Challenges of convex quadratic bi-objective benchmark problems
Genetic and Evolutionary Computation Conference(2019)
摘要
ABSTRACTConvex quadratic objective functions are an important base case in state-of-the-art benchmark collections for single-objective optimization on continuous domains. Although often considered rather simple, they represent the highly relevant challenges of non-separability and ill-conditioning. In the multi-objective case, quadratic benchmark problems are under-represented. In this paper we analyze the specific challenges that can be posed by quadratic functions in the bi-objective case. Our construction yields a full factorial design of 54 different problem classes. We perform experiments with well-established algorithms to demonstrate the insights that can be supported by this function class. We find huge performance differences, which can be clearly attributed to two root causes: non-separability and alignment of the Pareto set with the coordinate system.
更多查看译文
关键词
Multi-Objective Optimization, Analysis, Benchmarks
AI 理解论文
溯源树
样例
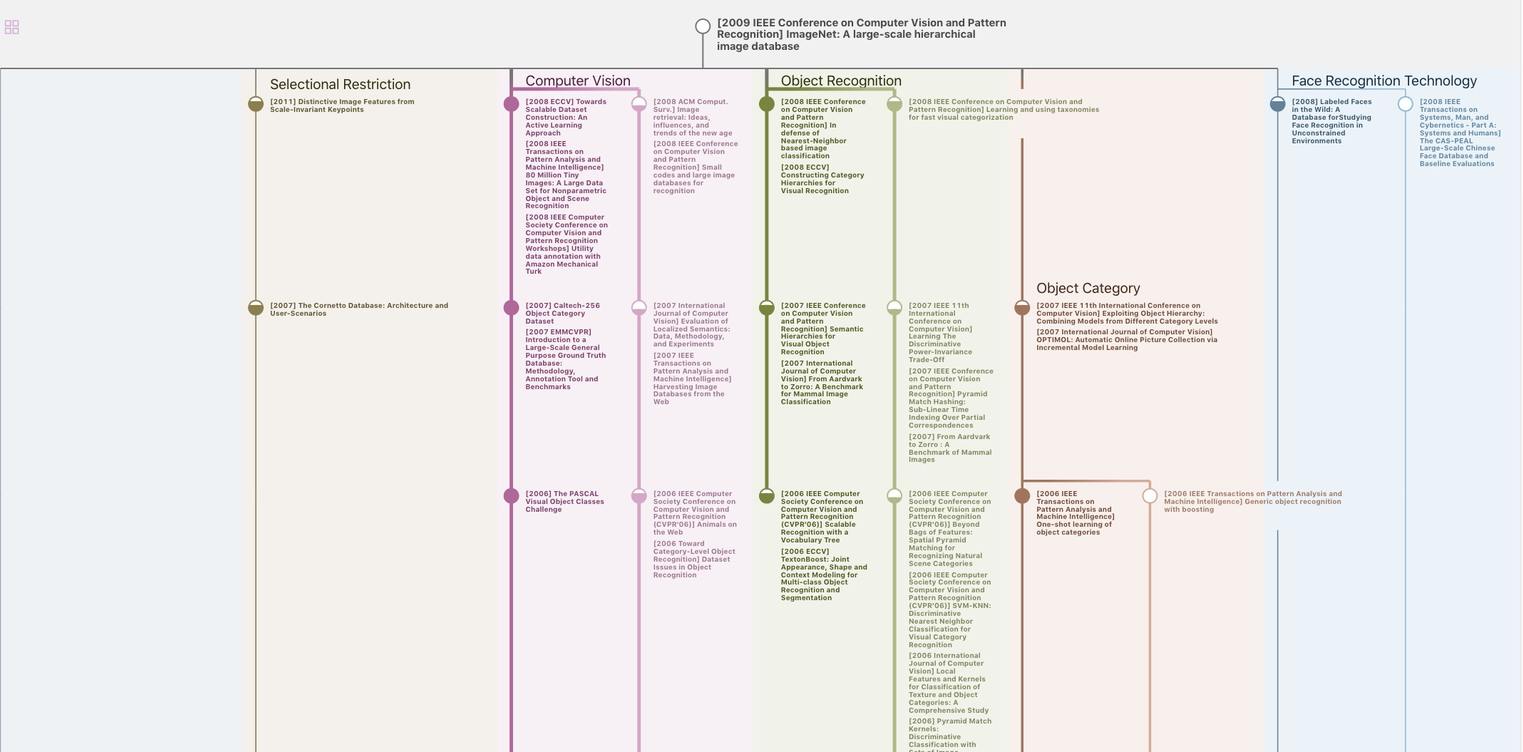
生成溯源树,研究论文发展脉络
Chat Paper
正在生成论文摘要