Qualitative and Descriptive Topic Extraction from Movie Reviews Using LDA.
MLDM(2017)
摘要
Crowdsourced review services such as IMDB or Rotten Tomatoes provide numerical ratings and raw text reviews to help users decide what movie to watch. As the amount of reviews per movie can be very large, selecting a movie among a catalog can be a tedious task. This problem can be addressed by providing the user the most relevant reviews or building automatic reviews summaries. We take a different approach by predicting personalized movie description from text reviews using Latent Dirichlet Allocation (LDA) based topic models. Our models extract distinct qualitative and descriptive topics by combining text reviews and movie ratings in a joint probabilistic model. We evaluate our models on an IMDB dataset and illustrate its performance through comparison of topics.
更多查看译文
AI 理解论文
溯源树
样例
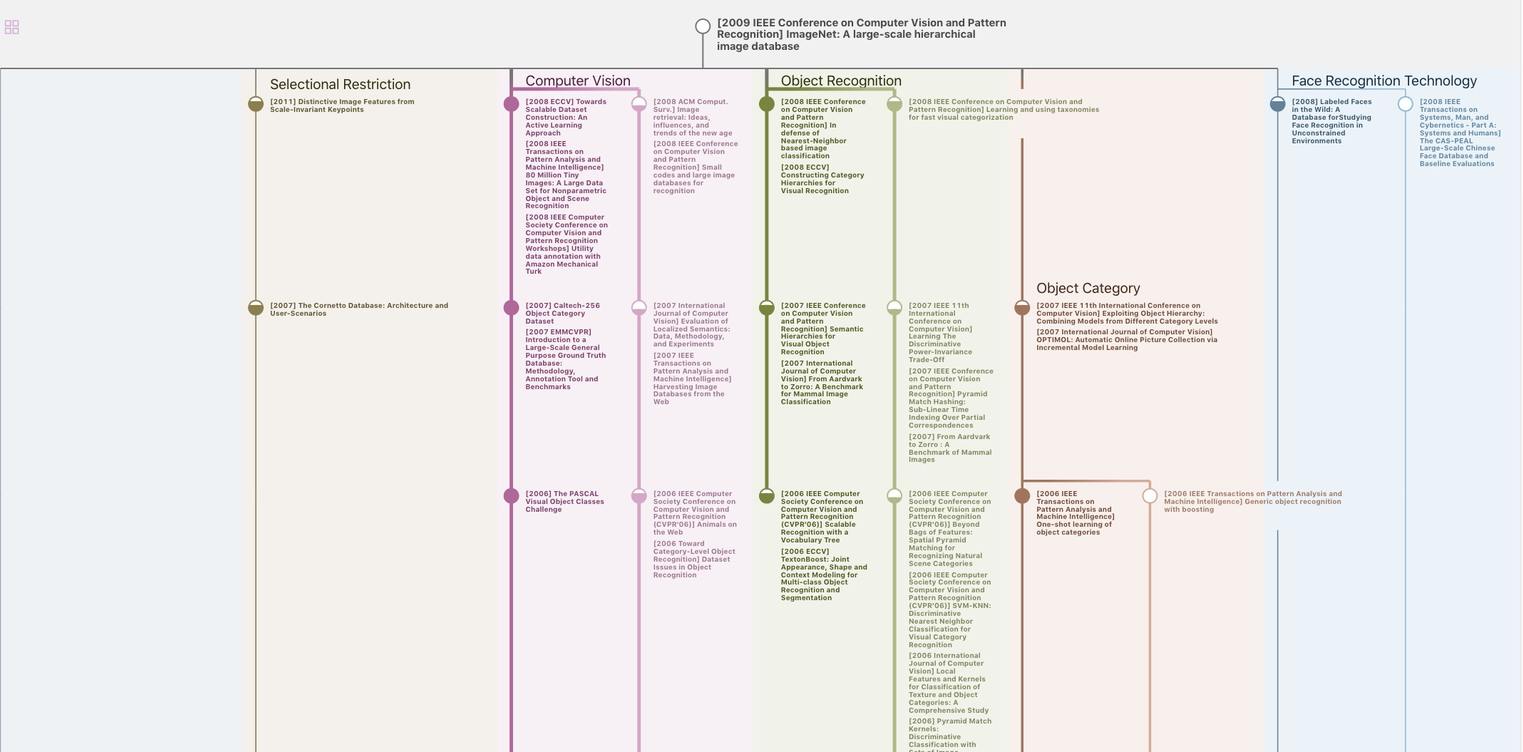
生成溯源树,研究论文发展脉络
Chat Paper
正在生成论文摘要