Rotation Averaging with the Chordal Distance: Global Minimizers and Strong Duality
IEEE Transactions on Pattern Analysis and Machine Intelligence(2021)
摘要
In this paper we explore the role of duality principles within the problem of rotation averaging, a fundamental task in a wide range of applications. In its conventional form, rotation averaging is stated as a minimization over multiple rotation constraints. As these constraints are non-convex, this problem is generally considered challenging to solve globally. We show how to circumvent this difficulty through the use of Lagrangian duality. While such an approach is well-known it is normally not guaranteed to provide a tight relaxation. Based on spectral graph theory, we analytically prove that in many cases there is no duality gap unless the noise levels are severe. This allows us to obtain certifiably global solutions to a class of important non-convex problems in polynomial time. We also propose an efficient, scalable algorithm that outperforms general purpose numerical solvers by a large margin and compares favourably to current state-of-the-art. Further, our approach is able to handle the large problem instances commonly occurring in structure from motion settings and it is trivially parallelizable. Experiments are presented for a number of different instances of both synthetic and real-world data.
更多查看译文
关键词
Rotation averaging,structure from motion,lagrangian duality,graph laplacian,chordal distance
AI 理解论文
溯源树
样例
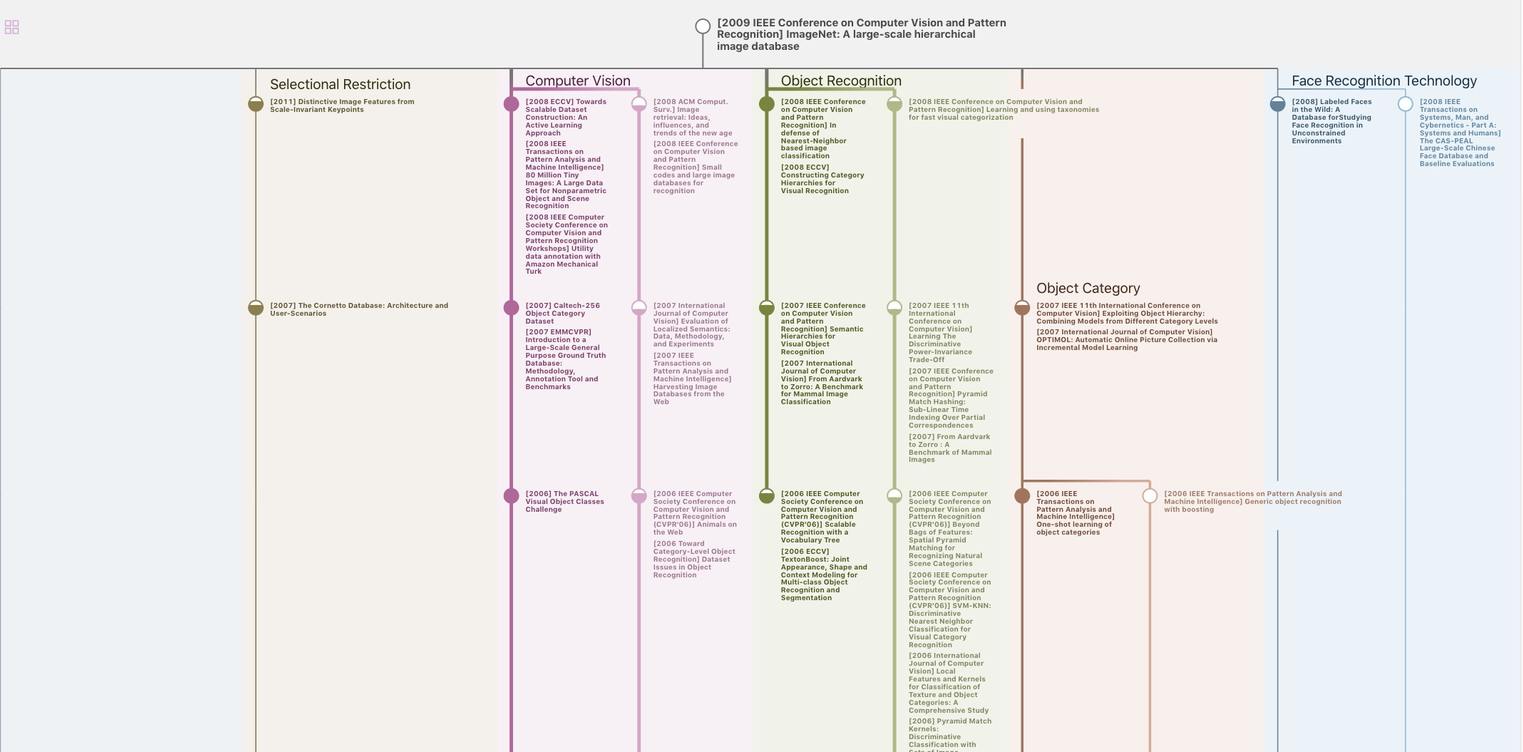
生成溯源树,研究论文发展脉络
Chat Paper
正在生成论文摘要