Interpretable recommendations via overlapping co-clusters.
arXiv: Information Retrieval(2016)
摘要
There is an increasing need to provide not only accurate but also interpretable recommendations, in order to enhance transparency and trust in the recommendation process. This is particularly important in a business-to-business setting, where recommendations are generated for experienced sales staff and not directly for the end-client. In this paper, we consider the problem of generating interpretable recommendations based on the purchase history of clients, or more general, based on positive or one-class ratings only. We present an algorithm that generates recommendations by identifying overlapping co-clusters consisting of clients and products. Our algorithm uses matrix factorization techniques to identify co-clusters, and recommends a client-product pair because of its membership in one or more client-product co-clusters. The algorithm exhibits linear complexity in the number of co-clusters and input examples, and can therefore be applied to very large datasets. We show, both on a real client-product dataset from our institution, as well as on publicly available datasets, that the recommendation accuracy of our algorithm is better than or equivalent to standard interpretable and non-interpretable recommendation techniques, such as standard one-class nearest neighbor and matrix factorization techniques. Most importantly, our approach is capable of offering textually and visually interpretable recommendations.
更多查看译文
AI 理解论文
溯源树
样例
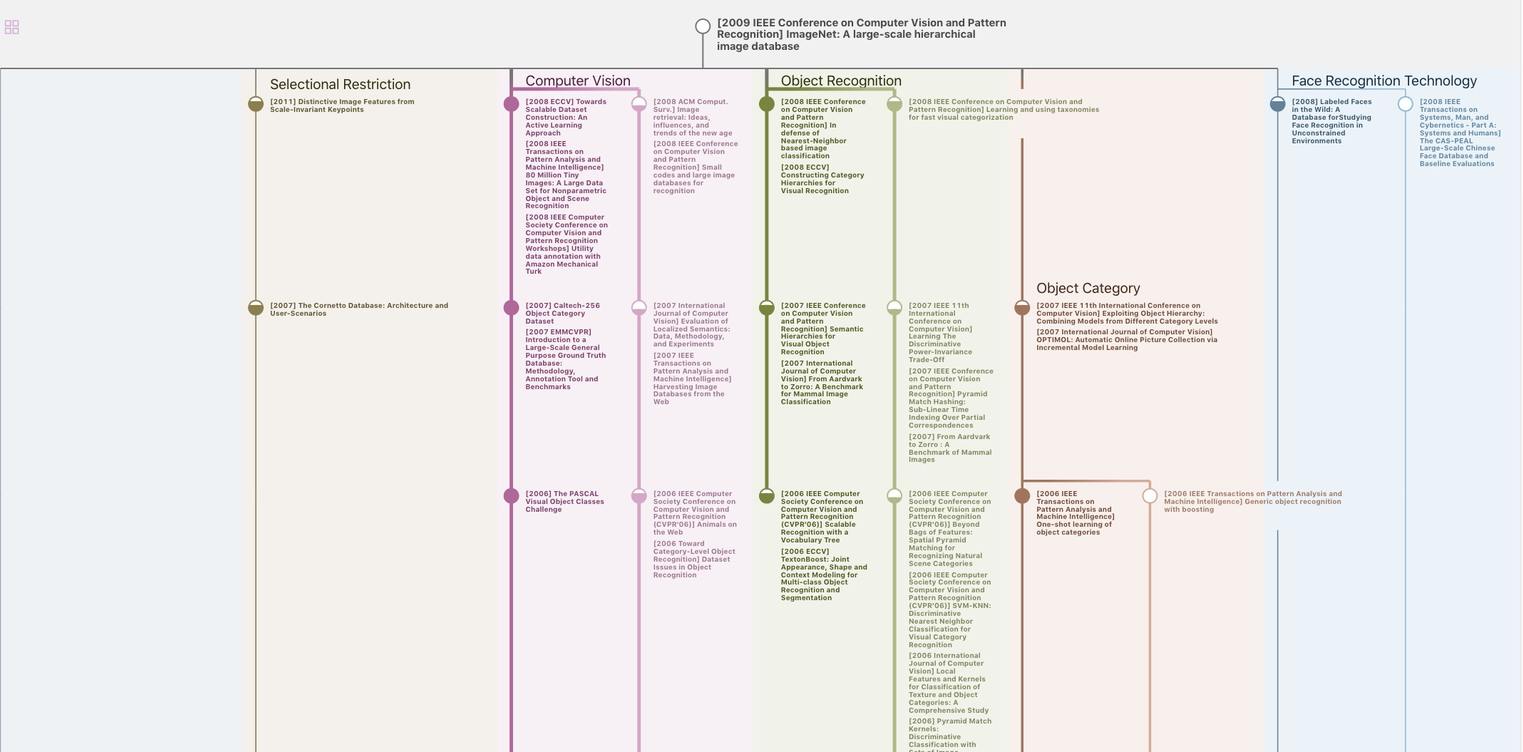
生成溯源树,研究论文发展脉络
Chat Paper
正在生成论文摘要