Beyond Inferring Class Representatives: User-Level Privacy Leakage From Federated Learning
arXiv (Cornell University)(2018)
摘要
Federated learning, i.e., a mobile edge computing framework for deep learning, is a recent advance in privacy-preserving machine learning, where the model is trained in a decentralized manner by the clients, i.e., data curators, preventing the server from directly accessing those private data from the clients. This learning mechanism significantly challenges the attack from the server side. Although the state-of-the-art attacking techniques that incorporated the advance of Generative adversarial networks (GANs) could construct class representatives of the global data distribution among all clients, it is still challenging to distinguishably attack a specific client (i.e., user-level privacy leakage), which is a stronger privacy threat to precisely recover the private data from a specific client. This paper gives the first attempt to explore user-level privacy leakage against the federated learning by the attack from a malicious server. We propose a framework incorporating GAN with a multi-task discriminator, which simultaneously discriminates category, reality, and client identity of input samples. The novel discrimination on client identity enables the generator to recover user specified private data. Unlike existing works that tend to interfere the training process of the federated learning, the proposed method works "invisibly" on the server side. The experimental results demonstrate the effectiveness of the proposed attacking approach and the superior to the state-of-the-art.
更多查看译文
关键词
Servers,Training,Data models,Privacy,Computational modeling,Gallium nitride,Data privacy
AI 理解论文
溯源树
样例
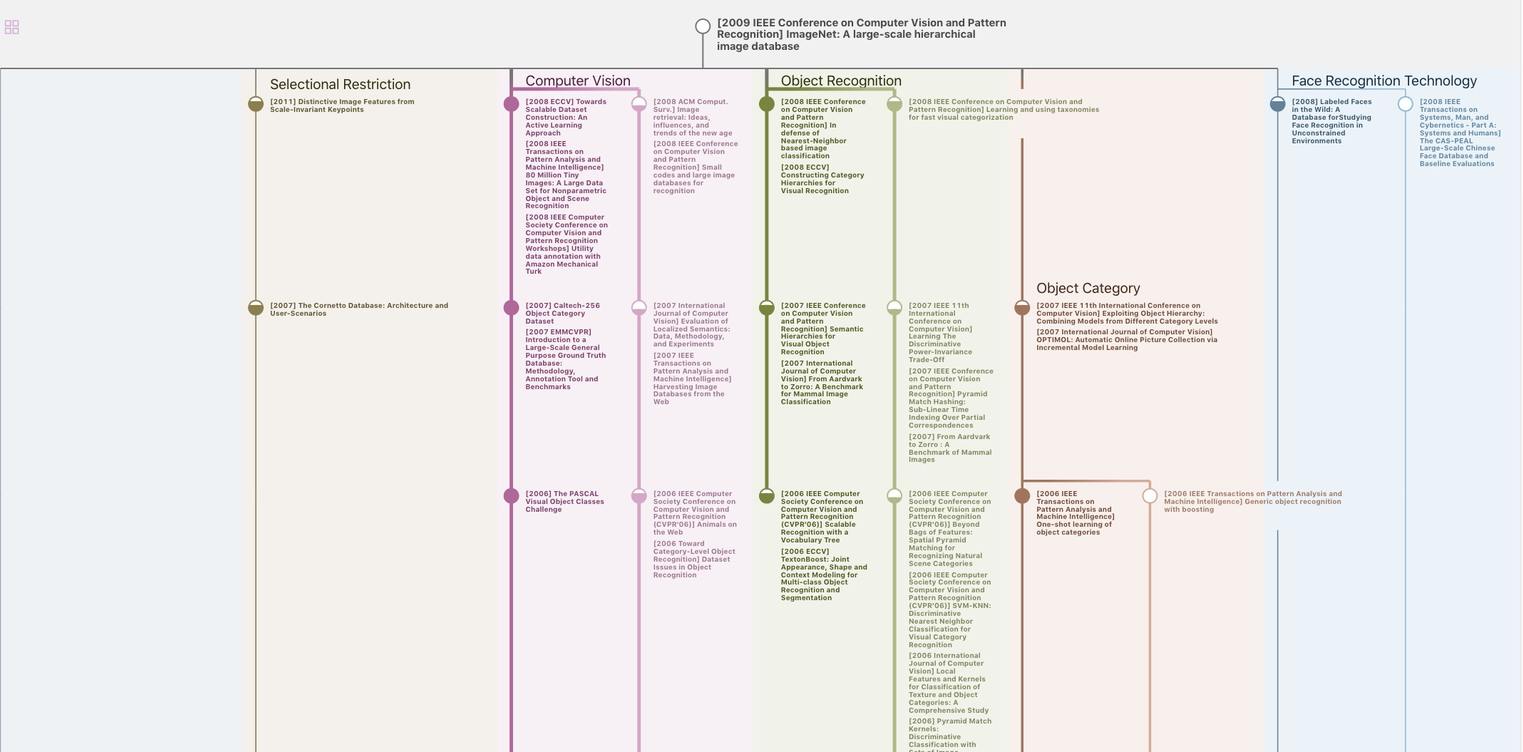
生成溯源树,研究论文发展脉络
Chat Paper
正在生成论文摘要