An Efficient Similarity Measure for Collaborative Filtering.
Procedia Computer Science(2019)
摘要
In the field of recommendation system, the memory-based Collaborative filtering has been proven to be useful in lots of practices. Similarity measures like Pearson correlation coefficient tend to only focus on improving as much as possible the accuracy. Handling datasets with different features, exiting measures cannot apply to different types of data simultaneously. In this paper, an improved similarity measure Common Pearson Correlation Coefficient (COPC) was proposed. Unlike existing measures, it strongly depends on chosen distance function, which adhere to the natural property of monotonicity and utilize consensus evaluation measure to capture an optimal value to improve PCC measure. To mitigate sparse problem, we also introduce the Hellinger Distance (Hg) as global similarity to lower the impact of lacking co-rated items. Experimental results on real-world datasets demonstrates that our measure outperformed the existing schemes of predicting ratings.
更多查看译文
关键词
recommendation system,User-based Collaborative filtering,similarity measure,versatility problem,sparse data
AI 理解论文
溯源树
样例
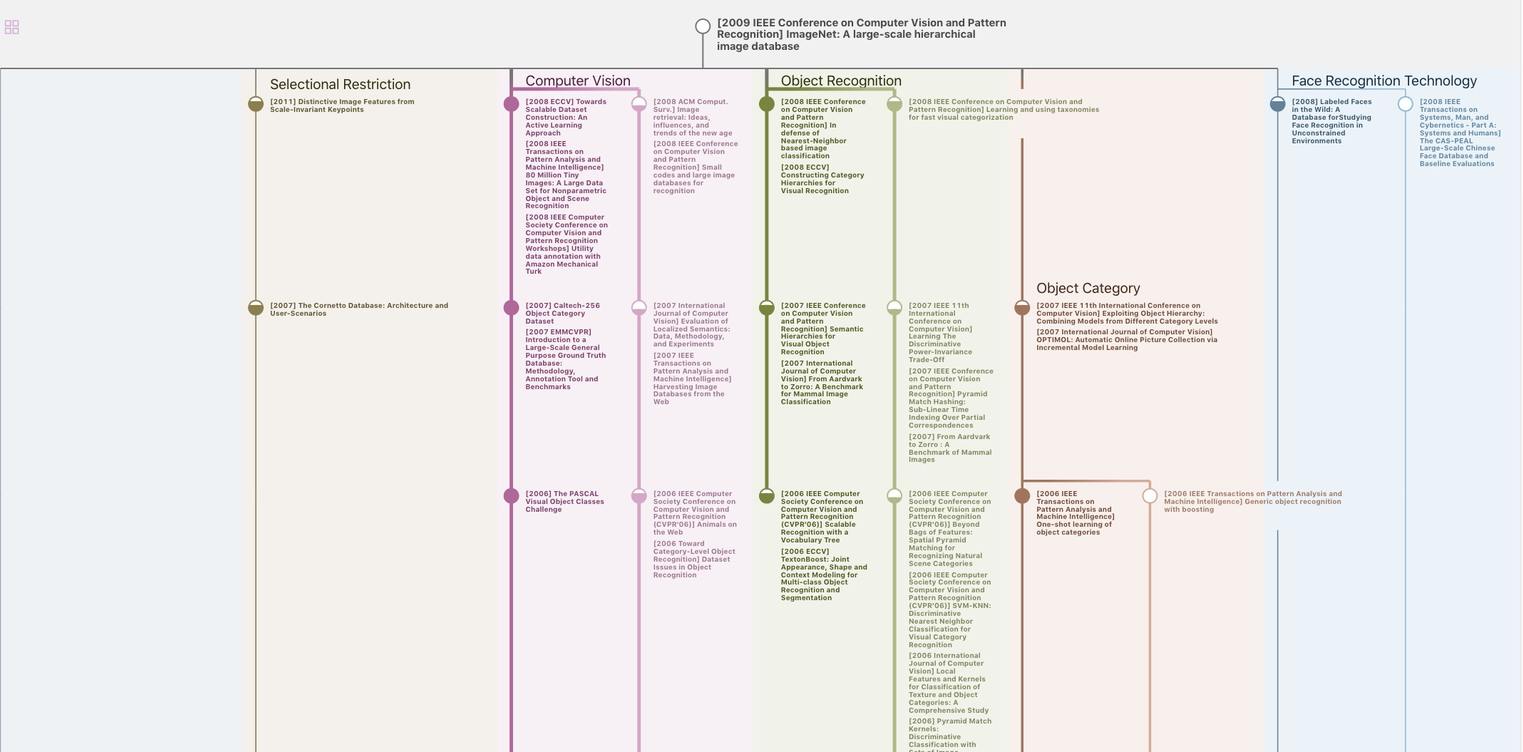
生成溯源树,研究论文发展脉络
Chat Paper
正在生成论文摘要