Identifying Structural Brain Networks From Functional Connectivity: A Network Deconvolution Approach
2019 IEEE INTERNATIONAL CONFERENCE ON ACOUSTICS, SPEECH AND SIGNAL PROCESSING (ICASSP)(2019)
摘要
We address the problem of identifying structural brain networks from signals measured by resting-state functional magnetic resonance imaging (fMRI). To this end, we model functional brain activity as graph signals generated through a linear diffusion process on the unknown structural network. While this is admittedly an oversimplification of the complex mechanisms at work in the brain, recent studies have shown it is an accurate generative model for the second- order statistics of functional signals. We show the diffusion model implies that the signal covariance matrix (a.k.a. functional connectivity) is an unknown polynomial function of the structural network's adjacency matrix. Accordingly, we advocate a network deconvolution approach whereby we: (i) use the fMRI signals to estimate the eigenvectors of the structural network from those of the empirical covariance; and (ii) solve a convex, sparsity- regularized inverse problem to recover the eigenvalues that were obscured by diffusion. The inferred structural networks capture some key patterns that match known pathology in attention deficit/hyper activity disorder. We also offer preliminary evidence supporting their role as potential biomarkers for subject diagnosis and classification.
更多查看译文
关键词
Brain network inference, functional signals, graph signal processing, diffusion process, network deconvolution
AI 理解论文
溯源树
样例
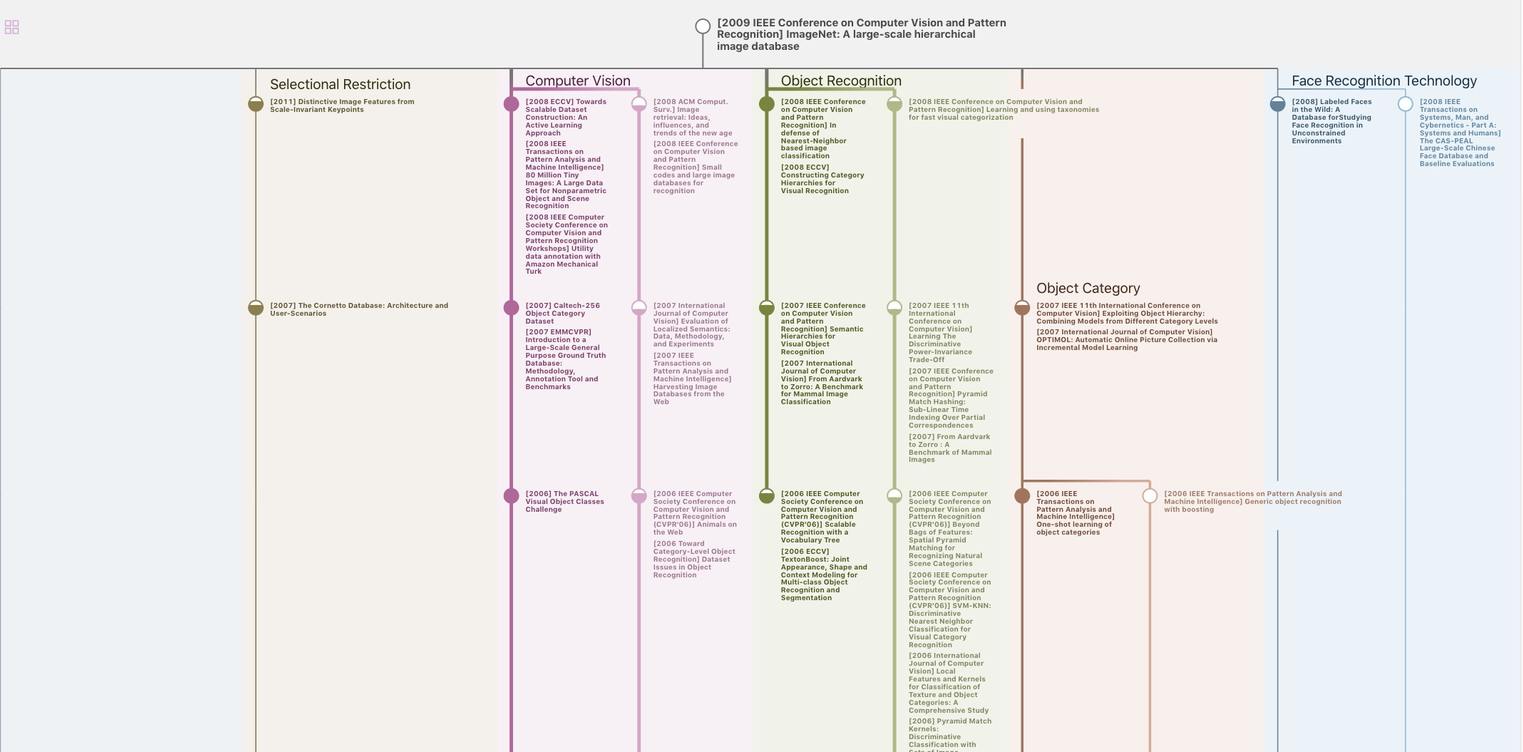
生成溯源树,研究论文发展脉络
Chat Paper
正在生成论文摘要