Sampling Of One-Dimensional Probability Measures In The Convex Order And Computation Of Robust Option Price Bounds
INTERNATIONAL JOURNAL OF THEORETICAL AND APPLIED FINANCE(2019)
摘要
For mu and nu two probability measures on the real line such that mu is smaller than nu in the convex order, this property is in general not preserved at the level of the empirical measures mu(I) = 1/I Sigma(I )(i=)(1)delta(Xi) and nu(J )= 1/J Sigma(J )(j=1)delta(Yj), where (X-i)(1 <= i <= I) (resp., (Y-j)(1 <= j <= J)) are independent and identically distributed according to mu (resp., nu). We investigate modifications of mu(I) (resp., nu(J)) smaller than nu(J )(resp., greater than mu(I)) in the convex order and weakly converging to mu (resp., nu) as I, J -> infinity. According to Kertz & Rosler(1992), the set of probability measures on the real line with a finite first order moment is a complete lattice for the increasing and the decreasing convex orders. For mu and nu in this set, this enables us to define a probability measure mu boolean OR nu (resp., mu boolean AND nu) greater than mu (resp., smaller than nu) in the convex order. We give efficient algorithms permitting to compute mu boolean OR nu and mu boolean AND nu (and therefore mu(I) boolean OR nu(J )and mu(I) boolean AND nu(J)) when mu and nu have finite supports. Last, we illustrate by numerical experiments the resulting sampling methods that preserve the convex order and their application to approximate martingale optimal transport problems and in particular to calculate robust option price bounds.
更多查看译文
关键词
Convex order, martingale optimal transport, robust option price bounds, sampling techniques, linear programming
AI 理解论文
溯源树
样例
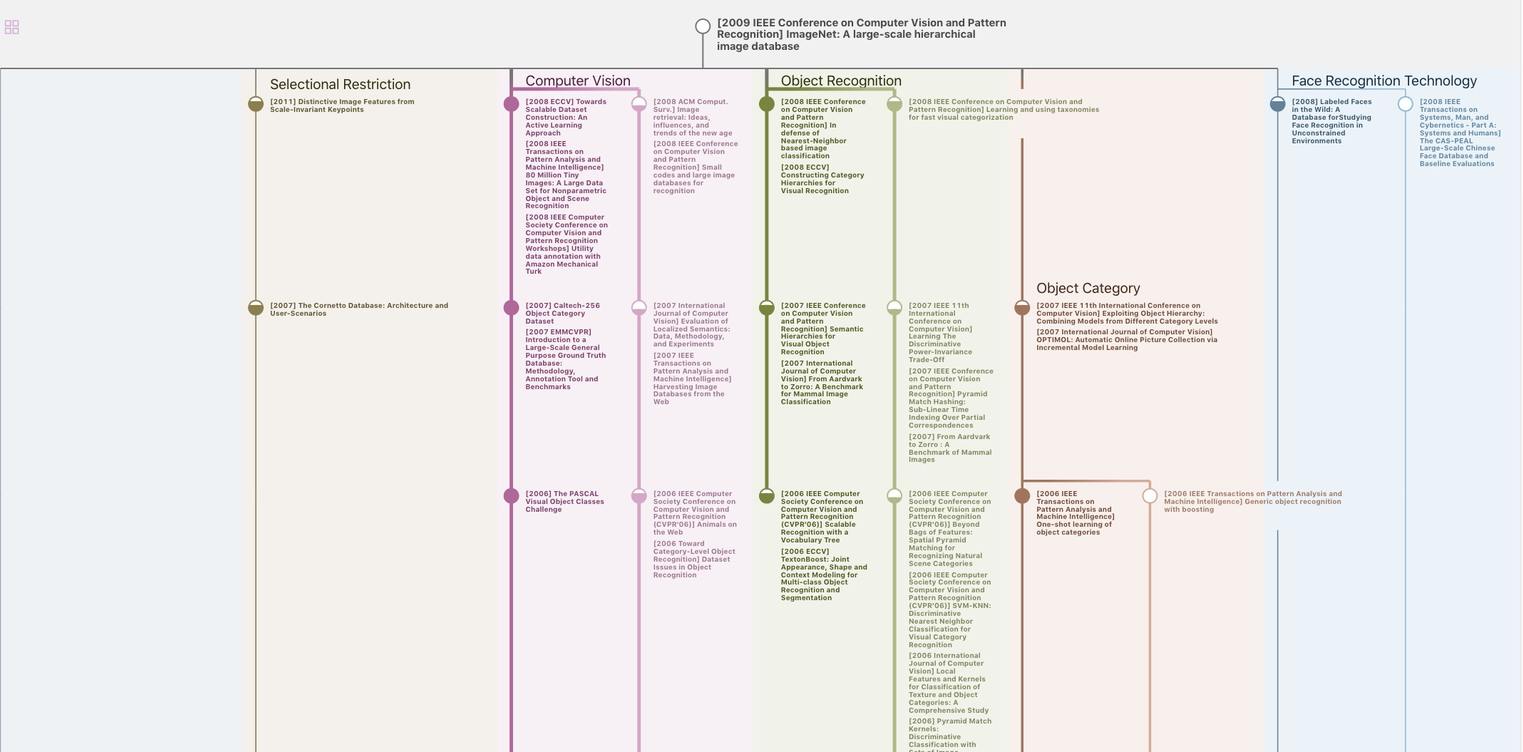
生成溯源树,研究论文发展脉络
Chat Paper
正在生成论文摘要