Stochastic Weight Completion for Road Networks Using Graph Convolutional Networks
2019 IEEE 35th International Conference on Data Engineering (ICDE)(2019)
摘要
Innovations in transportation, such as mobility-on-demand services and autonomous driving, call for high-resolution routing that relies on an accurate representation of travel time throughout the underlying road network. Specifically, the travel time of a road-network edge is modeled as a time-varying distribution that captures the variability of traffic over time and the fact that different drivers may traverse the same edge at the same time at different speeds. Such stochastic weights may be extracted from data sources such as GPS and loop detector data. However, even very large data sources are incapable of covering all edges of a road network at all times. Yet, high-resolution routing needs stochastic weights for all edges. We solve the problem of filling in the missing weights. To achieve that, we provide techniques capable of estimating stochastic edge weights for all edges from traffic data that covers only a fraction of all edges. We propose a generic learning framework called Graph Convolutional Weight Completion (GCWC) that exploits the topology of a road network graph and the correlations of weights among adjacent edges to estimate stochastic weights for all edges. Next, we incorporate contextual information into GCWC to further improve accuracy. Empirical studies using loop detector data from a highway toll gate network and GPS data from a large city offer insight into the design properties of GCWC and its effectiveness.
更多查看译文
关键词
Stochastic processes,Roads,Image edge detection,Histograms,Correlation,Global Positioning System,Detectors
AI 理解论文
溯源树
样例
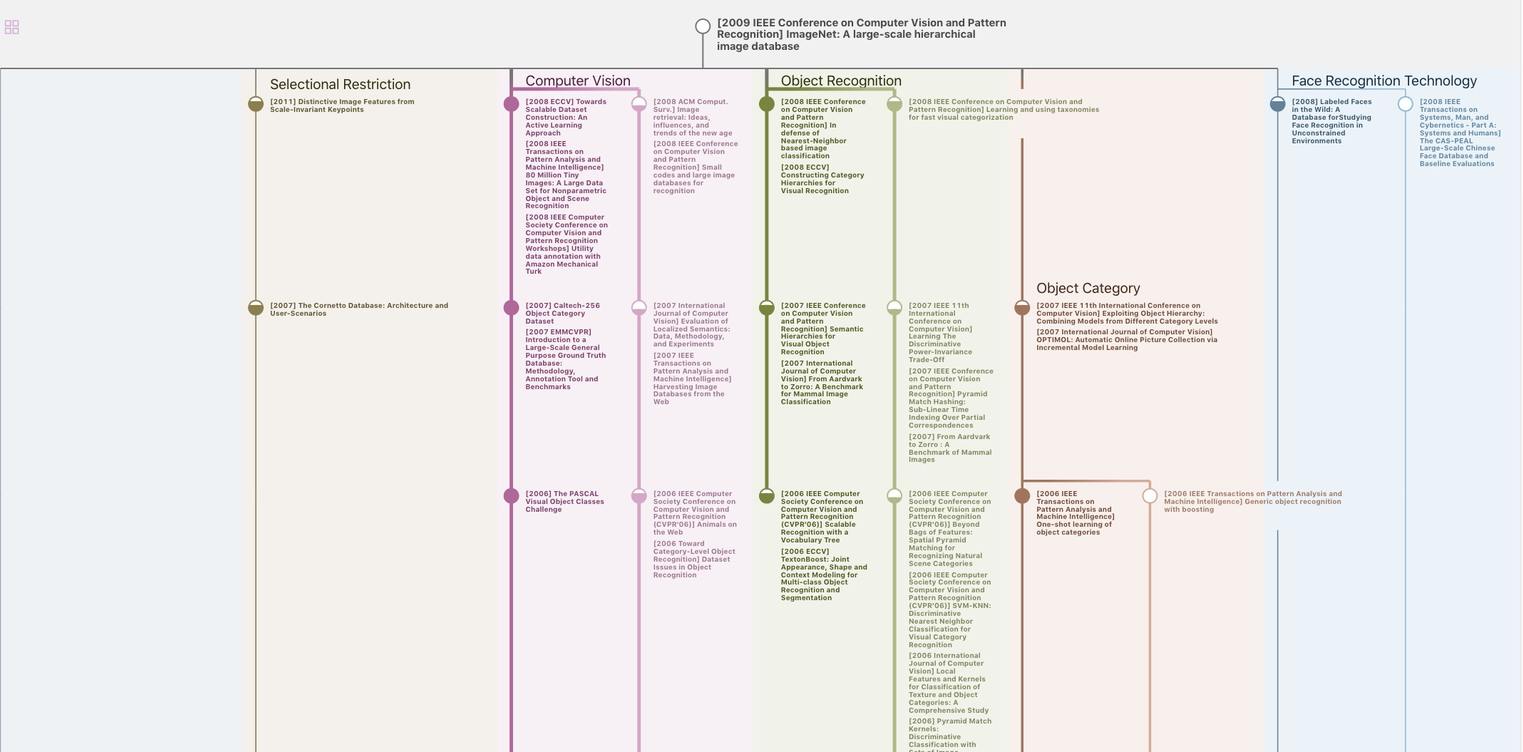
生成溯源树,研究论文发展脉络
Chat Paper
正在生成论文摘要