Online Learning Of A Weighted Selective Naive Bayes Classifier With Non-Convex Optimization
ADVANCES IN KNOWLEDGE DISCOVERY AND MANAGEMENT, VOL 6(2017)
摘要
We study supervised classification for data streams with a high number of input variables. The basic naive Bayes classifier is attractive for its simplicity and performance when the strong assumption of conditional independence is valid. Variable selection and model averaging are two common ways to improve this model. This process leads to manipulate a weighted naive Bayes classifier. We focus here on direct estimation of weighted naive Bayes classifiers. We propose a sparse regularization of the model log-likelihood which takes into account knowledge relative to each input variable. The sparse regularized likelihood being non convex, we propose an online gradient algorithm using mini-batches and random perturbation according to a metaheuristic to avoid local minima. In our experiments, we first study the optimization quality, then the classifier performance under varying its parameterization. The results confirm the effectiveness of our approach.
更多查看译文
关键词
Supervised classification, Naive Bayes classifier, Non-convex optimization, Stochastic optimization, Variables selection, Sparse regularization
AI 理解论文
溯源树
样例
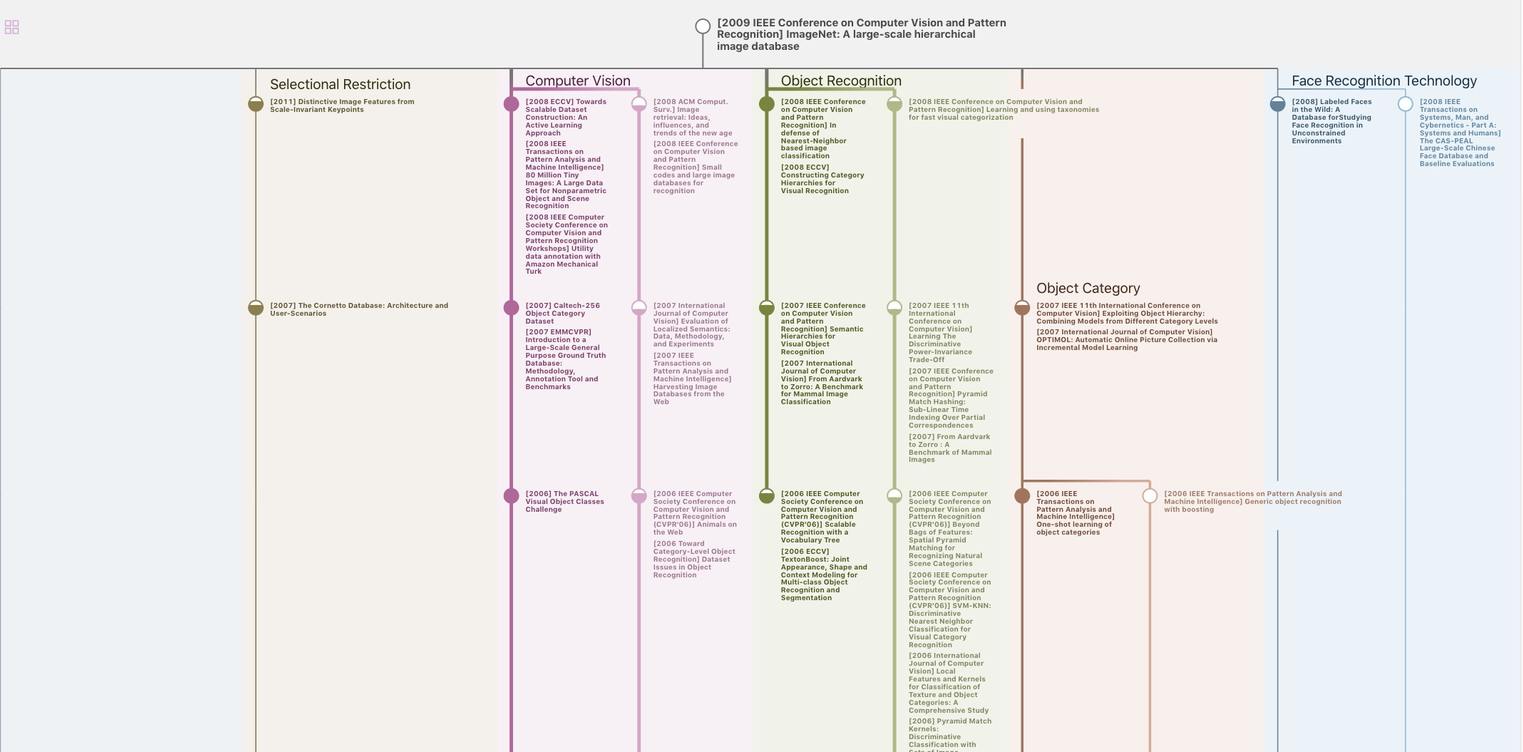
生成溯源树,研究论文发展脉络
Chat Paper
正在生成论文摘要