Content and Colour Distillation for Learning Image Translations with the Spatial Profile Loss
BMVC(2019)
摘要
Generative adversarial networks has emerged as a defacto standard for image translation problems. To successfully drive such models, one has to rely on additional networks e.g., discriminators and/or perceptual networks. Training these networks with pixel based losses alone are generally not sufficient to learn the target distribution. In this paper, we propose a novel method of computing the loss directly between the source and target images that enable proper distillation of shape/content and colour/style. We show that this is useful in typical image-to-image translations allowing us to successfully drive the generator without relying on additional networks. We demonstrate this on many difficult image translation problems such as image-to-image domain mapping, single image super-resolution and photo realistic makeup transfer. Our extensive evaluation shows the effectiveness of the proposed formulation and its ability to synthesize realistic images. [Code release: https://github.com/ssarfraz/SPL]
更多查看译文
关键词
image translations,colour distillation,learning,loss
AI 理解论文
溯源树
样例
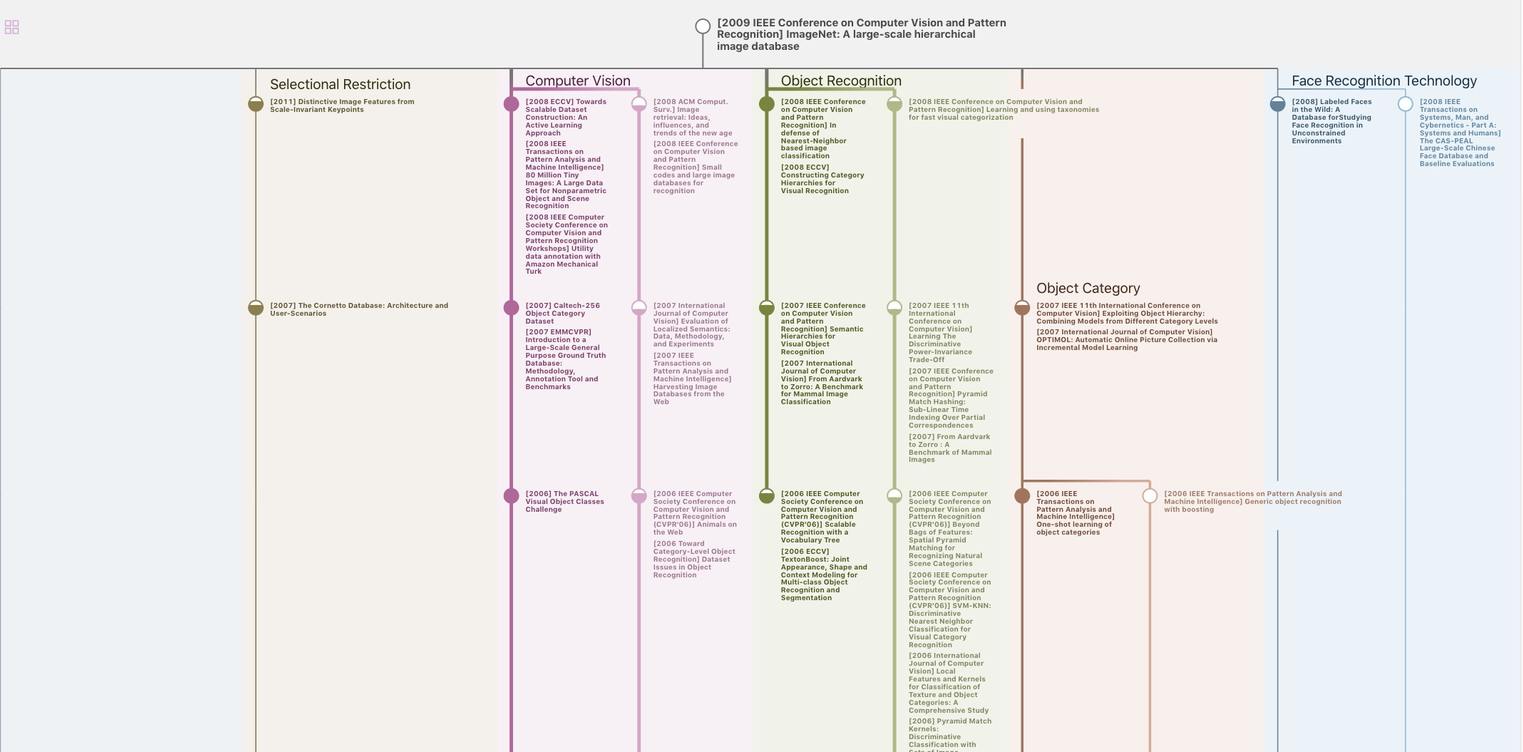
生成溯源树,研究论文发展脉络
Chat Paper
正在生成论文摘要