Graph Powering And Spectral Robustness
SIAM JOURNAL ON MATHEMATICS OF DATA SCIENCE(2020)
摘要
Spectral algorithms, such as principal component analysis and spectral clustering, rely on the extremal eigenpairs of a matrix A. However, these may be uninformative without preprocessing A with a proper transformation. The reason is that the spectrum of A may be contaminated by top eigenvalues resulting from scale variations in the data, such as high-degree nodes. Designing a good psi and establishing what good means is often challenging and model dependent. This paper proposes a simple and generic construction for sparse graphs, psi(A) = 1((I + A)(r) >= 1), where A denotes the adjacency matrix, r is an integer, and the indicator function is applied entrywise. We support this "graph powering" construction with the following regularization properties: (i) if the graph is drawn from the sparse Erdos-Renyi ensemble, which has no spectral gap, then graph powering produces a "maximal" spectral gap, comparable to that obtained when powering a random regular graph; (ii) if the graph is drawn from the sparse stochastic block model, graph powering achieves the fundamental limit for weak recovery (the Kesten-Stigum threshold), settling at the same time a related conjecture by Massoulie in 2013; (iii) we also demonstrate that graph powering is significantly more robust to tangles and cliques than previous spectral algorithms based on self-avoiding or nonbacktracking walk counts, using a geometric block model as our benchmark and introducing new conjectures for this model.
更多查看译文
关键词
community detection, stochastic block models, random graphs, spectral algorithms, network data analysis, spectral embeddings
AI 理解论文
溯源树
样例
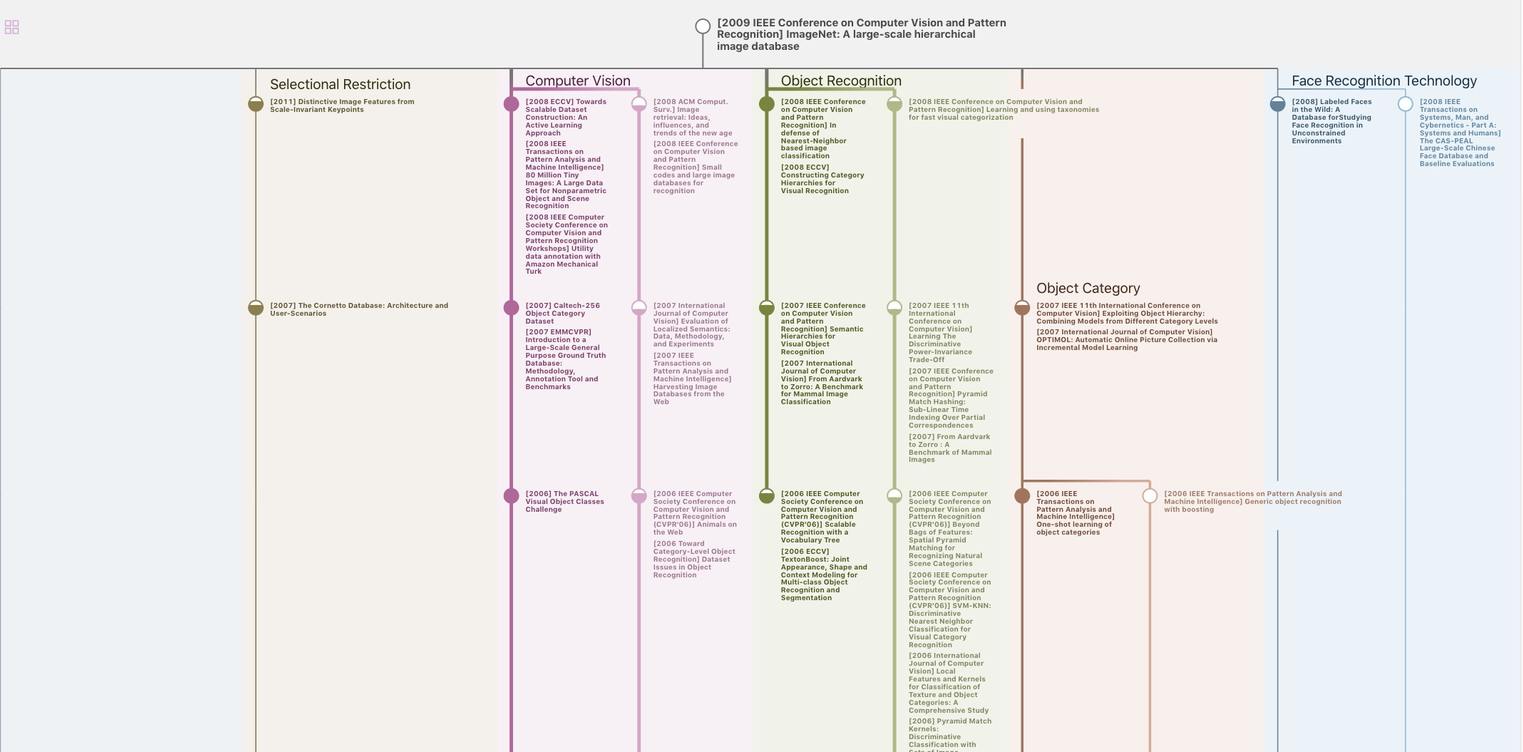
生成溯源树,研究论文发展脉络
Chat Paper
正在生成论文摘要