List Decodable Learning via Sum of Squares
PROCEEDINGS OF THE THIRTY-FIRST ANNUAL ACM-SIAM SYMPOSIUM ON DISCRETE ALGORITHMS (SODA'20)(2020)
摘要
In the list-decodable learning setup, an overwhelming majority (say a 1 - beta-fraction) of the input data consists of outliers and the goal of an algorithm is to output a small list L of hypotheses such that one of them agrees with inliers. We devise list decodable learning algorithms for the problem of linear regression using the sum of squares SDP hierarchy. In the list-decodable linear regression problem, we are given labelled examples {(X-i, y(i))}(i is an element of[N]) containing a subset S of beta N inliers {X-i}(i is an element of S) that are drawn i.i.d. from standard Gaussian distribution N(0, I) in R-d, where the corresponding labels y(i) are well-approximated by a linear function (l) over cap. We devise an algorithm that outputs a list L of linear functions such that there exists some l is an element of L that is close to This yields the first algorithm for linear regression in a listdecodable setting. Our results hold for a general distribution of examples whose concentration and anti-concentration properties can be certified by low degree sum-of-squares proofs. In an independent and concurrent work, Karmalkar et al. [K. K K19] also obtain an algorithm for list-decodable linear regression using the Sum-of-Squares SDP hierarchy.
更多查看译文
AI 理解论文
溯源树
样例
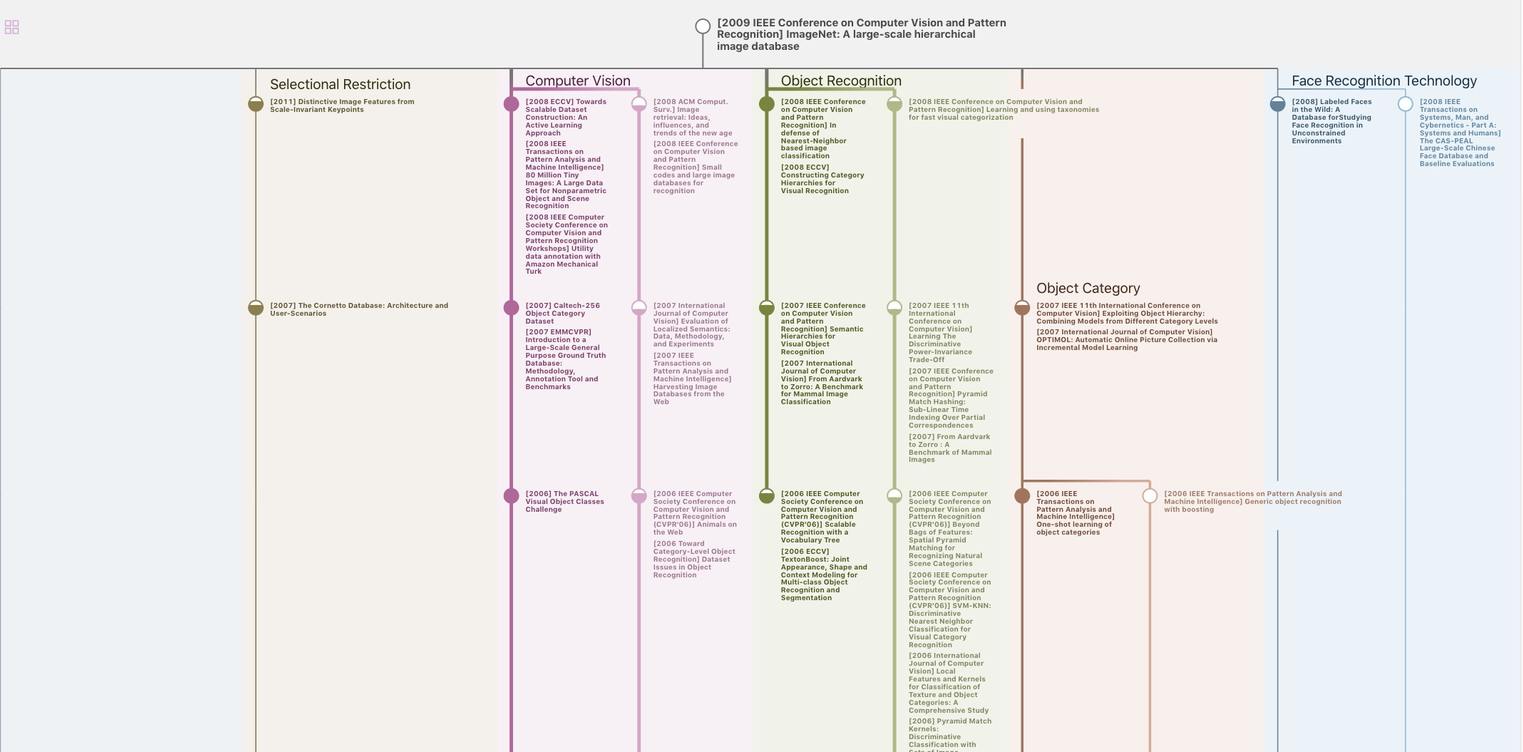
生成溯源树,研究论文发展脉络
Chat Paper
正在生成论文摘要