Detecting Compromised Implicit Association Test Results Using Supervised Learning
2018 17th IEEE International Conference on Machine Learning and Applications (ICMLA)(2019)
摘要
An implicit association test is a human psychological test used to measure subconscious associations. While widely recognized by psychologists as an effective tool in measuring attitudes and biases, the validity of the results can be compromised if a subject does not follow the instructions or attempts to manipulate the outcome. Compared to previous work, we collect training data using a more generalized methodology. We train a variety of different classifiers to identify a participant's first attempt versus a second possibly compromised attempt. To compromise the second attempt, participants are shown their score and are instructed to change it using one of five randomly selected deception methods. Compared to previous work, our methodology demonstrates a more robust and practical framework for accurately identifying a wide variety of deception techniques applicable to the IAT.
更多查看译文
关键词
implicit association test,supervised learning,machine learning,psychology application
AI 理解论文
溯源树
样例
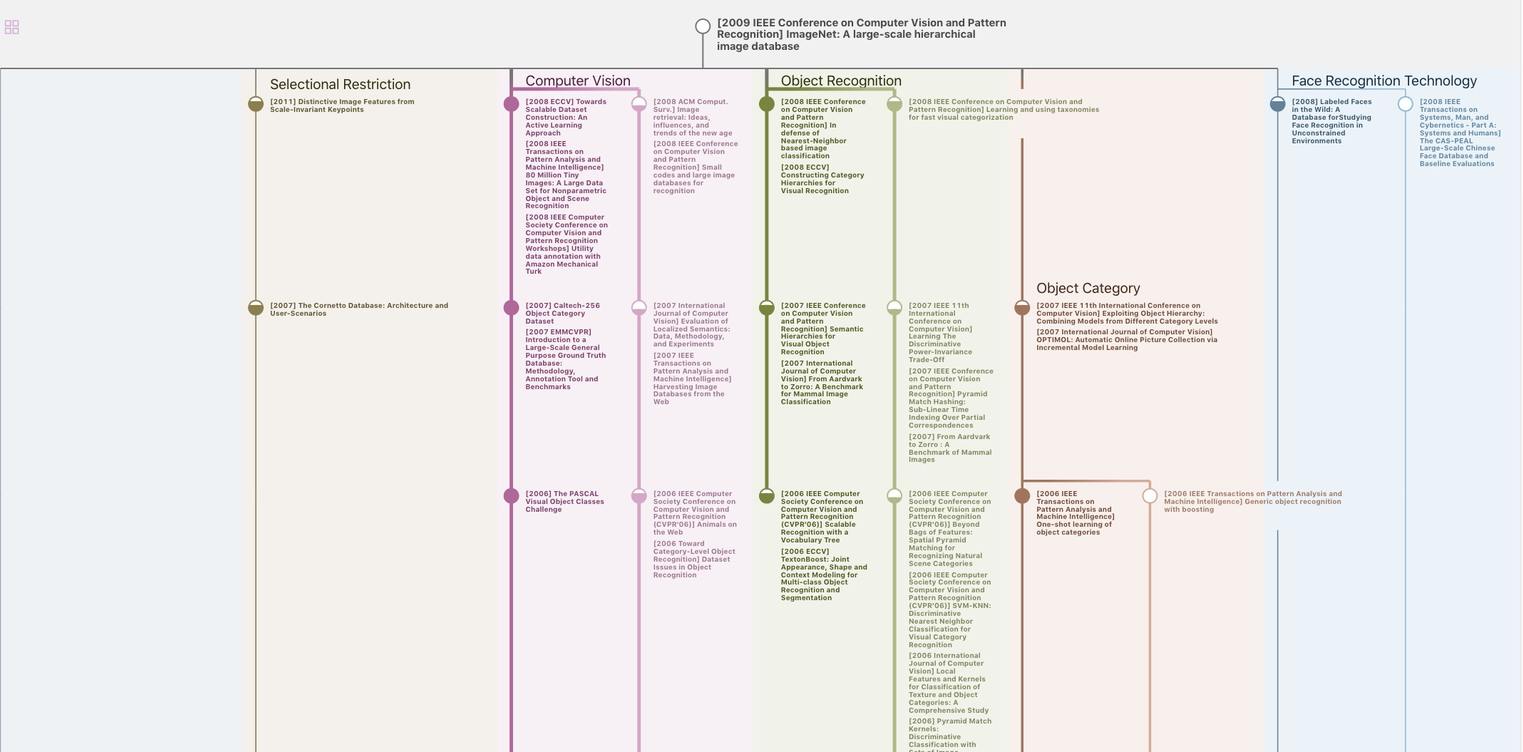
生成溯源树,研究论文发展脉络
Chat Paper
正在生成论文摘要