Hardness Amplification of Optimization Problems.
Electronic Colloquium on Computational Complexity (ECCC)(2019)
摘要
In this paper, we prove a general hardness amplification scheme for optimization problems based on the technique of direct products. We say that an optimization problem $\Pi$ is direct product feasible if it is possible to efficiently aggregate any $k$ instances of $\Pi$ and form one large instance of $\Pi$ such that given an optimal feasible solution to the larger instance, we can efficiently find optimal feasible solutions to all the $k$ smaller instances. Given a direct product feasible optimization problem $\Pi$, our hardness amplification theorem may be informally stated as follows: If there is a distribution $\mathcal{D}$ over instances of $\Pi$ of size $n$ such that every randomized algorithm running in time $t(n)$ fails to solve $\Pi$ on $\frac{1}{\alpha(n)}$ fraction of inputs sampled from $\mathcal{D}$, then, assuming some relationships on $\alpha(n)$ and $t(n)$, there is a distribution $\mathcal{D}'$ over instances of $\Pi$ of size $O(n\cdot \alpha(n))$ such that every randomized algorithm running in time $\frac{t(n)}{poly(\alpha(n))}$ fails to solve $\Pi$ on $\frac{99}{100}$ fraction of inputs sampled from $\mathcal{D}'$. As a consequence of the above theorem, we show hardness amplification of problems in various classes such as NP-hard problems like Max-Clique, Knapsack, and Max-SAT, problems in P such as Longest Common Subsequence, Edit Distance, Matrix Multiplication, and even problems in TFNP such as Factoring and computing Nash equilibrium.
更多查看译文
关键词
optimization problems,hardness,amplification
AI 理解论文
溯源树
样例
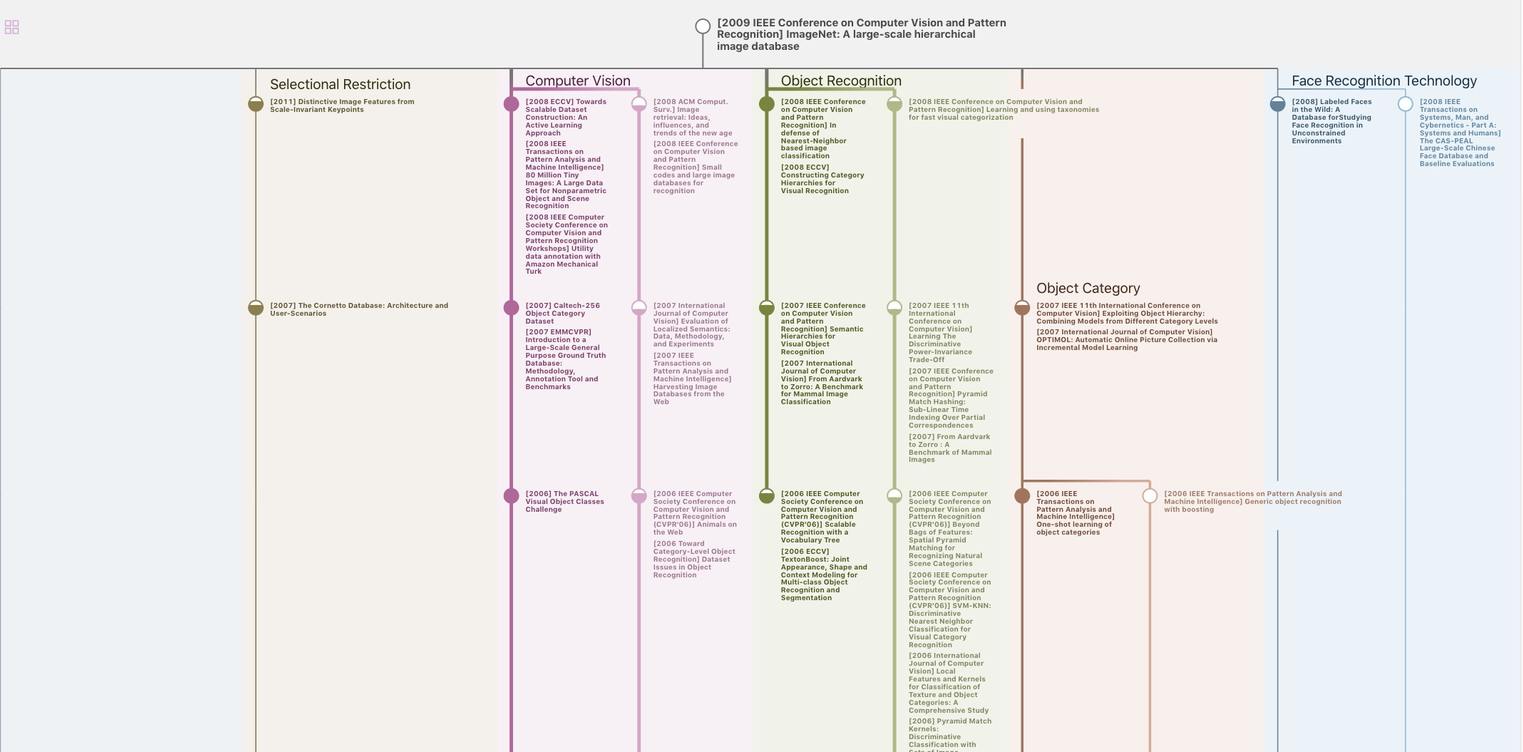
生成溯源树,研究论文发展脉络
Chat Paper
正在生成论文摘要