed-size embedding. Such representation naturally captures the dynamic influence that each neighbors exert over a given vertex providing a valuable description of the problem setting. The proposed architecture has been extensively evaluated against strong baselines on toy as well as real-world tasks, such as prediction of household energy load and traffic congestion.
更多查看译文
关键词
Interactive Graphs,Attention Network
AI 理解论文
溯源树
样例
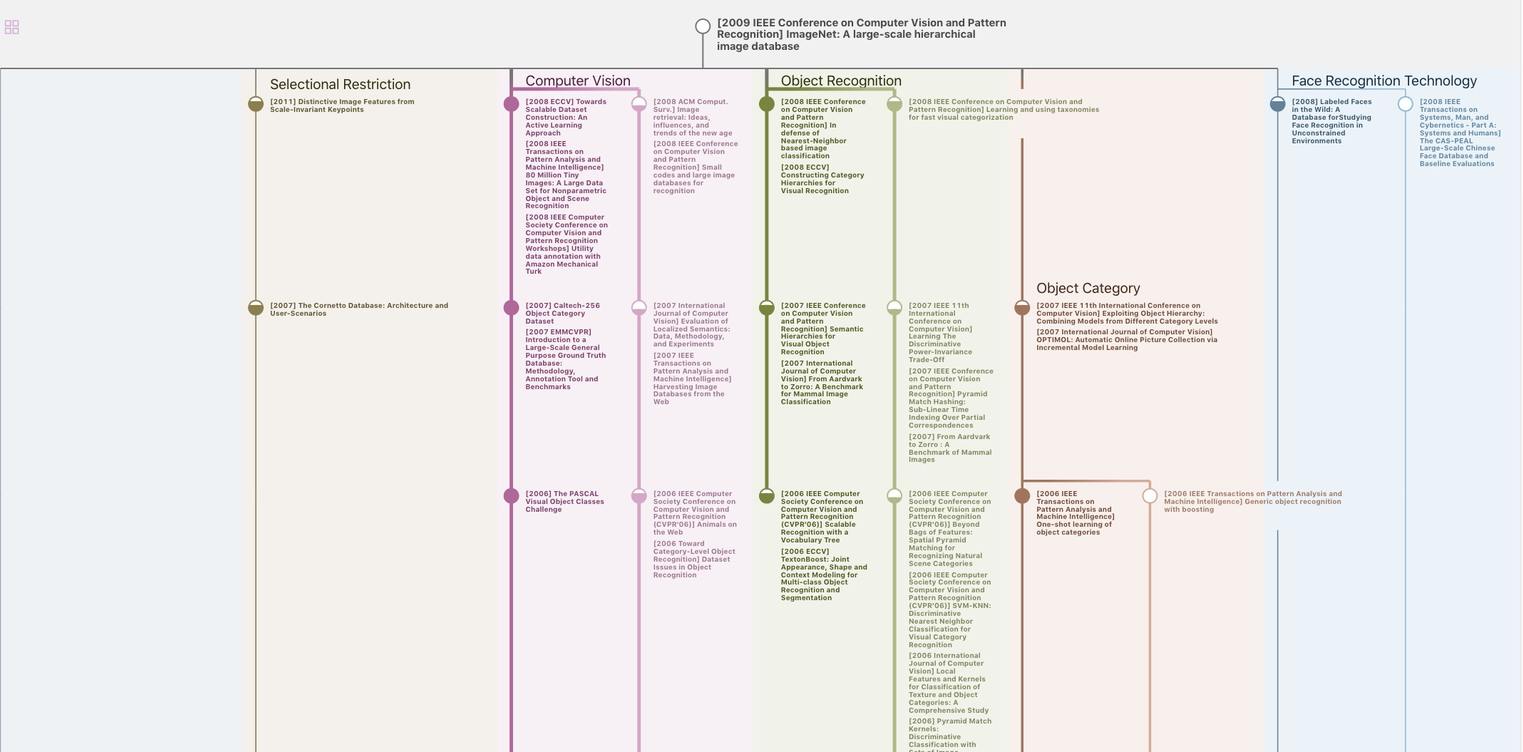
生成溯源树,研究论文发展脉络
Chat Paper
正在生成论文摘要