Autoencoders For Training Compact Deep Learning Rf Classifiers For Wireless Protocols
2019 IEEE 20TH INTERNATIONAL WORKSHOP ON SIGNAL PROCESSING ADVANCES IN WIRELESS COMMUNICATIONS (SPAWC 2019)(2019)
摘要
We show that compact fully connected (FC) deep learning networks trained to classify wireless protocols using a hierarchy of multiple denoising autoencoders (AEs) outperform reference FC networks trained in a typical way, i.e., with a stochastic gradient based optimization of a given FC architecture. Not only is the complexity of such FC network, measured in number of trainable parameters and scalar multiplications, much lower than the reference FC and residual models, its accuracy also outperforms both models for nearly all tested SNR values (0 dB to 50dB). Such AE-trained networks are suited for in-situ protocol inference performed by simple mobile devices based on noisy signal measurements. Training is based on the data transmitted by real devices, and collected in a controlled environment, and systematically augmented by a policy-based data synthesis process by adding to the signal any subset of impairments commonly seen in a wireless receiver.
更多查看译文
关键词
AE-trained networks,in-situ protocol inference,noisy signal measurements,policy-based data synthesis process,wireless receiver,wireless protocols,multiple denoising autoencoders,stochastic gradient based optimization,FC network,scalar multiplications,residual models,FC architecture,SNR values,deep learning RF classifiers,fully connected deep learning networks,noise figure 0.0 dB to 50.0 dB
AI 理解论文
溯源树
样例
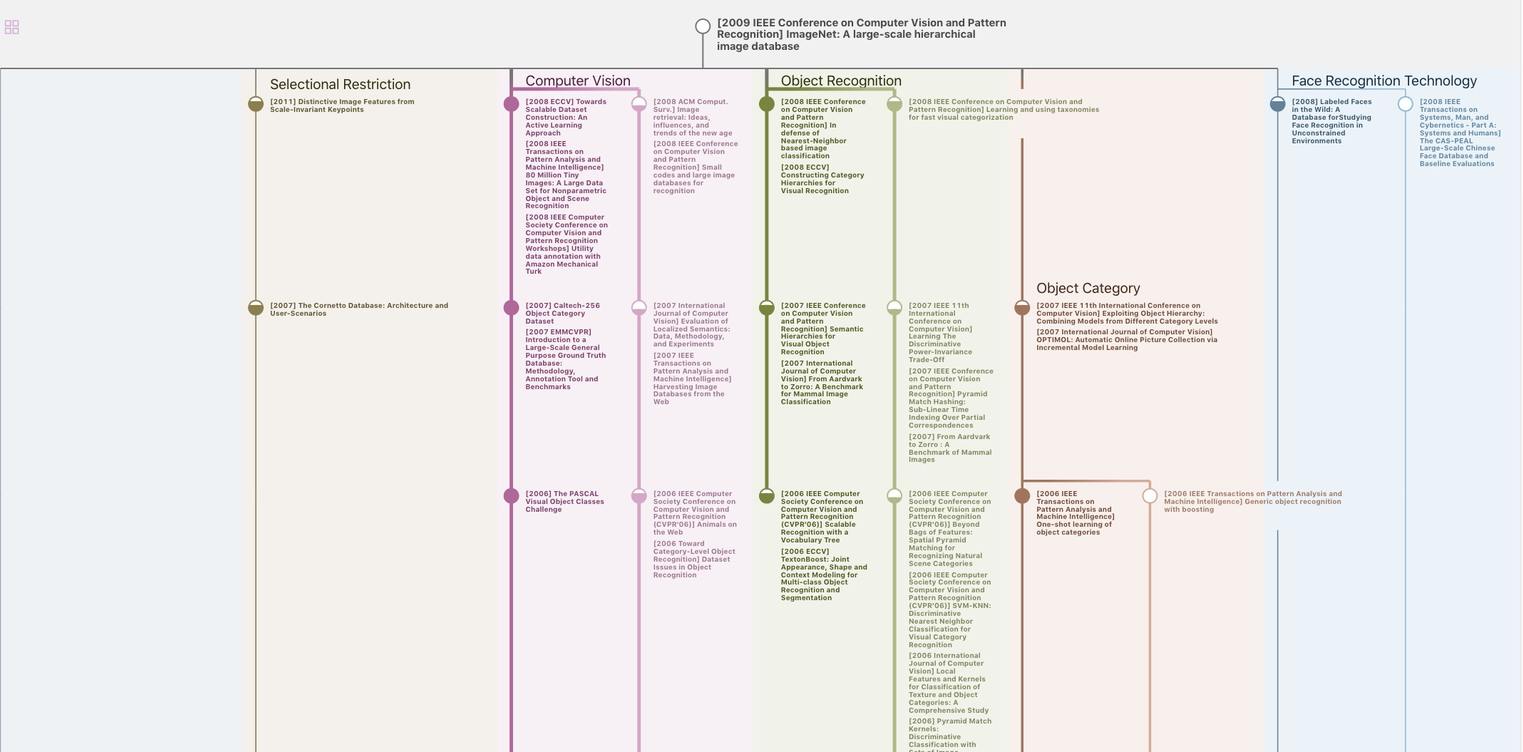
生成溯源树,研究论文发展脉络
Chat Paper
正在生成论文摘要