GAN-Based Super Resolution for Accurate 3D Surface Reconstruction from Light Field Skin Images Towards Haptic Palpation
2018 17th IEEE International Conference on Machine Learning and Applications (ICMLA)(2018)
摘要
The development of vision technology for observation of skin surface and diagnosis of skin disease for preventing secondary infections caused by direct skin touch has consistently been in the medical field spotlight. Many studies have been conducted to acquire three dimensional (3D) data through stereo images, multiple images, and lasers because (3D) data of in-vivo skin image is essential for accurate medical diagnosis. However, stereo vision systems or 3D laser systems for obtaining 3D information require high cost and have high computational complexity, and hence they have not been used universally. Additionally, the use of such systems is still not preferred in the medical field due to limitations on visual decision making. Therefore, a haptic diagnosis system that can blend vision information from a camera and palpation information from a dermatologist has been considered. In this study, we propose a 3D skin surface reconstruction method using a light field camera for haptic rendering and palpation. To achieve this goal, we addressed the low resolution problem, which has been consistently present in light field cameras, through the generative adversarial nets (GANs)-based super resolution method, and exploited the light field system which has been applied only to the object scene for obtaining 3D skin surface texture. Experimental results show that the method proposed in this study is promising and offers sufficient potential for haptic diagnosis.
更多查看译文
关键词
Light field images, deep learning, super resolution, haptic palpation, disparity map, 3D skin surface reconstruction
AI 理解论文
溯源树
样例
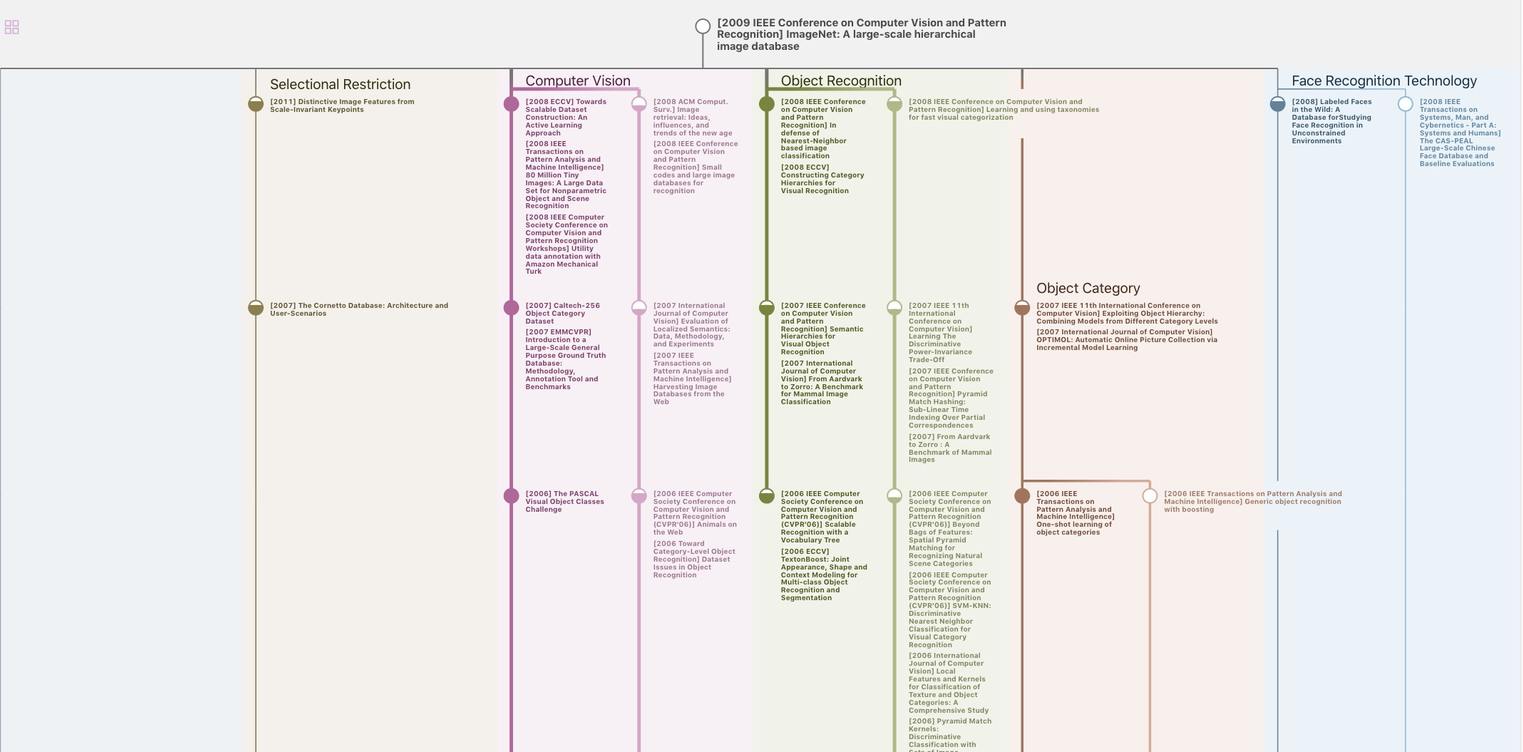
生成溯源树,研究论文发展脉络
Chat Paper
正在生成论文摘要