Efficient Window Aggregation with General Stream Slicing.
EDBT(2019)
摘要
Window aggregation is a core operation in data stream processing. Existing aggregation techniques focus on reducing latency, eliminating redundant computations, and minimizing memory usage. However, each technique operates under different assumptions with respect to workload characteristics such as properties of aggregation functions (e.g., invertible, associative), window types (e.g., sliding, sessions), windowing measures (e.g., time- or count-based), and stream (dis)order. Violating the assumptions of a technique can deem it unusable or drastically reduce its performance. In this paper, we present the first general stream slicing technique for window aggregation. General stream slicing automatically adapts to workload characteristics to improve performance without sacrificing its general applicability. As a prerequisite, we identify workload characteristics which affect the performance and applicability of aggregation techniques. Our experiments show that general stream slicing outperforms alternative concepts by up to one order of magnitude.
更多查看译文
关键词
efficient window aggregation,general stream slicing
AI 理解论文
溯源树
样例
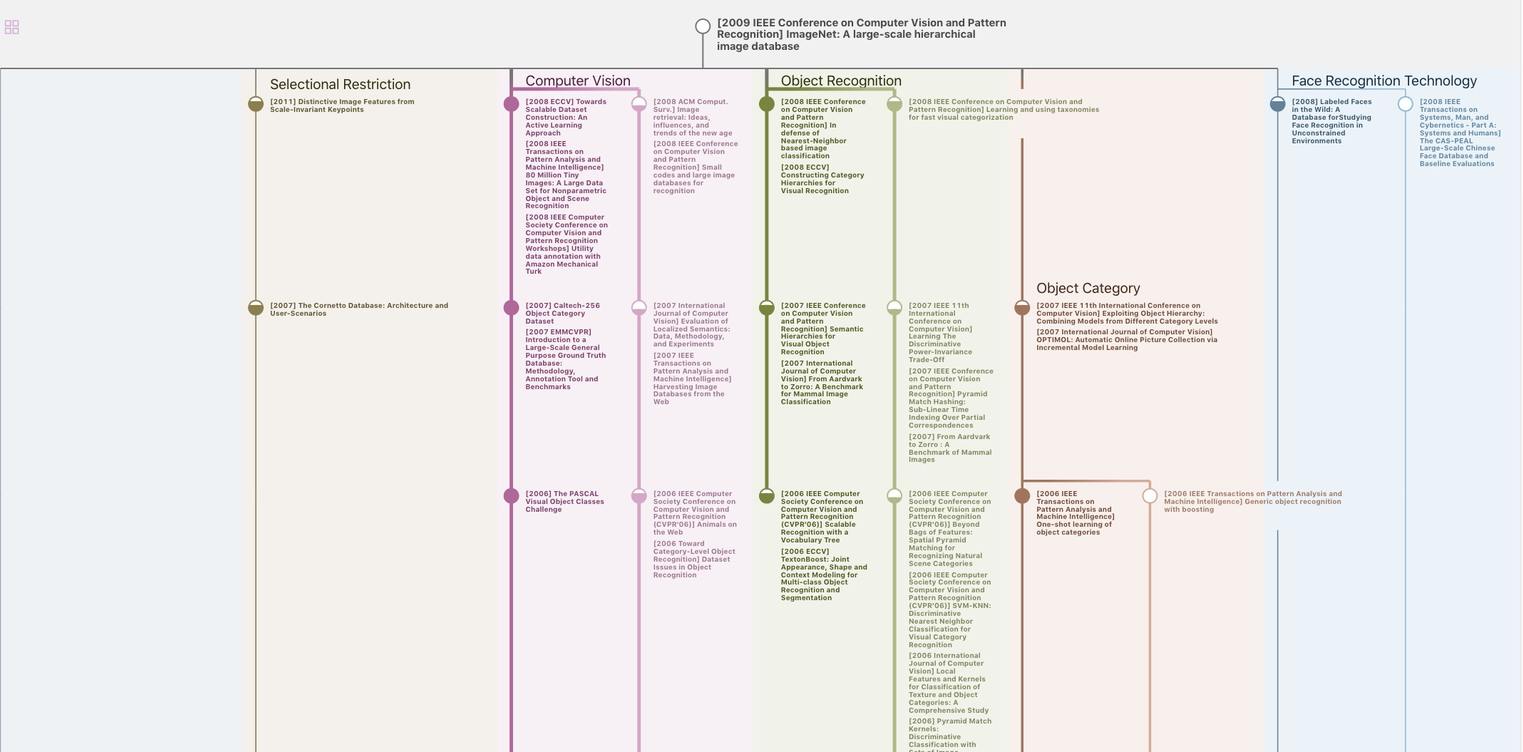
生成溯源树,研究论文发展脉络
Chat Paper
正在生成论文摘要