Guaranteed Globally Optimal Planar Pose Graph and Landmark SLAM via Sparse-Bounded Sums-of-Squares Programming
2019 International Conference on Robotics and Automation (ICRA)(2022)
摘要
Autonomous navigation requires an accurate model or map of the environment. While dramatic progress in the prior two decades has enabled large-scale SLAM, the majority of existing methods rely on non-linear optimization techniques to find the MLE of the robot trajectory and surrounding environment. These methods are prone to local minima and are thus sensitive to initialization. Several recent papers have developed optimization algorithms for the Pose-Graph SLAM problem that can certify the optimality of a computed solution. Though this does not guarantee a priori that this approach generates an optimal solution, a recent extension has shown that when the noise lies within a critical threshold that the solution to the optimization algorithm is guaranteed to be optimal. To address the limitations of existing approaches, this paper illustrates that the Pose-Graph SLAM and Landmark SLAM can be formulated as polynomial optimization programs that are SOS convex. This paper then describes how the Pose-Graph and Landmark SLAM problems can be solved to a global minimum without initialization regardless of noise level using the Sparse-BSOS hierarchy. This paper also empirically illustrates that convergence happens at the second step in this hierarchy. In addition, this paper illustrates how this Sparse-BSOS hierarchy can be implemented in the complex domain and empirically shows that convergence happens also at the second step of this complex domain hierarchy. Finally, the superior performance of the proposed approach when compared to existing SLAM methods is illustrated on graphs with several hundred nodes.
更多查看译文
关键词
autonomous navigation,nonlinear optimization techniques,maximum likelihood estimate,robot trajectory,polynomial optimization programs,planar pose graph,landmark SLAM,sparse-bounded sums-of-squares programming,simultaneous localization and mapping,pose-graph SLAM problem,sum-of-squares convex,SOS convex,sparse bounded degree sum-of-squares optimization method,sparse-BSOS optimization method
AI 理解论文
溯源树
样例
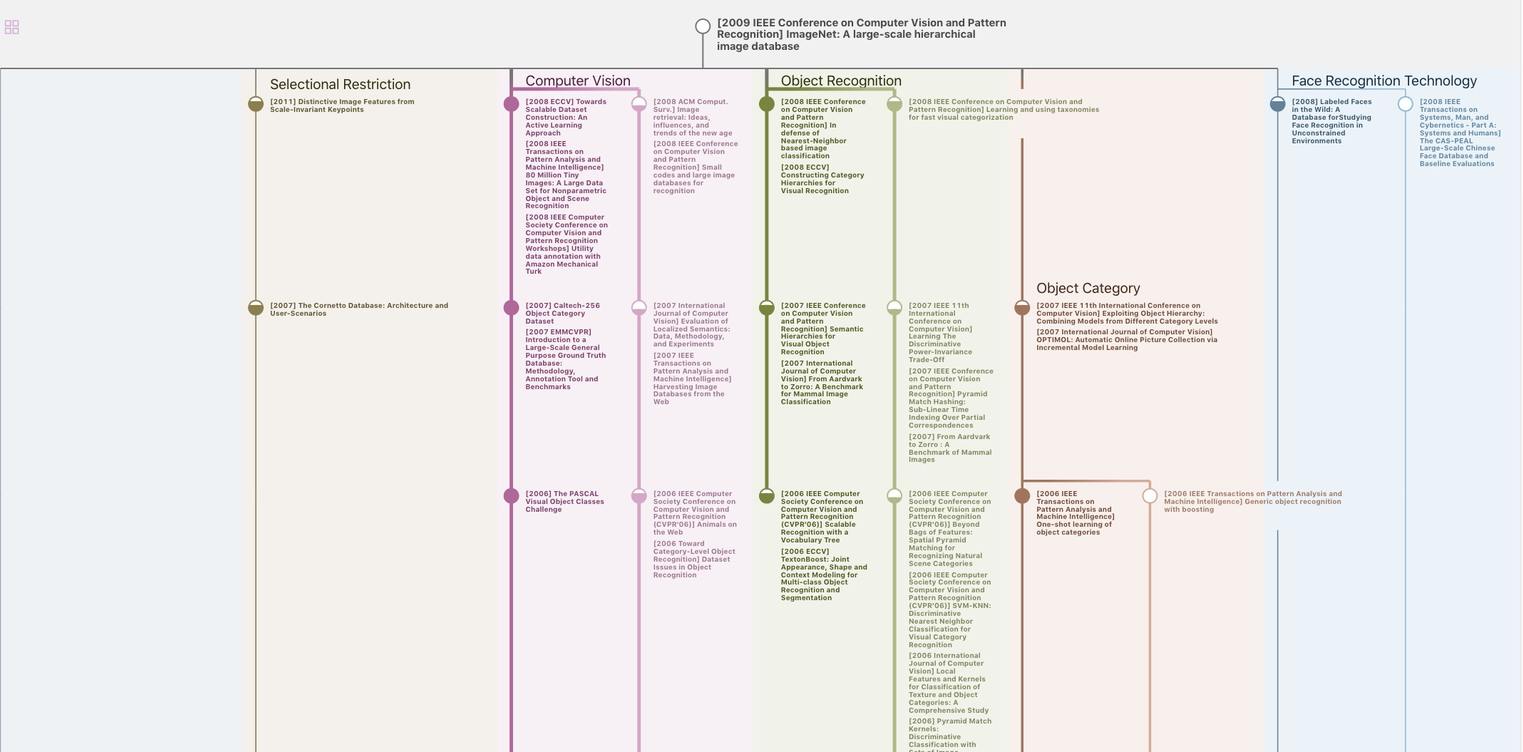
生成溯源树,研究论文发展脉络
Chat Paper
正在生成论文摘要