Attention-Driven Multi-Sensor Selection
2019 INTERNATIONAL JOINT CONFERENCE ON NEURAL NETWORKS (IJCNN)(2019)
摘要
Recent encoder-decoder models for sequence-to-sequence mapping show that integrating both temporal and spatial attention mechanisms into neural networks considerably improve network performance. The use of attention for sensor selection in multi-sensor setups and the benefit of such an attention mechanism is less studied. This work reports on a sensor transformation attention network (STAN) that embeds a sensory attention mechanism to dynamically weigh and combine individual input sensors based on their task-relevant information. We demonstrate the correlation of the attentional signal to changing noise levels of each sensor on the audio-visual GRID dataset and synthetic noise; and on CHiME-4, a multi-microphone real-world noisy dataset. In addition, we demonstrate that the STAN model is able to deal with sensor removal and addition without retraining, and is invariant to channel order. Compared to a two-sensor model that weighs both sensors equally, the equivalent STAN model has a relative parameter increase of only 0.09%, but reduces the relative character error rate (CER) by up to 19.1% on the CHiME-4 dataset. The attentional signal helps to identify a lower SNR sensor with up to 94.2% accuracy.
更多查看译文
关键词
multi-sensor input, attention mechanism, sensor fusion, end-to-end speech recognition
AI 理解论文
溯源树
样例
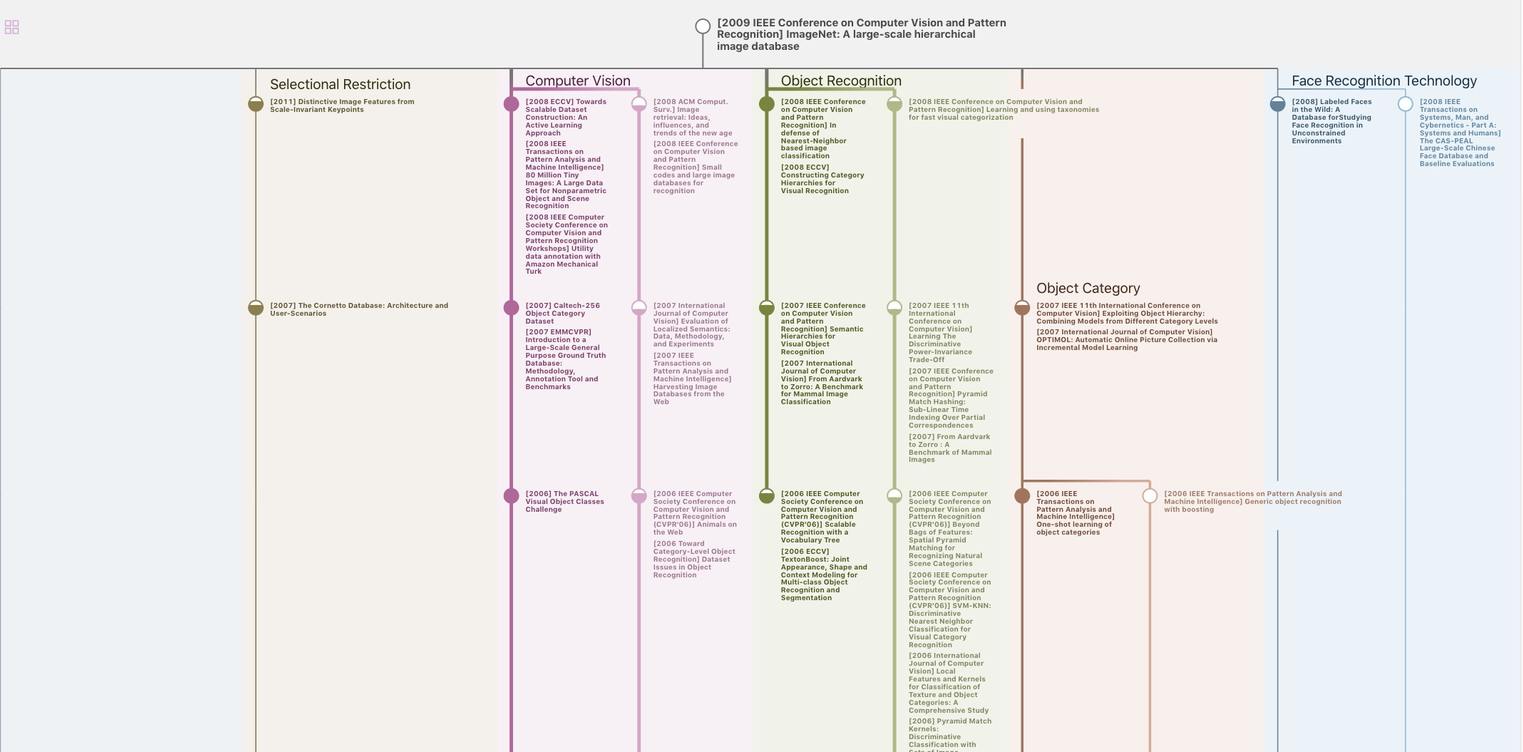
生成溯源树,研究论文发展脉络
Chat Paper
正在生成论文摘要