Deep Reinforcement Learning for Modulation and Coding Scheme Selection in Cognitive HetNets
ICC 2019 - 2019 IEEE International Conference on Communications (ICC)(2019)
摘要
We study a cognitive heterogeneous network (HetNet), in which multiple pairs of secondary users coexist with a pair of primary users on a certain spectrum band. To protect primary transmissions, secondary transmitters (STs) adopt a sensing-based approach to access the spectrum band. Nevertheless, STs may cause uncertain interference to the primary receiver (PR) due to imperfect spectrum sensing, which is particularly significant when the wireless links between the primary transmitter (PT) and STs are extremely weak and the wireless links between STs and the PR are non-ignorable. This makes it difficult for the PR to select a proper modulation and/or coding scheme (MCS). To deal with the issue, we propose an intelligent deep reinforcement learning (DRL) based MCS selection algorithm for the primary transmission. With the proposed algorithm, the DRL agent at the PR is able to learn the pattern of the interference from the STs and predict the interference in the future. Simulation results show that the transmission rate of the proposed algorithm can converge to 90% ^ 100% transmission rate of the optimal MCS selection algorithm, which assumes that the interference from the STs is perfectly known at the PR as prior information. Meanwhile, the transmission rate of the proposed algorithm is around 100% higher than the transmission rate of the benchmark algorithm, which selects the MCS without the information about interference.
更多查看译文
关键词
coding scheme selection,cognitive heterogeneous network,secondary users,primary users,spectrum band,primary transmission,secondary transmitters,sensing-based approach,primary receiver,spectrum sensing,wireless links,primary transmitter,intelligent deep reinforcement learning,optimal MCS selection algorithm,imperfect spectrum sensing,interference information
AI 理解论文
溯源树
样例
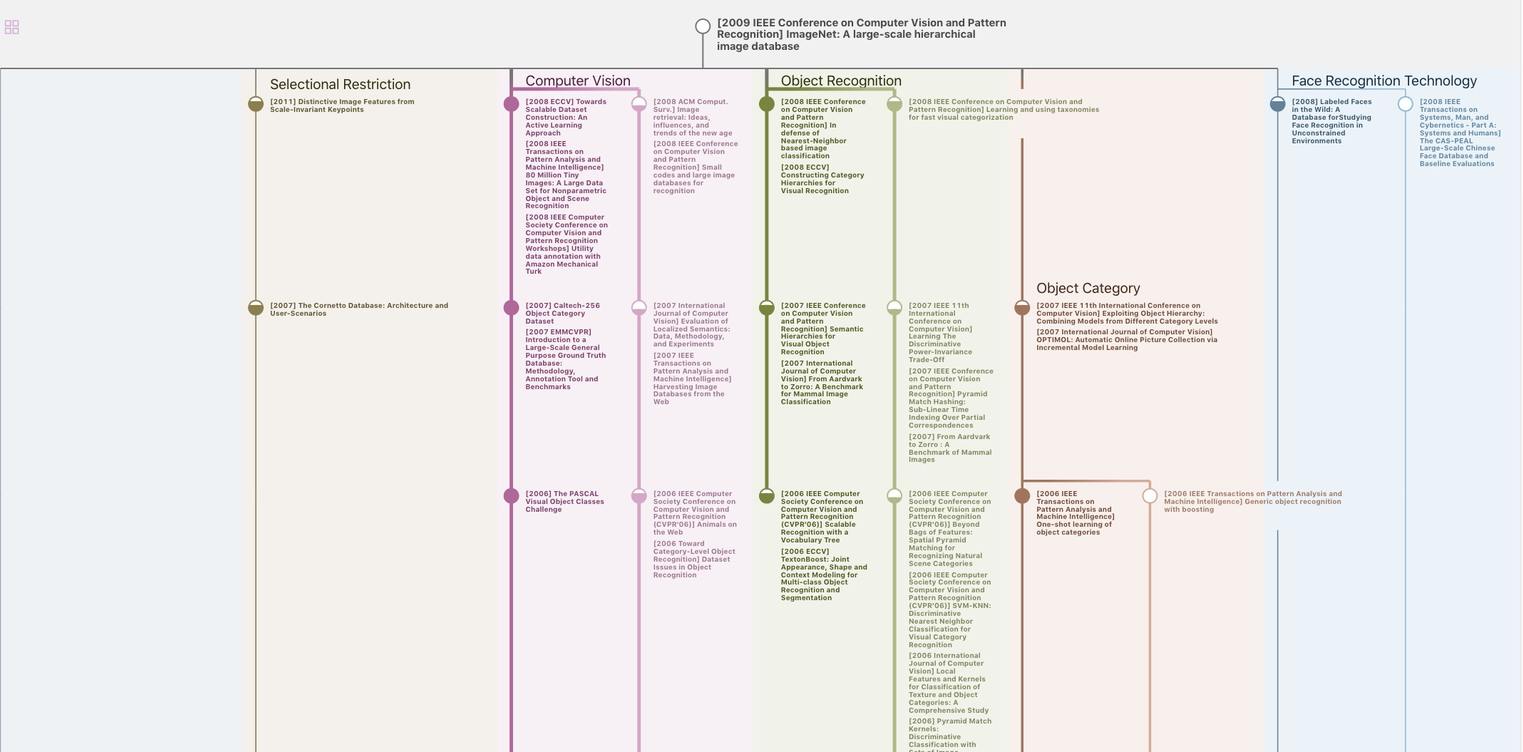
生成溯源树,研究论文发展脉络
Chat Paper
正在生成论文摘要