Parameter Uncertainty For End-To-End Speech Recognition
2019 IEEE INTERNATIONAL CONFERENCE ON ACOUSTICS, SPEECH AND SIGNAL PROCESSING (ICASSP)(2019)
摘要
Recent work on neural networks with probabilistic parameters has shown that parameter uncertainty improves network regularization. Parameter-specific signal-to-noise ratio (SNR) levels derived from parameter distributions were further found to have high correlations with task importance. However, most of these studies focus on tasks other than automatic speech recognition (ASR). This work investigates end-to-end models with probabilistic parameters for ASR. We demonstrate that probabilistic networks outperform conventional deterministic networks in pruning and domain adaptation experiments carried out on the Wall Street Journal and CHiME-4 datasets. We use parameter-specific SNR information to select parameters for pruning and to condition the parameter updates during adaptation. Experimental results further show that networks with lower SNR parameters (1) tolerate increased sparsity levels during parameter pruning and (2) reduce catastrophic forgetting during domain adaptation.
更多查看译文
关键词
end-to-end speech recognition, parameter uncertainty, pruning, adaptation
AI 理解论文
溯源树
样例
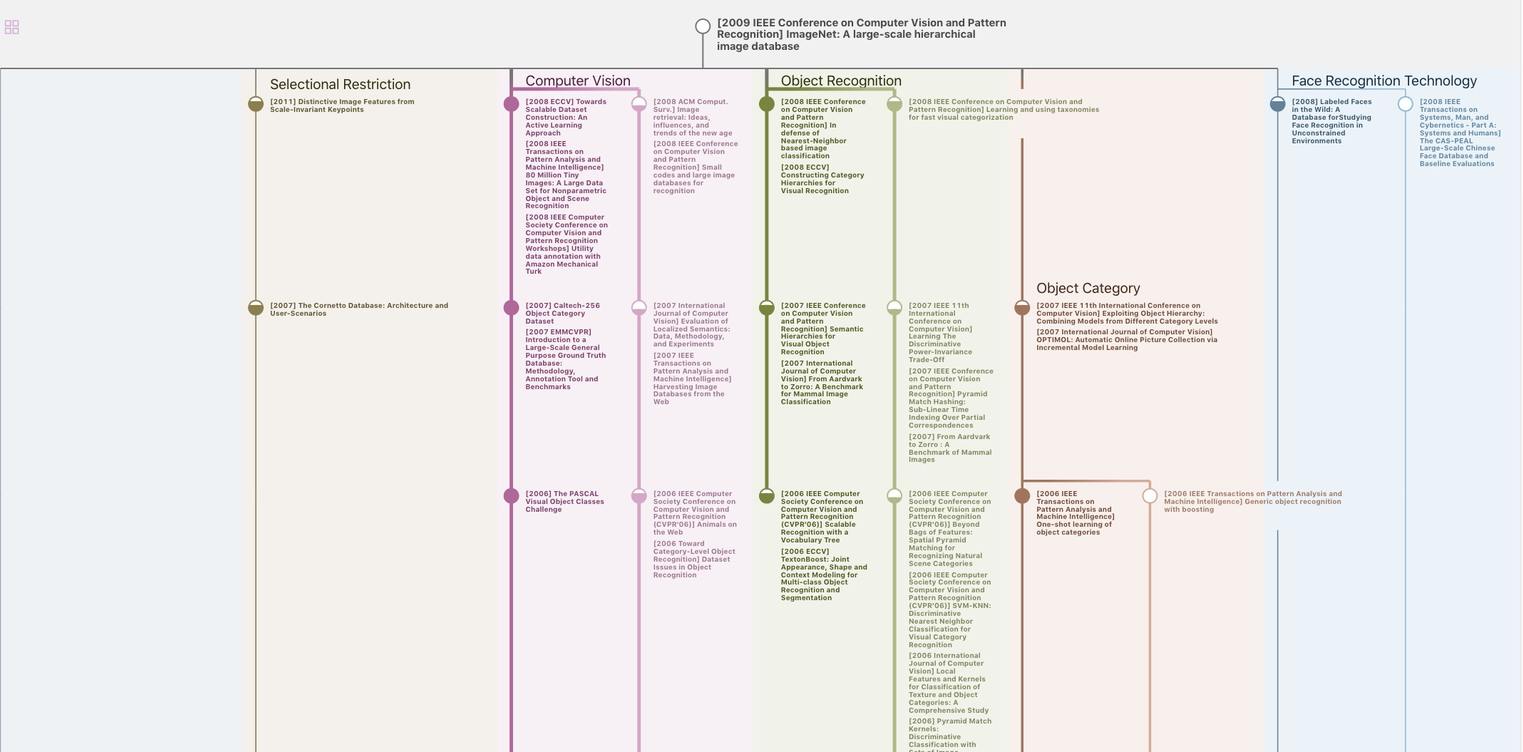
生成溯源树,研究论文发展脉络
Chat Paper
正在生成论文摘要