Sketching Linear Classifiers over Data Streams.
SIGMOD/PODS '18: International Conference on Management of Data Houston TX USA June, 2018(2018)
摘要
We introduce a new sub-linear space sketch---the Weight-Median Sketch---for learning compressed linear classifiers over data streams while supporting the efficient recovery of large-magnitude weights in the model. This enables memory-limited execution of several statistical analyses over streams, including online feature selection, streaming data explanation, relative deltoid detection, and streaming estimation of pointwise mutual information. Unlike related sketches that capture the most frequently-occurring features (or items) in a data stream, the Weight-Median Sketch captures the features that are most discriminative of one stream (or class) compared to another. The Weight-Median Sketch adopts the core data structure used in the Count-Sketch, but, instead of sketching counts, it captures sketched gradient updates to the model parameters. We provide a theoretical analysis that establishes recovery guarantees for batch and online learning, and demonstrate empirical improvements in memory-accuracy trade-offs over alternative memory-budgeted methods, including count-based sketches and feature hashing.
更多查看译文
关键词
linear classifiers,data streams
AI 理解论文
溯源树
样例
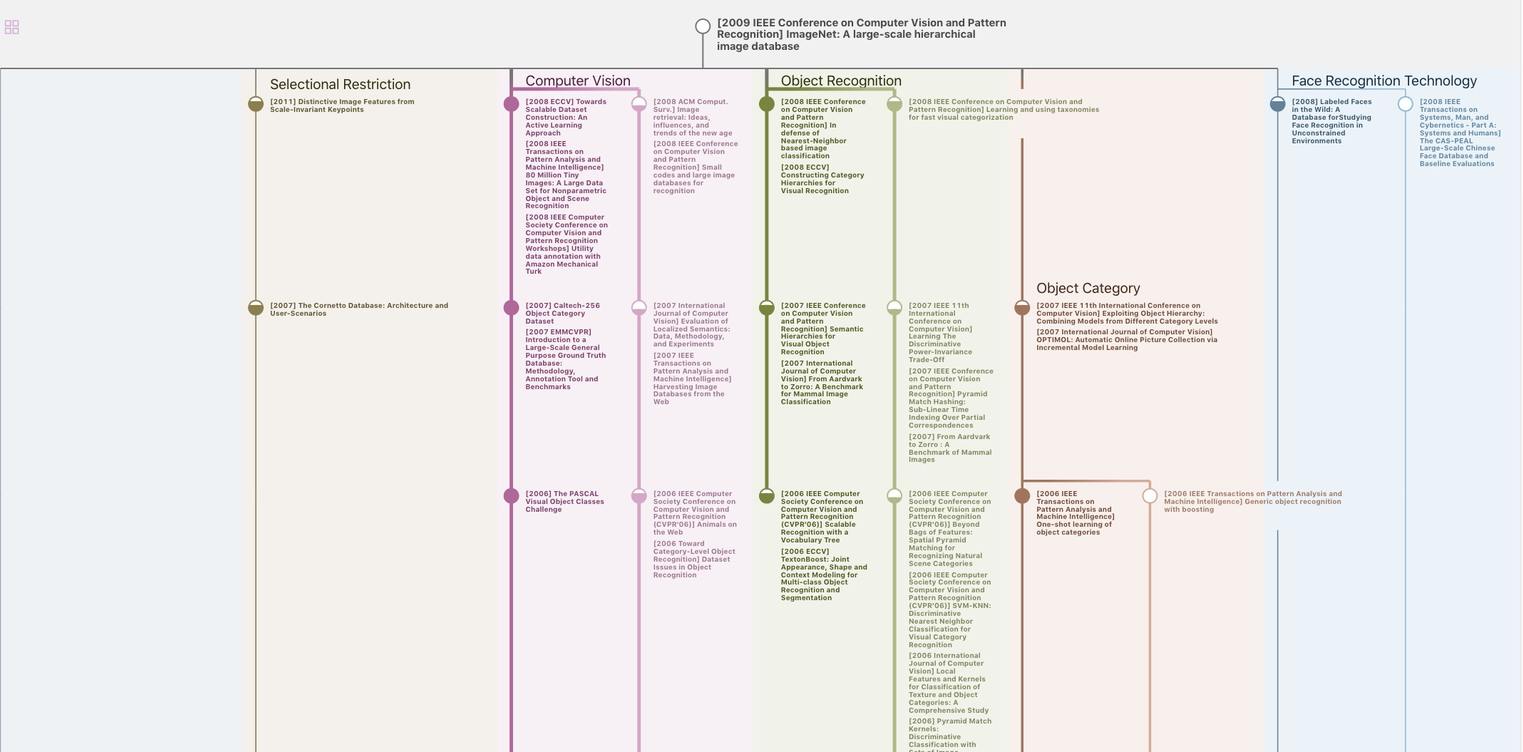
生成溯源树,研究论文发展脉络
Chat Paper
正在生成论文摘要