A Deep Learning Approach for Segmentation of Plain Carbon Steel Microstructure Images
Iet Image Processing(2019)
摘要
To bring about variation in the physical and structural properties or grade of a metal, it is made to undergo specific heat treatment procedures; which can be customized to make the metal microstructure evolve desirably, to obtain specific targeted properties. Recently, computer-based simulations of such heat treatment procedures have become popular, however, such simulations are feasible only if the digital microstructure images are available in suitable forms (optimal digital forms of the microstructure images means the distinct grains identified and the grain boundaries demarcated, i.e., segmentation of microstructure images). To this end, the authors propose a deep learning based Generative Adversarial Network (GAN) architecture for steel microstructure image segmentation. The authors’ experimental results prove the performance efficiency of the proposed GAN model, as compared to the state-of-the-art. However, the proposed network architecture requires large volumes of training data, in the form of annotated ground truth segmentation masks. The current literature lacks sufficient segmented steel microstructure images for this training, to the best of their knowledge. Hence, their second contribution in this study is the development of a Convolutional Neural Network-based framework for sufficient ground truths generation, to aid in the proposed segmentation network training.
更多查看译文
关键词
neural nets,carbon steel,construction industry,learning (artificial intelligence),metallurgy,steel,image segmentation
AI 理解论文
溯源树
样例
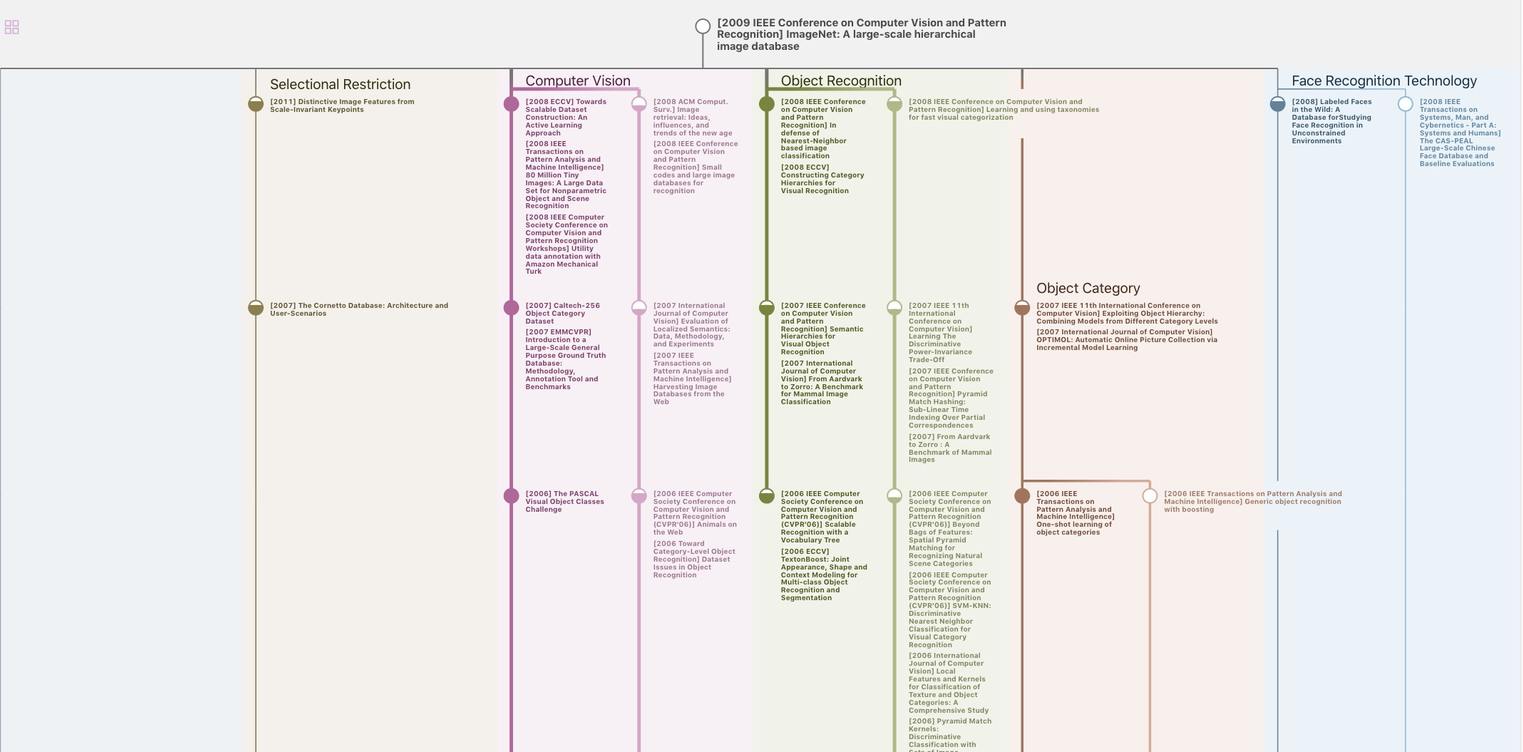
生成溯源树,研究论文发展脉络
Chat Paper
正在生成论文摘要