Staggered Time Average Algorithm for Stochastic Non-smooth Optimization with O(1/T) Convergence
arXiv: Optimization and Control(2016)
摘要
Stochastic non-smooth convex optimization constitutes a class of problems in machine learning and operations research. This paper considers minimization of a non-smooth function based on stochastic subgradients. When the function has a locally polyhedral structure, a staggered time average algorithm is proven to have O(1/T) convergence rate. A more general convergence result is proven when the locally polyhedral assumption is removed. In that case, the convergence bound depends on the curvature of the function near the minimum. Finally, the locally polyhedral assumption is shown to improve convergence beyond O(1/T) for a special case of deterministic problems.
更多查看译文
AI 理解论文
溯源树
样例
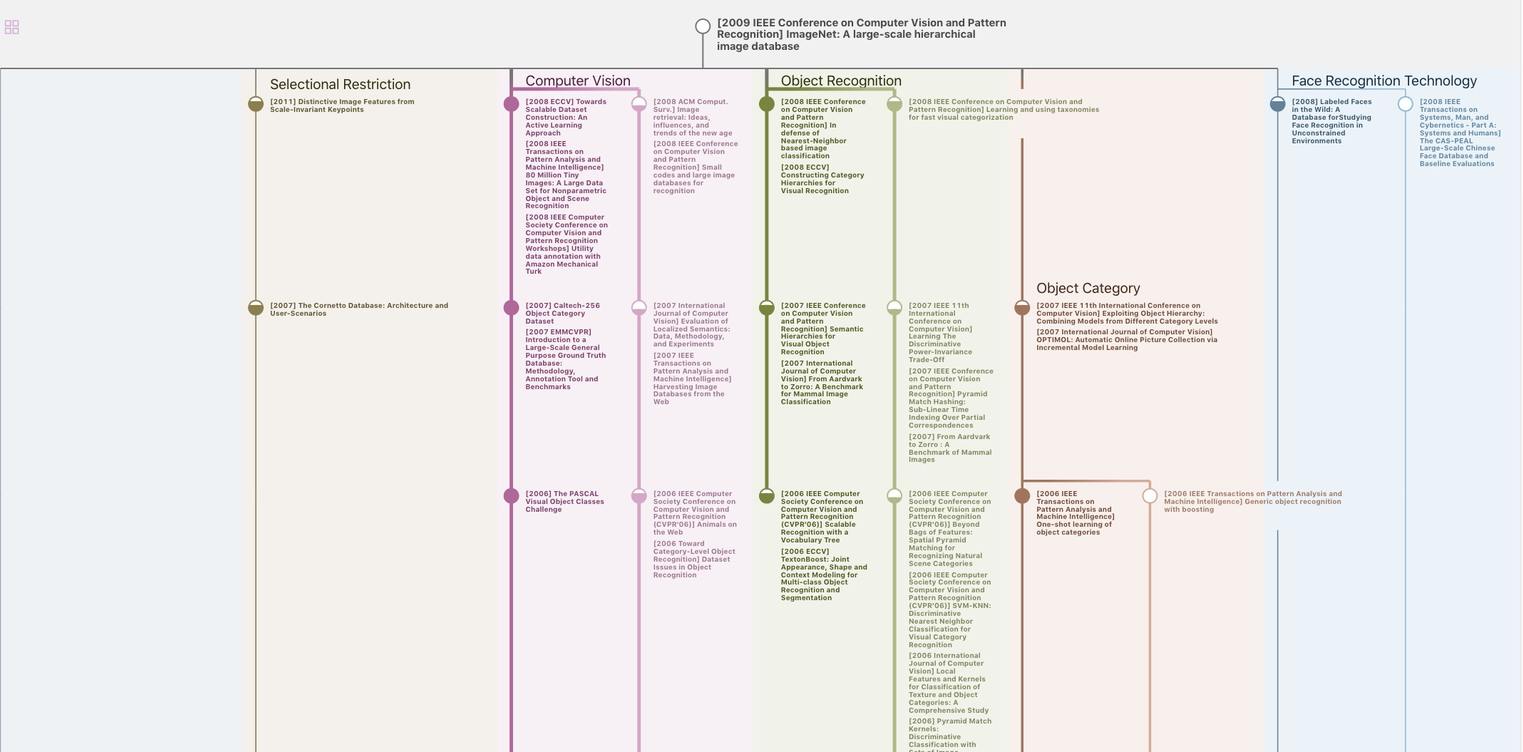
生成溯源树,研究论文发展脉络
Chat Paper
正在生成论文摘要