CodedPrivateML: A Fast and Privacy-Preserving Framework for Distributed Machine Learning
IEEE Journal on Selected Areas in Information Theory(2021)
摘要
How to train a machine learning model while keeping the data private and secure? We present CodedPrivateML, a fast and scalable approach to this critical problem. CodedPrivateML keeps both the data and the model information-theoretically private, while allowing efficient parallelization of training across distributed workers. We characterize CodedPrivateML's privacy threshold and prove its convergence for logistic (and linear) regression. Furthermore, via extensive experiments on Amazon EC2, we demonstrate that CodedPrivateML provides significant speedup over cryptographic approaches based on multi-party computing (MPC).
更多查看译文
关键词
Distributed training,privacy-preserving machine learning
AI 理解论文
溯源树
样例
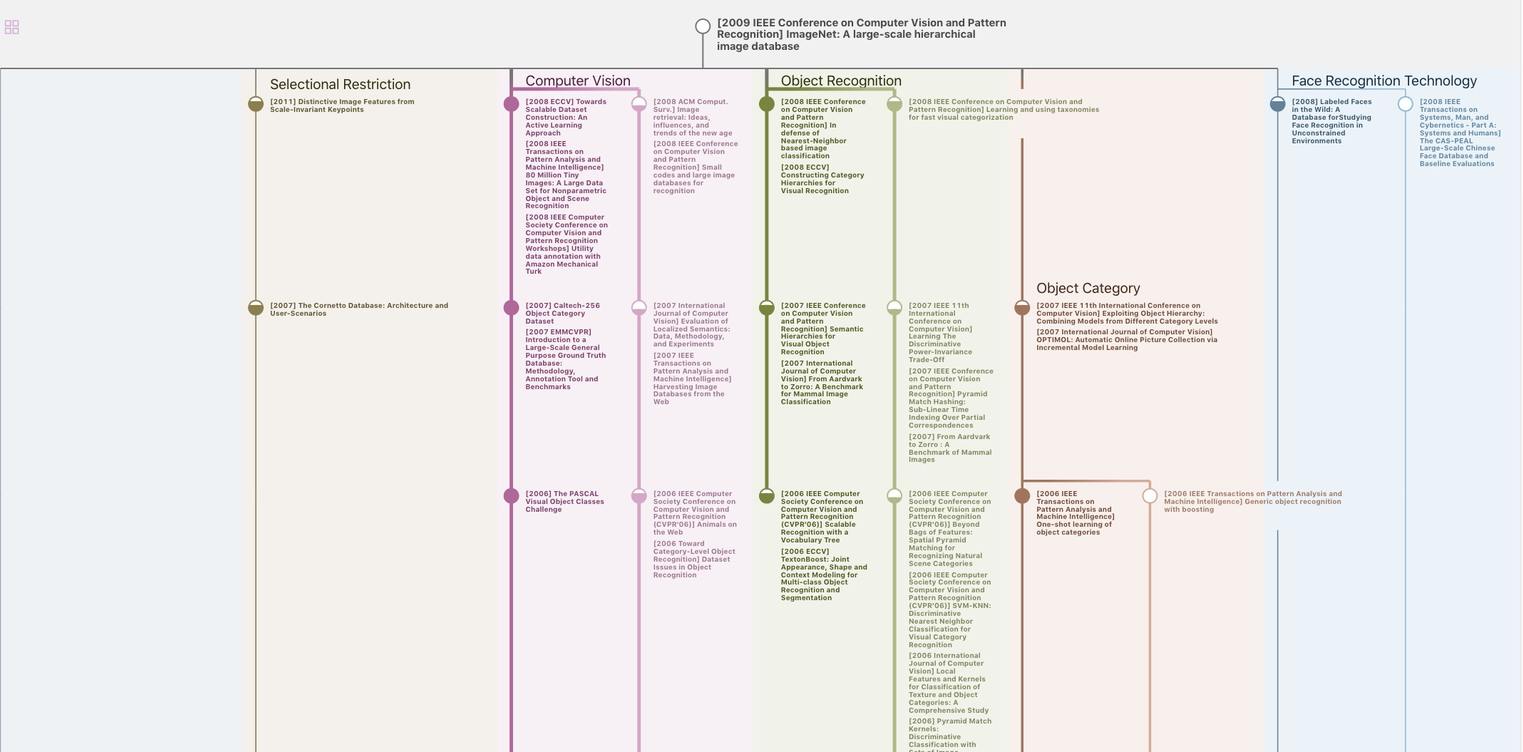
生成溯源树,研究论文发展脉络
Chat Paper
正在生成论文摘要