Sparkle: optimizing spark for large memory machines and analytics.
SoCC '17: ACM Symposium on Cloud Computing Santa Clara California September, 2017(2017)
摘要
Given the growing availability of affordable scale-up servers, our goal is to bring the performance benefits of in-memory processing on scale-up servers to an increasingly common class of data analytics applications that process small to medium size datasets (up to a few 100GBs) that can easily fit in the memory of a typical scale-up server To achieve this goal, we leverage Spark, an existing memory-centric data analytics framework with wide-spread adoption among data scientists. Bringing Spark's data analytic capabilities to a scale-up system requires rethinking the original design assumptions, which, although effective for a scale-out system, are a poor match to a scale-up system resulting in unnecessary communication and memory inefficiencies.
更多查看译文
AI 理解论文
溯源树
样例
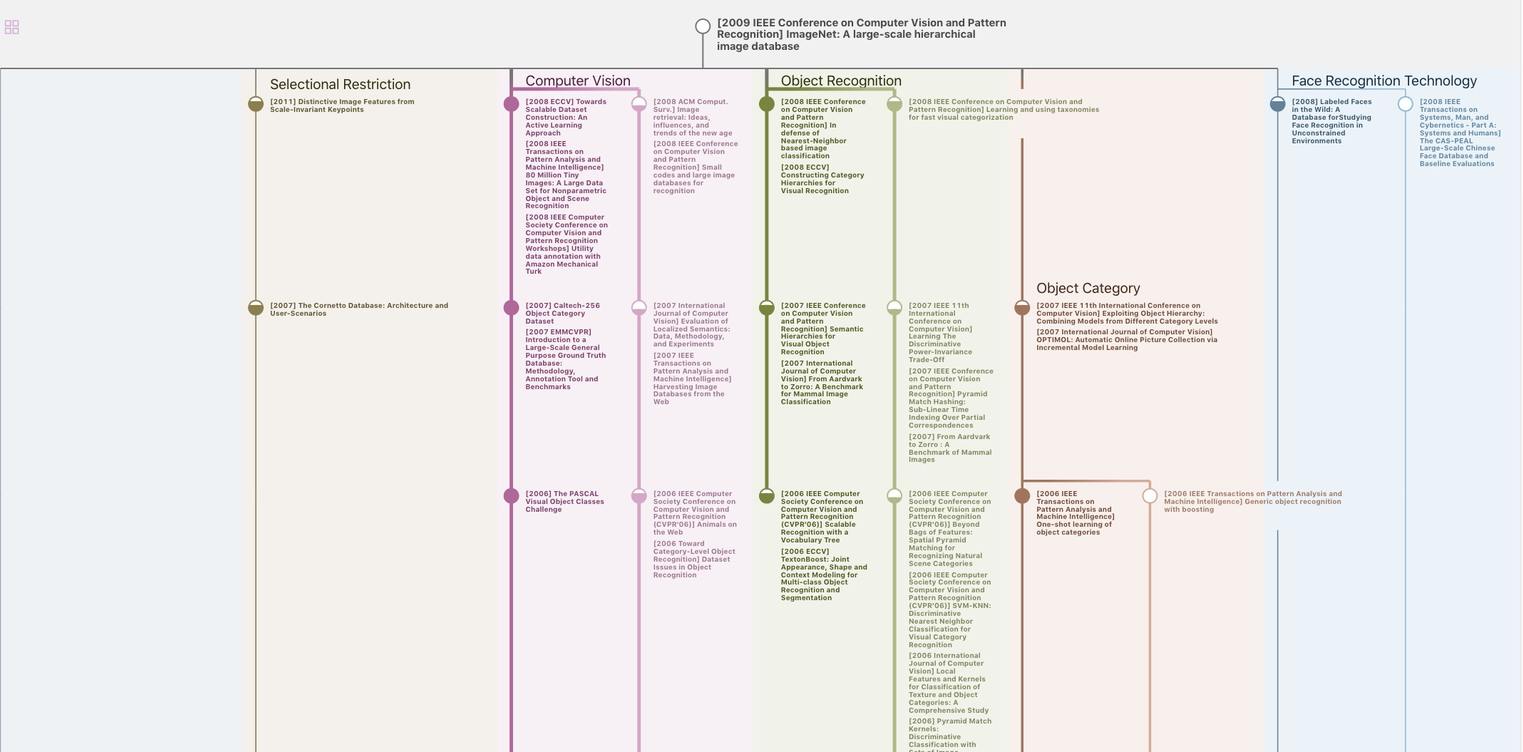
生成溯源树,研究论文发展脉络
Chat Paper
正在生成论文摘要