Cooperative Co-Evolution With Improved Differential Grouping Method For Large-Scale Global Optimisation
INTERNATIONAL JOURNAL OF BIO-INSPIRED COMPUTATION(2018)
摘要
The cooperative co-evolution (CC) framework has shown to be effective in dealing with large-scale global optimisation (LSGO). However, the performance of algorithms based on the CC framework is often affected by the chosen variable grouping method, i.e., how variables are grouped into different sub-components. In this study, an improved variable grouping strategy based on the differential grouping (DG) is proposed, namely epsilon-based differential grouping (epsilon-DG). The epsilon-DG strategy can identify both direct and indirect interactions between variables. Moreover, a simple yet effective method is introduced in epsilon-DG to identify the calculation error that is detrimental to variable grouping in the original DG method (which needs to set the threshold value appropriately). The epsilon-DG is compared against the DG on the CEC 2010 LSGO benchmarks, and is found to perform better in terms of the grouping accuracy on almost all problems. Moreover, a CC-based differential evolution algorithm with the epsilon-DG strategy shows good performance on the 2010 LSGO benchmarks.
更多查看译文
关键词
large-scale global optimisation, LSGO, variable grouping strategy, differential grouping, differential evolution, evolutionary algorithms
AI 理解论文
溯源树
样例
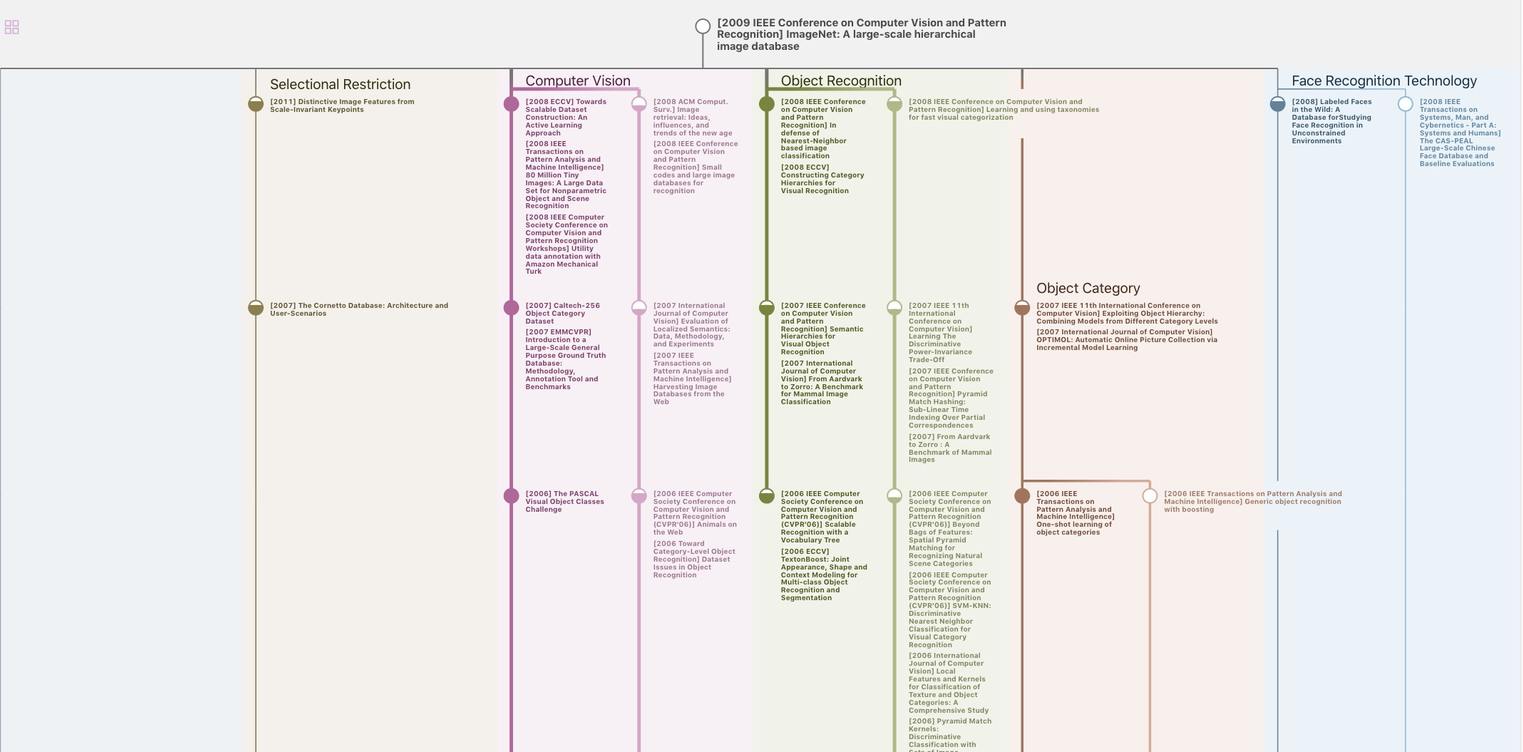
生成溯源树,研究论文发展脉络
Chat Paper
正在生成论文摘要