Deep Spline Networks With Control Of Lipschitz Regularity
2019 IEEE INTERNATIONAL CONFERENCE ON ACOUSTICS, SPEECH AND SIGNAL PROCESSING (ICASSP)(2019)
摘要
The motivation for this work is to improve the performance of deep neural networks through the optimization of the individual activation functions. Since the latter results in an infinite-dimensional optimization problem, we resolve the ambiguity by searching for the sparsest and most regular solution in the sense of Lipschitz. To that end, we first introduce a bound that relates the properties of the pointwise nonlinearities to the global Lipschitz constant of the network. By using the proposed bound as regularizer, we then derive a representer theorem that shows that the optimum configuration is achievable by a deep spline network. It is a variant of a conventional deep ReLU network where each activation function is a piecewise-linear spline with adaptive knots. The practical interest is that the underlying spline activations can be expressed as linear combinations of ReLU units and optimized using l(1)-minimization techniques.
更多查看译文
关键词
Deep learning, Lipschitz regularity, learned activations, deep spline, representer theorem
AI 理解论文
溯源树
样例
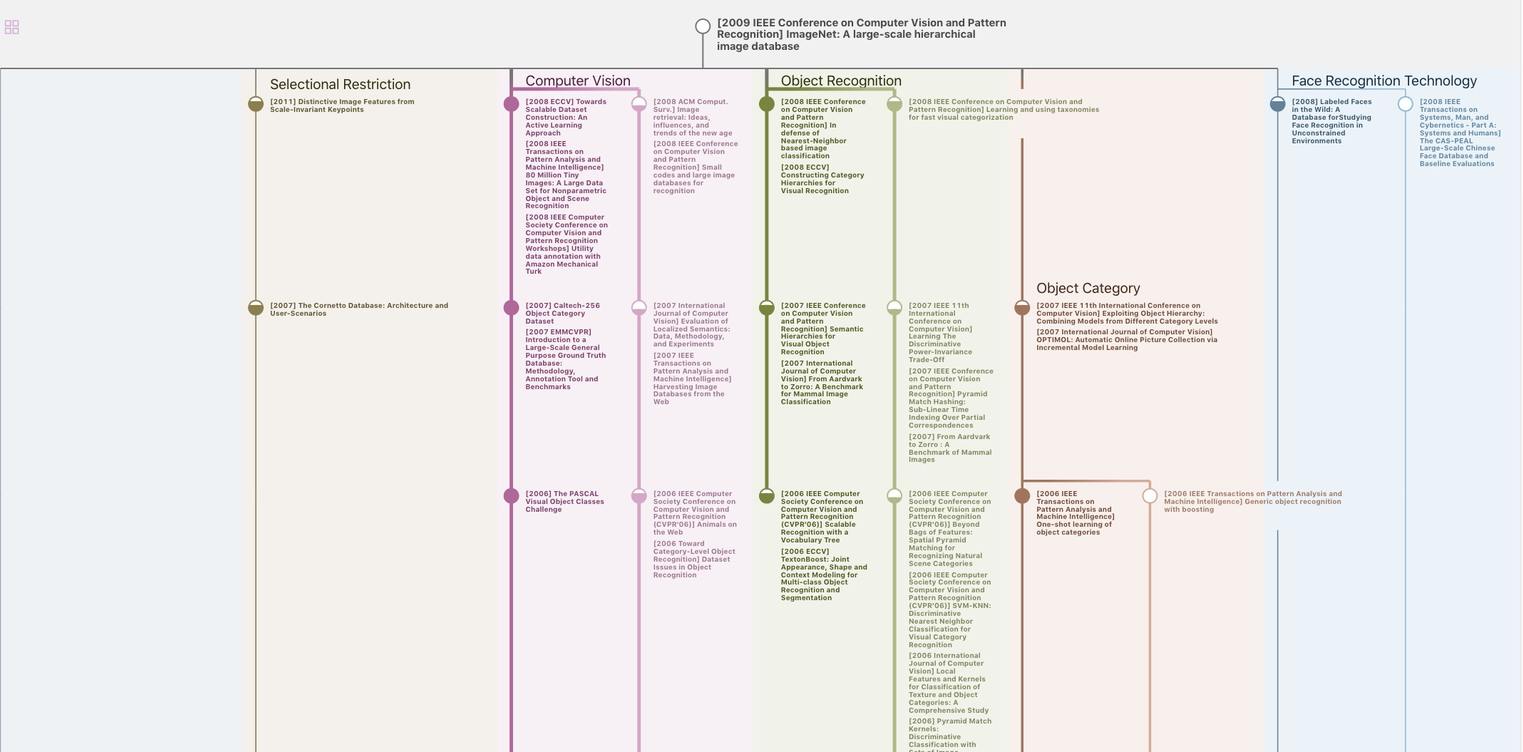
生成溯源树,研究论文发展脉络
Chat Paper
正在生成论文摘要