Structural sparsity of complex networks: Bounded expansion in random models and real-world graphs
Journal of Computer and System Sciences(2019)
摘要
This research establishes that many real-world networks exhibit bounded expansion2, a strong notion of structural sparsity, and demonstrates that it can be leveraged to design efficient algorithms for network analysis. Specifically, we give a new linear-time fpt algorithm for motif counting and linear time algorithms to compute localized variants of several centrality measures. To establish structural sparsity in real-world networks, we analyze several common network models regarding their structural sparsity. We show that, with high probability, (1) graphs sampled with a prescribed sparse degree sequence; (2) perturbed bounded-degree graphs; (3) stochastic block models with small probabilities; result in graphs of bounded expansion. In contrast, we show that the Kleinberg and the Barabási–Albert model have unbounded expansion. We support our findings with empirical measurements on a corpus of real-world networks.
更多查看译文
关键词
Structural sparsity,Bounded expansion,Complex networks,Random graphs,Motif counting,Centrality measures
AI 理解论文
溯源树
样例
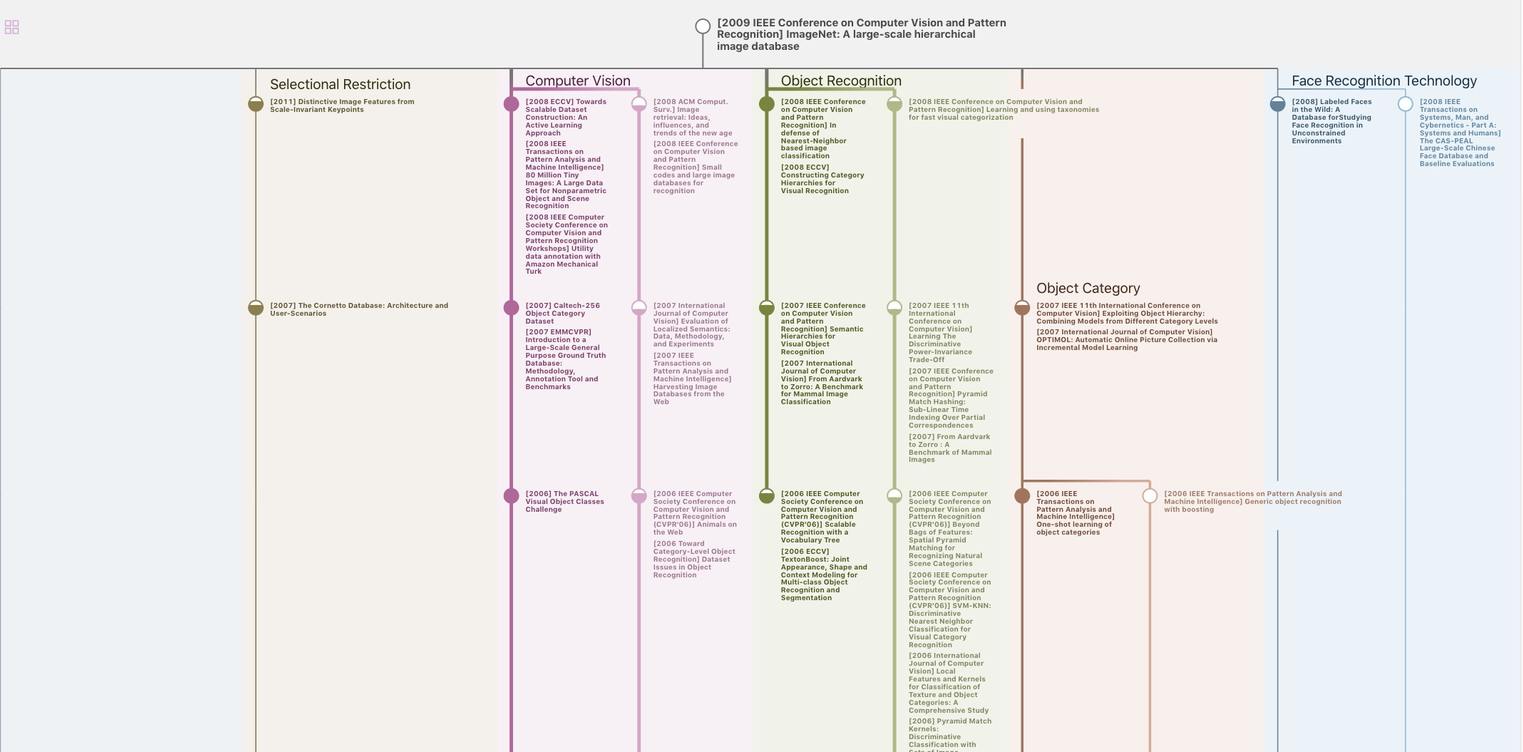
生成溯源树,研究论文发展脉络
Chat Paper
正在生成论文摘要