Beyond News Contents: The Role of Social Context for Fake News Detection
WSDM(2017)
摘要
Social media is becoming popular for news consumption due to its fast dissemination, easy access, and low cost. However, it also enables the wide propagation of fake news, i.e., news with intentionally false information. Detecting fake news is an important task, which not only ensures users to receive authentic information but also help maintain a trustworthy news ecosystem. The majority of existing detection algorithms focus on finding clues from news contents, which are generally not effective because fake news is often intentionally written to mislead users by mimicking true news. Therefore, we need to explore auxiliary information to improve detection. The social context during news dissemination process on social media forms the inherent tri-relationship, the relationship among publishers, news pieces, and users, which has potential to improve fake news detection. For example, partisan-biased publishers are more likely to publish fake news, and low-credible users are more likely to share fake news. In this paper, we study the novel problem of exploiting social context for fake news detection. We propose a tri-relationship embedding framework TriFN, which models publisher-news relations and user-news interactions simultaneously for fake news classification. We conduct experiments on two real-world datasets, which demonstrate that the proposed approach significantly outperforms other baseline methods for fake news detection.
更多查看译文
关键词
fake news detection, joint learning, social media mining
AI 理解论文
溯源树
样例
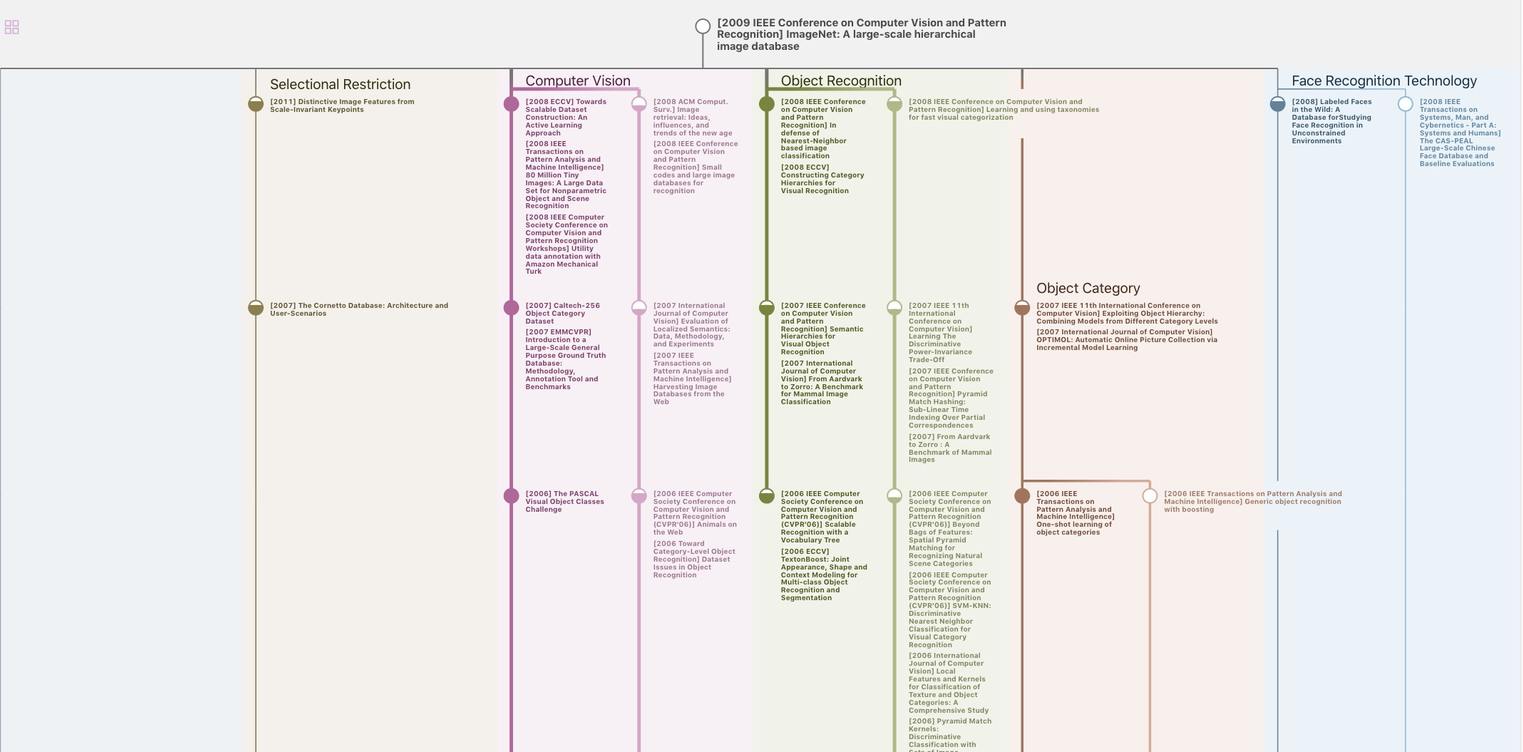
生成溯源树,研究论文发展脉络
Chat Paper
正在生成论文摘要