Studying the Potential of Multi-Target Classification on Patient Screening Data to Predict Dropout Cases
2018 IEEE 31st International Symposium on Computer-Based Medical Systems (CBMS)(2018)
摘要
Treatment of patients with tinnitus is mainly pursued on the basis of screening data, encompassing answers to questionnaires or audiological examinations. Since tinnitus affects the quality of life of patients and is associated with comorbidities like depression, the screening step involves the acquisition of extensive amounts of information, which may contribute to the design of a personalized treatment. Often times, it can be observed that patients give up their treatment before completion (i.e., they constitute “dropout” cases). In this study, we investigate how multi-target classification on the screening data can contribute to characterize patients that will drop out of the study. For our analysis, we base or considerations on the target variable “tinnitus loudness”, i.e. the subjectively perceived loudness of the phantom signal. Following this, we attempt to identify variables that explain the tinnitus loudness together with the likelihood of interrupting the treatment. To be more precise, we report on results from gathered data of 1419 tinnitus patients from the University Hospital of Regensburg.
更多查看译文
关键词
multi target classification,data mining,medical mining,tinnitus loudness,tinnitus dropout
AI 理解论文
溯源树
样例
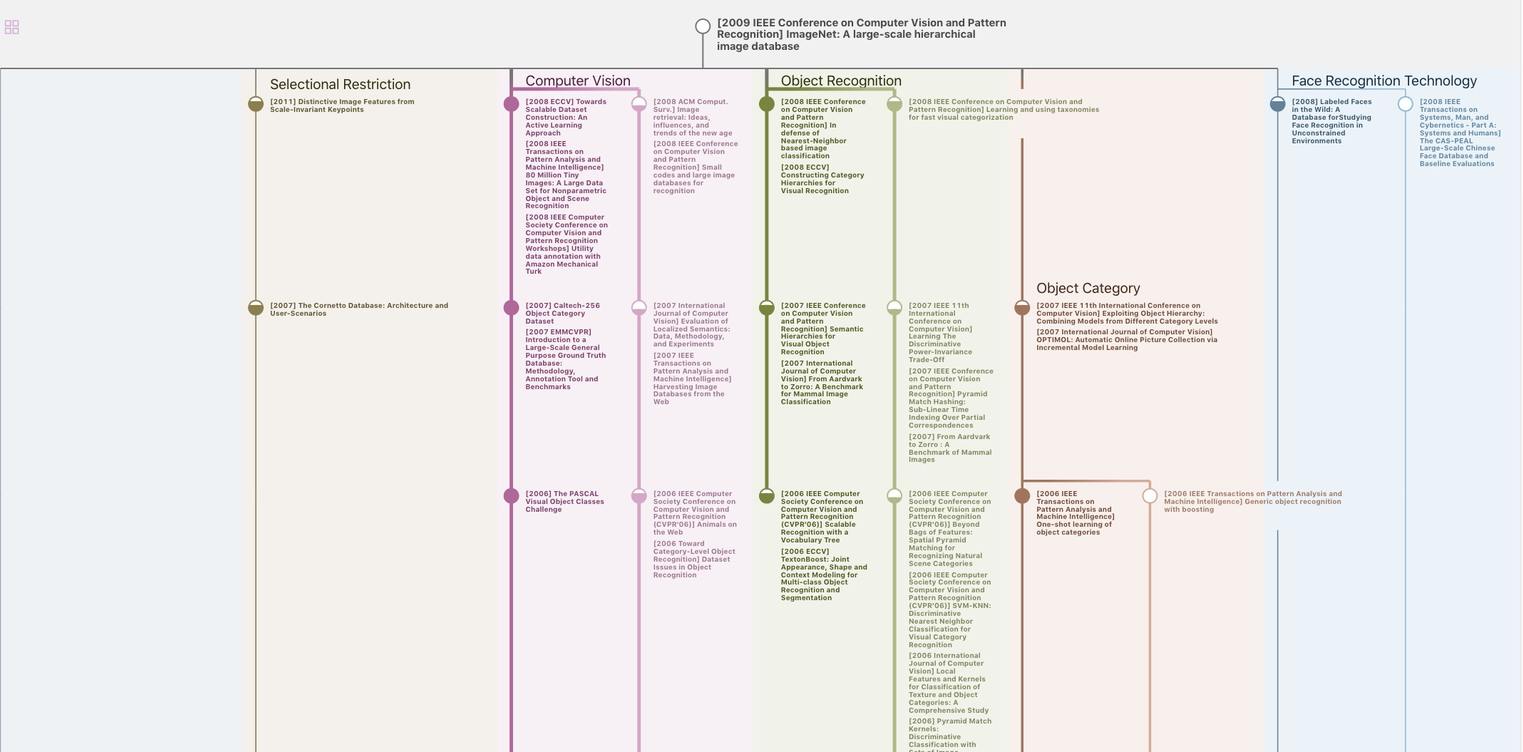
生成溯源树,研究论文发展脉络
Chat Paper
正在生成论文摘要