Wrapper-Based Fuzzy Inductive Reasoning Model Identification For Imbalance Data Classification
2018 IEEE INTERNATIONAL CONFERENCE ON FUZZY SYSTEMS (FUZZ-IEEE)(2018)
摘要
Fuzzy Inductive Reasoning (FIR) is a qualitative inductive modeling and simulation methodology for dealing with complex dynamical systems. FIR has proven to be a powerful tool for qualitative model identification and prediction of future behavior of different kinds of system domains including biology, medicine, ecology, etc. FIR has been mainly applied to regression problems, but recently we are interested in studying the feasibility of FIR as a classifier. The main objective of this study is to analyze and revise the model selection process in FIR methodology from the perspective of a classifier when dealing with imbalance data. In this research we propose a wrapper technique for fuzzy model identification in the context of FIR. We demonstrate that this new approach exhibits a significant improvement comparing to classical FIR model selection when applied to imbalanced data classification. In this paper we also compare FIR Classifier with wrapper model selection to similar genre of classic rule-based and instance-based classifiers, i.e. RISE, kNN, C4.5, CN2, PART, RIPPER and Modlem, when applied to a set of classification benchmarks.
更多查看译文
关键词
Fuzzy inductive reasoning, imbalance data, classification, wraper-based models
AI 理解论文
溯源树
样例
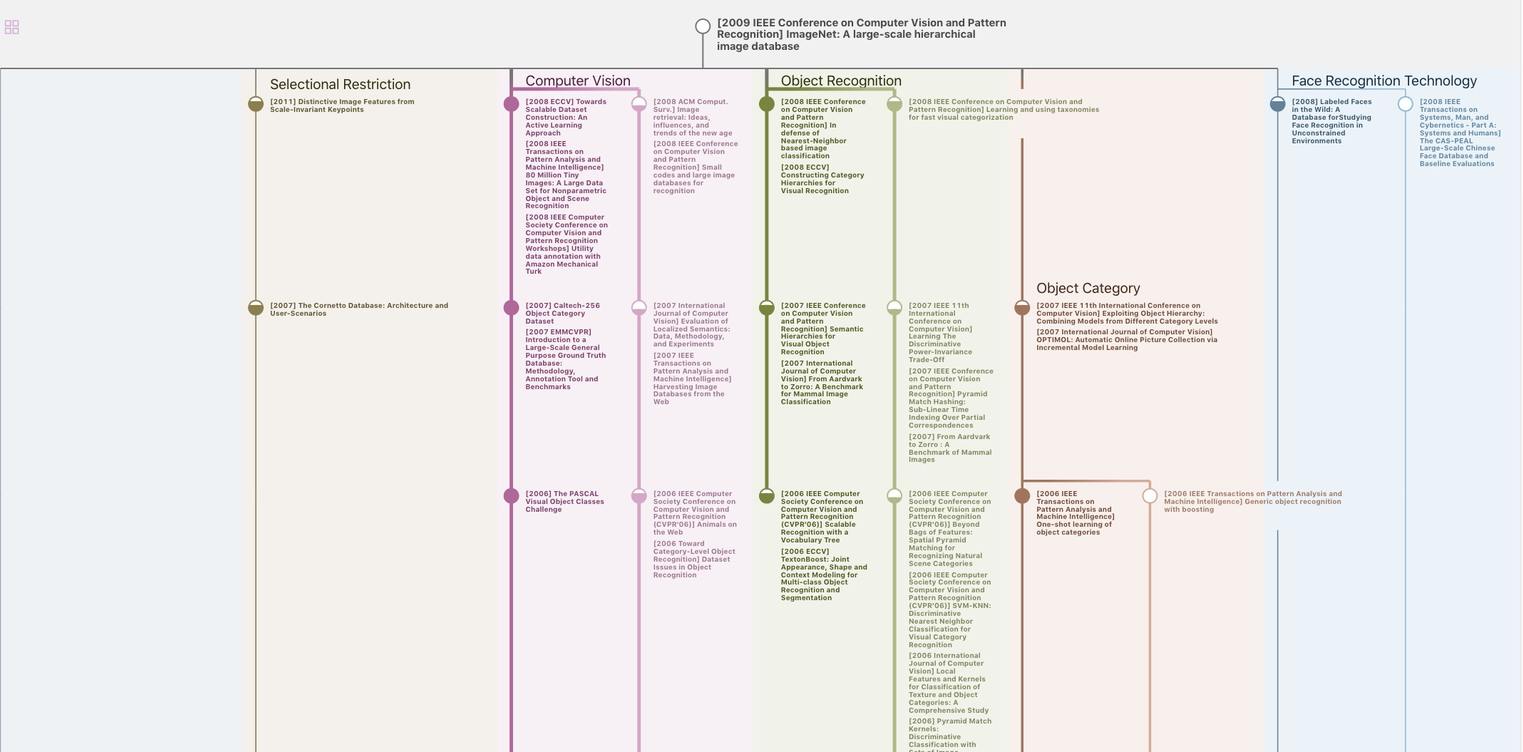
生成溯源树,研究论文发展脉络
Chat Paper
正在生成论文摘要