The right complexity measure in locally private estimation: it is not the fisher information
ANNALS OF STATISTICS(2024)
摘要
We identify fundamental tradeoffs between statistical utility and privacy under local models of privacy in which data is kept private even from the statistician, providing instance-specific bounds for private estimation and learning problems by developing the local minimax risk. In contrast to approaches based on worst-case (minimax) error, which are conservative, this allows us to evaluate the difficulty of individual problem instances and delineate the possibilities for adaptation in private estimation and inference. Our main results show that the local modulus of continuity of the estimand with respect to the variation distance-as opposed to the Hellinger distance central to classical statistics-characterizes rates of convergence under locally private estimation for many notions of privacy, including differential privacy and its relaxations. As consequences of these results, we identify an alternative to the Fisher information for private estimation, giving a more nuanced understanding of the challenges of adaptivity and optimality.
更多查看译文
关键词
Locally private estimation,local minimax complexity,L-1 information,strong data processing inequalities
AI 理解论文
溯源树
样例
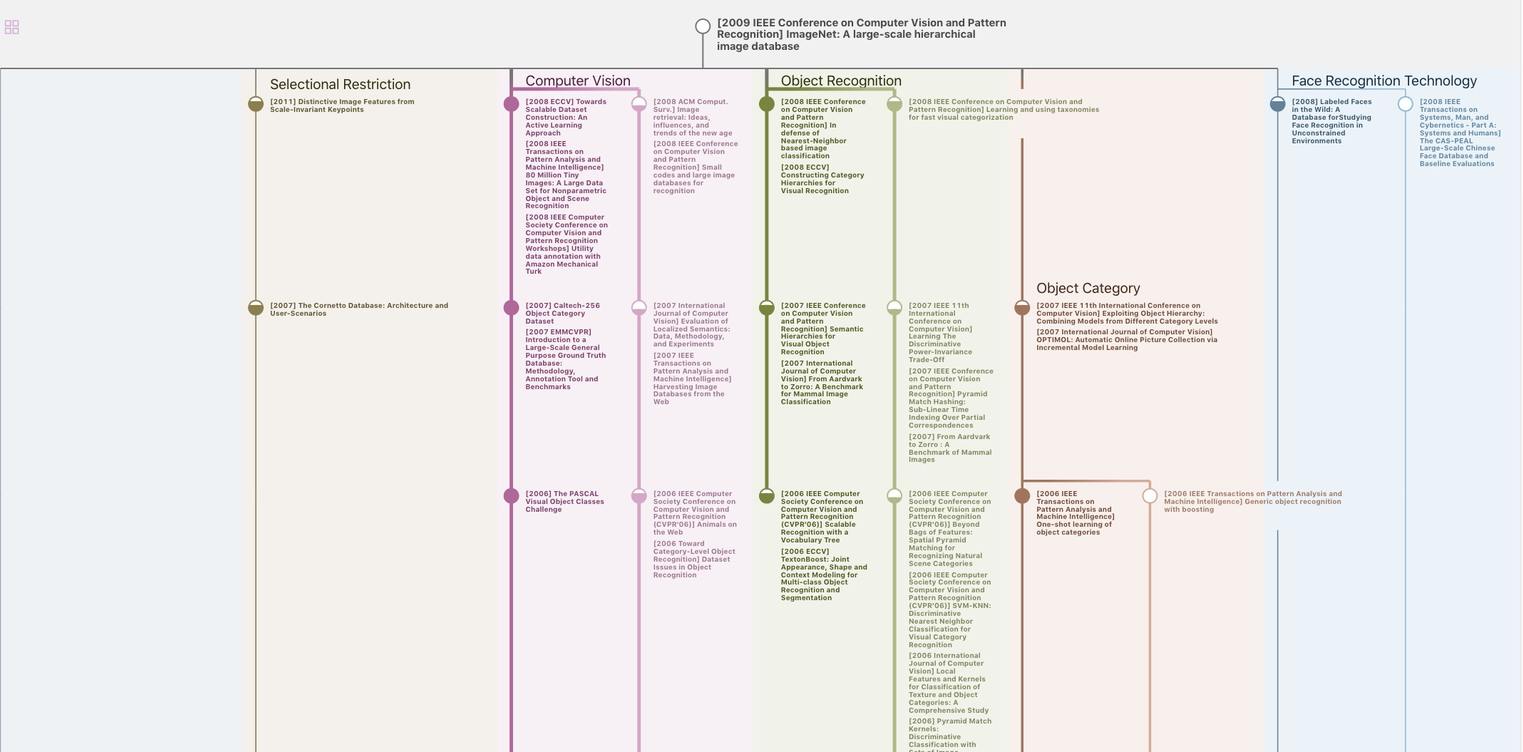
生成溯源树,研究论文发展脉络
Chat Paper
正在生成论文摘要