Walk and learn: Enabling accurate indoor positioning by profiling outdoor movement on smartphones.
Pervasive and Mobile Computing(2018)
摘要
One of the key objectives in developing pervasive and mobile applications is to localize users within indoor environments. By employing accelerometers on smartphones, dead reckoning is an intuitive and common approach to generate a user’s indoor motion trace via mobile devices. Nevertheless, dead reckoning often deviates from the ground truth due to noise in the sensing data. We propose IOLoc, an indoor localization approach that benefits by transferring learning from tracking outdoor motions to the indoor environment. Via sensing data on a smartphone, IOLoc constructs two datasets: relatively accurate outdoor motions from GPS and less accurate indoor motions from accelerometers. Then, IOLoc leverages a Motion Range Space to improve a user’s acceleration and orientation values used for computing dead reckoning. After using a transfer learning algorithm to the two datasets, IOLoc boosts the Motion Range Box to achieve better indoor localization results. Additionally, IOLoc exploits indoor GPS exception cases, pedometer, and average speed estimation to further improve dead reckoning. Through case studies on 15 volunteers for the indoor and outdoor scenarios, we show IOLoc is a non-infrastructure, low-training complexity, and energy saving indoor positioning approach that achieved a localization accuracy of 0.26∼0.49 m in multiple scenarios.
更多查看译文
关键词
Indoor localization,Smartphone,Transfer learning
AI 理解论文
溯源树
样例
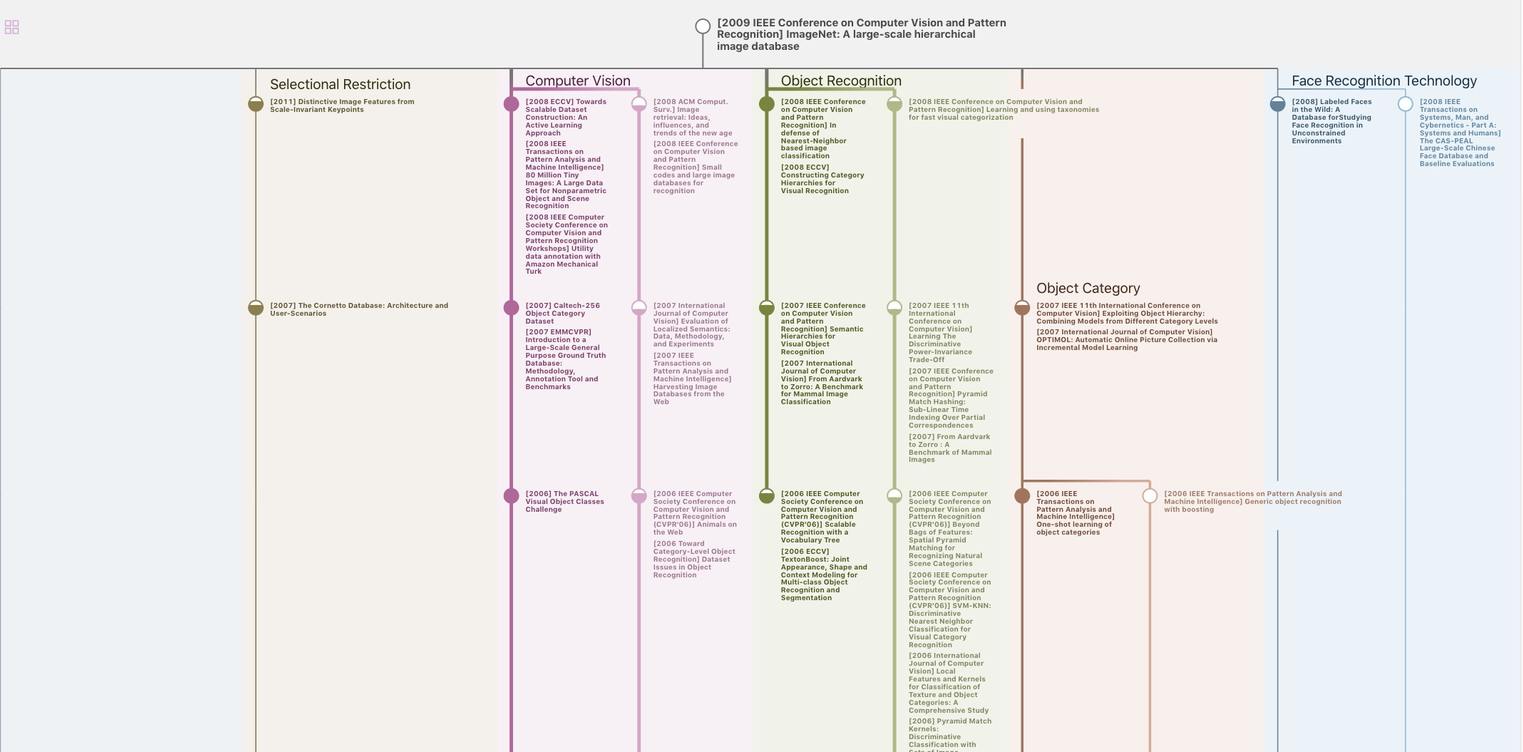
生成溯源树,研究论文发展脉络
Chat Paper
正在生成论文摘要